Towards a Robust On-line Performance Model Identification for Change Impact Prediction
SEAMS(2023)
摘要
In self-adaptive systems, model-based control assumes decisions are taken based on a model that is identified at run-time. The model is built by measuring the control inputs, disturbances, and outputs of the controlled system and fitting the data into a function. Models can be accurate locally, that is, for data already seen by the system and by the model identification method. However, many times an Autonomic Manager (AM) needs to move the cloud-native applications into new operational points, e.g. by adding new applications to the shared environment, scaling applications or consolidating resources. There are no data points yet for these new operational regions to have any certainty that the prediction models are accurate. In this paper, we propose a method to identify a model that predicts metrics at any unexplored operational point of a cloud-native application. The method is based on a lightweight Look-Ahead Scanner (LAS) mechanism that explores different operational points by injecting controlled short-lived load. We evaluate our method on realistic applications deployed on public clouds. We show that the proposed method can build models that outperform the state of the art ML models by 42%.
更多查看译文
关键词
Microservices,Performance Modeling,Cloud Computing,Machine Learning
AI 理解论文
溯源树
样例
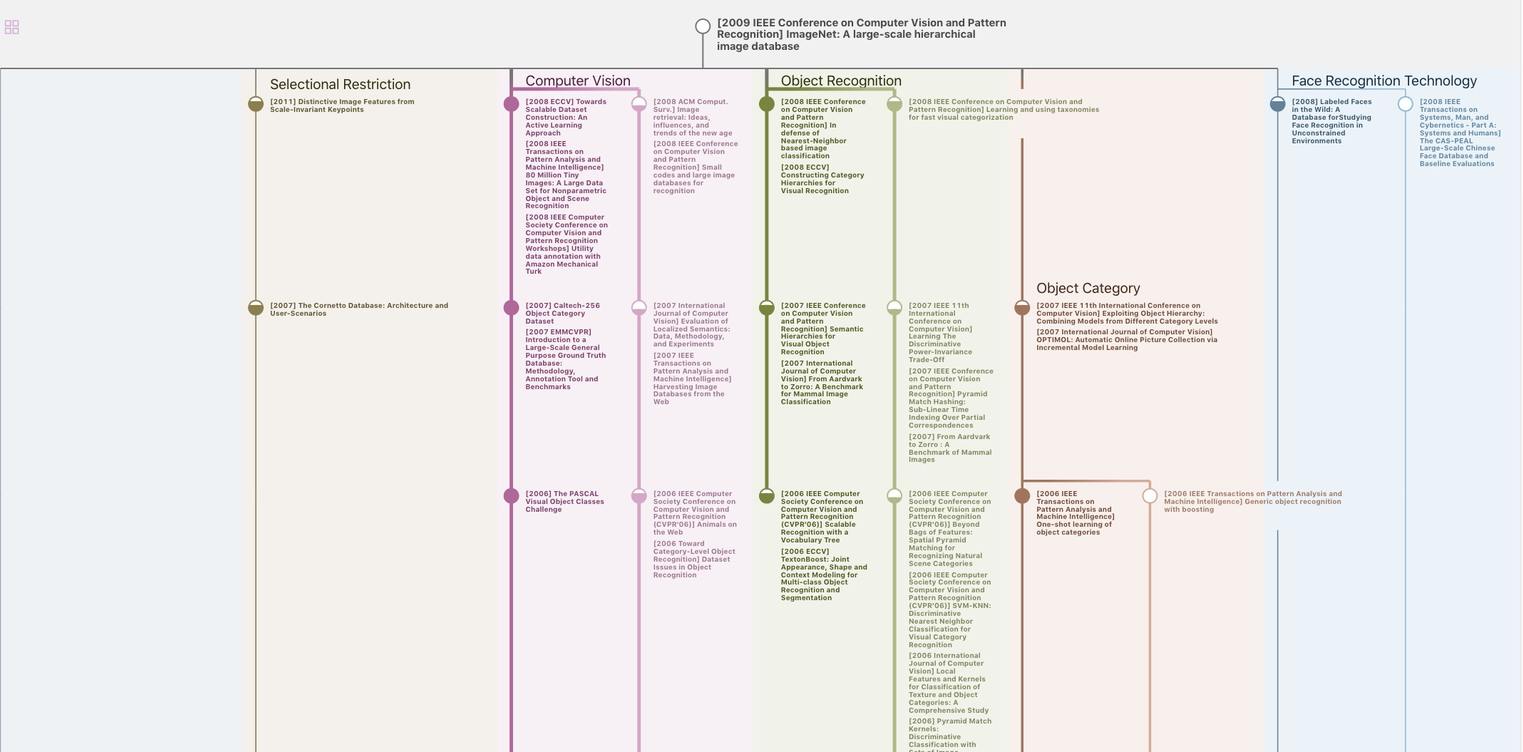
生成溯源树,研究论文发展脉络
Chat Paper
正在生成论文摘要