EXARL-PARS: Parallel Augmented Random Search Using Reinforcement Learning at Scale for Applications in Power Systems.
e-Energy (Companion)(2023)
摘要
With recent advances in deep learning and large-scale computing, learning-based controls have become increasingly attractive for complex physical systems. Consequently, developing generalized learning-based control software that takes into account the next generation of computing architectures is paramount. Specifically, for the case of complex control, we present the Easily eXtendable Architecture for Reinforcement Learning (EXARL), which aims to support various scientific applications seeking to leverage reinforcement learning (RL) on exascale computing architectures. We demonstrate the efficacy and performance of the EXARL library for the scientific use case of designing a complex control policy to stabilize a power system after experiencing a fault. We use a parallel augmented random search method developed within EXARL and present its preliminary validation and performance stabilization of a fault for the IEEE 39-bus system.
更多查看译文
关键词
Reinforcement learning, augmented random search, exascale computing, power systems
AI 理解论文
溯源树
样例
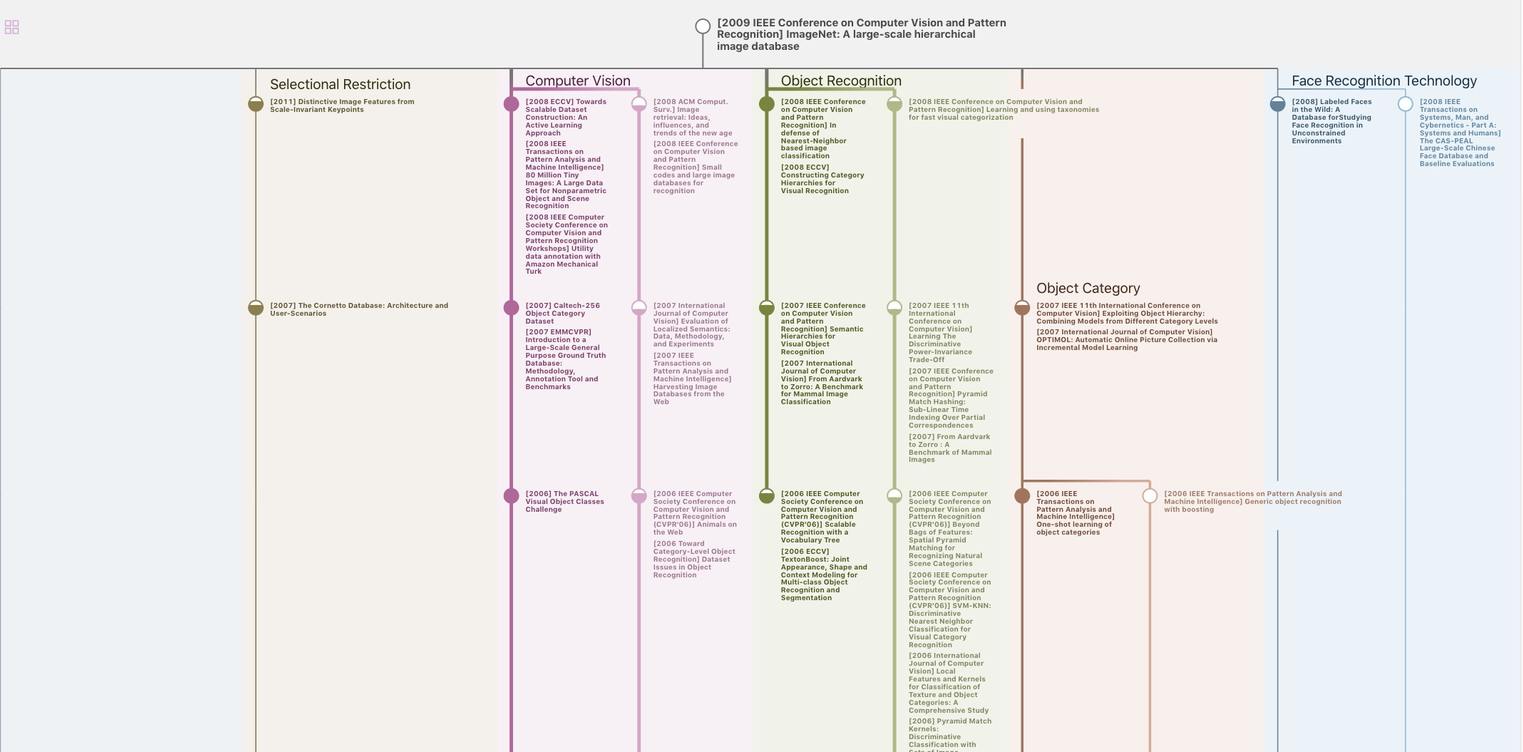
生成溯源树,研究论文发展脉络
Chat Paper
正在生成论文摘要