D-DAE: Defense-Penetrating Model Extraction Attacks.
SP(2023)
摘要
Recent studies show that machine learning models are vulnerable to model extraction attacks, where the adversary builds a substitute model that achieves almost the same performance of a black-box victim model simply via querying the victim model. To defend against such attacks, a series of methods have been proposed to disrupt the query results before returning them to potential attackers, greatly degrading the performance of existing model extraction attacks. In this paper, we make the first attempt to develop a defensepenetrating model extraction attack framework, named D- DAE, which aims to break disruption-based defenses. The linchpins of D- DAE are the design of two modules, i.e., disruption detection and disruption recovery, which can be integrated with generic model extraction attacks. More specifically, after obtaining query results from the victim model, the disruption detection module infers the defense mechanism adopted by the defender. We design a meta-learning-based disruption detection algorithm for learning the fundamental differences between the distributions of disrupted and undisrupted query results. The algorithm features a good generalization property even if we have no access to the original training dataset of the victim model. Given the detected defense mechanism, the disruption recovery module tries to restore a clean query result from the disrupted query result with well-designed generative models. Our extensive evaluations on MNIST, FashionMNIST, CIFAR-10, GTSRB, and ImageNette datasets demonstrate that D- DAE can enhance the substitute model accuracy of the existing model extraction attacks by as much as 82.24% in the face of 4 state-of-the-art defenses and combinations of multiple defenses. We also verify the effectiveness of D-DAE in penetrating unknown defenses in real-world APIs hosted by Microsoft Azure and Face++.
更多查看译文
关键词
Model extraction attacks,disruption-based defenses,meta-learning
AI 理解论文
溯源树
样例
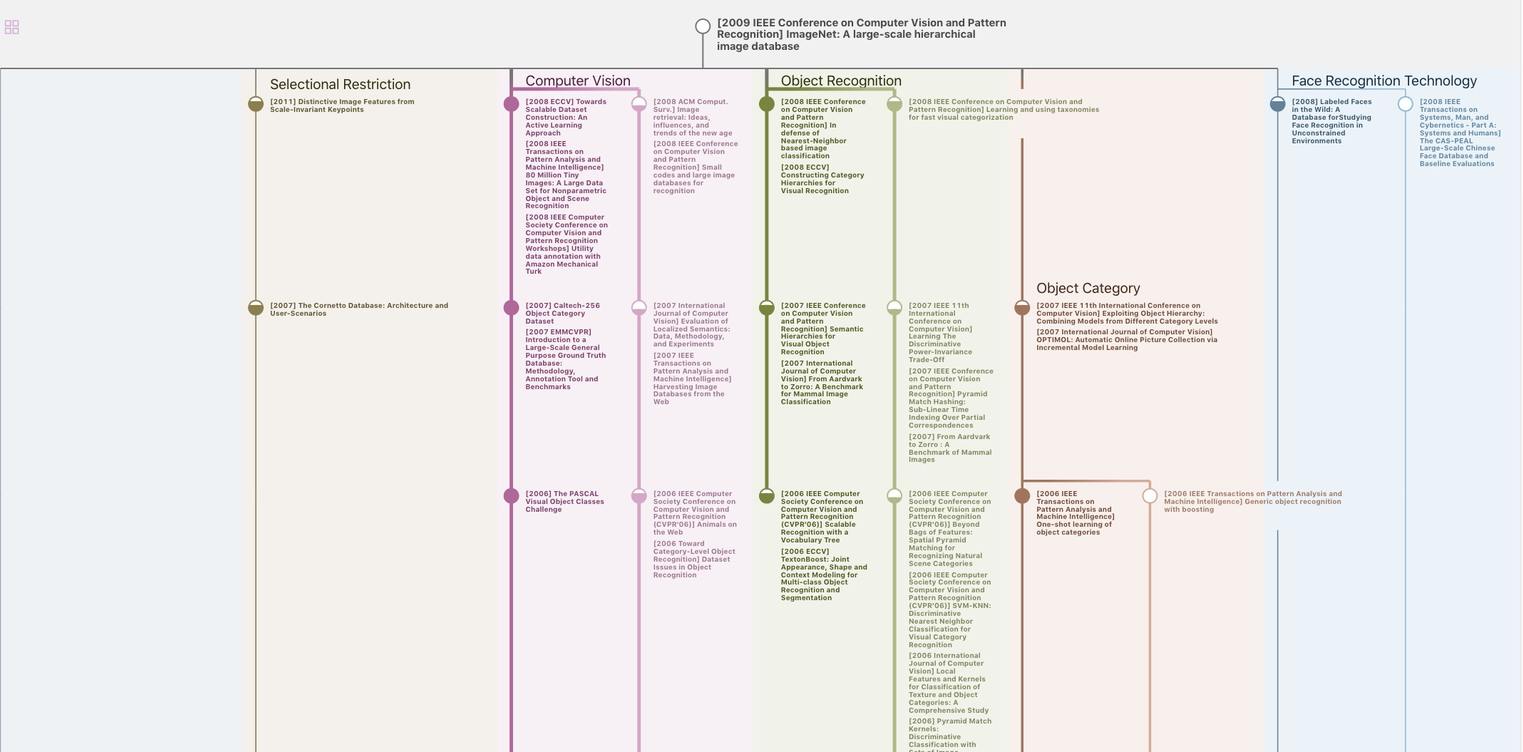
生成溯源树,研究论文发展脉络
Chat Paper
正在生成论文摘要