Learning by Reasoning: An Explainable Hierarchical Association Regularized Deep Learning Method for Disease Prediction.
HCI (28)(2023)
摘要
Multimodal healthcare data provides a huge opportunity for big-data-based disease prediction, supporting the diagnosis and treatment decision-making process for doctors. Many deep learning based methods are developed to yield better performance of multimodal data based disease prediction considering their powerful representation abilities. However, the black-box nature of deep learning methods results in many serious concerns: e.g. the reliability of the prediction performance is questionable; the end-users (i.e., doctors) can not understand the reasons behind the prediction. These issues make it difficult for deep learning based disease prediction systems to apply in practice. Therefore, we aim to tackle the aforementioned challenges and propose an explainable hierarchical association regularized deep learning method to produce interpretable prediction results while maintaining prediction accuracy. The method takes the multimodal healthcare data into consideration for the disease prediction task and constructs a hierarchical association path for each sample. An ingenious loss function is designed to learn consistent features among different data modalities and the disease prediction path with a hierarchical structure. The experimental results based on a public dataset show the superiority of our proposed method. The ablation study and sensitivity analysis verify the effectiveness and necessity of the method design.
更多查看译文
关键词
explainable hierarchical association,deep learning method,prediction,disease
AI 理解论文
溯源树
样例
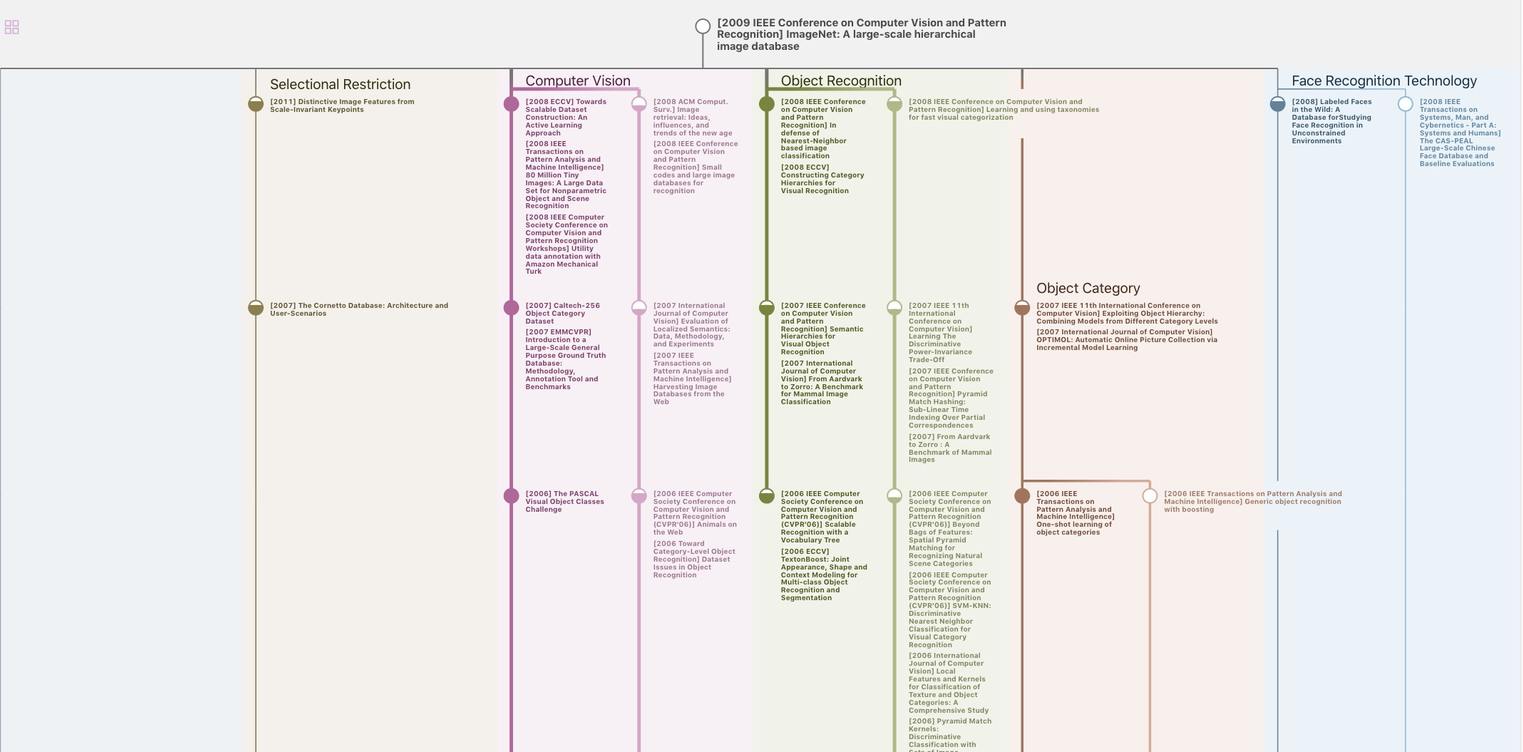
生成溯源树,研究论文发展脉络
Chat Paper
正在生成论文摘要