Local Analysis of Entropy-Regularized Stochastic Soft-Max Policy Gradient Methods
2023 EUROPEAN CONTROL CONFERENCE, ECC(2023)
摘要
Entropy regularization is an efficient technique for encouraging exploration and preventing a premature convergence of (vanilla) policy gradient methods in reinforcement learning (RL). However, the theoretical understanding of entropy-regularized RL algorithms has been limited by the assumption of exact gradient oracles. To go beyond this limitation, we study the convergence of stochastic soft-max vanilla policy gradient with entropy regularization and prove how to utilize the curvature information around the optimal policy to guarantee that the action probabilities will still remain uniformly bounded with high probability. Moreover, we develop the "last iterate" convergence and sample complexity result for the proposed algorithm given a good initialization.
更多查看译文
AI 理解论文
溯源树
样例
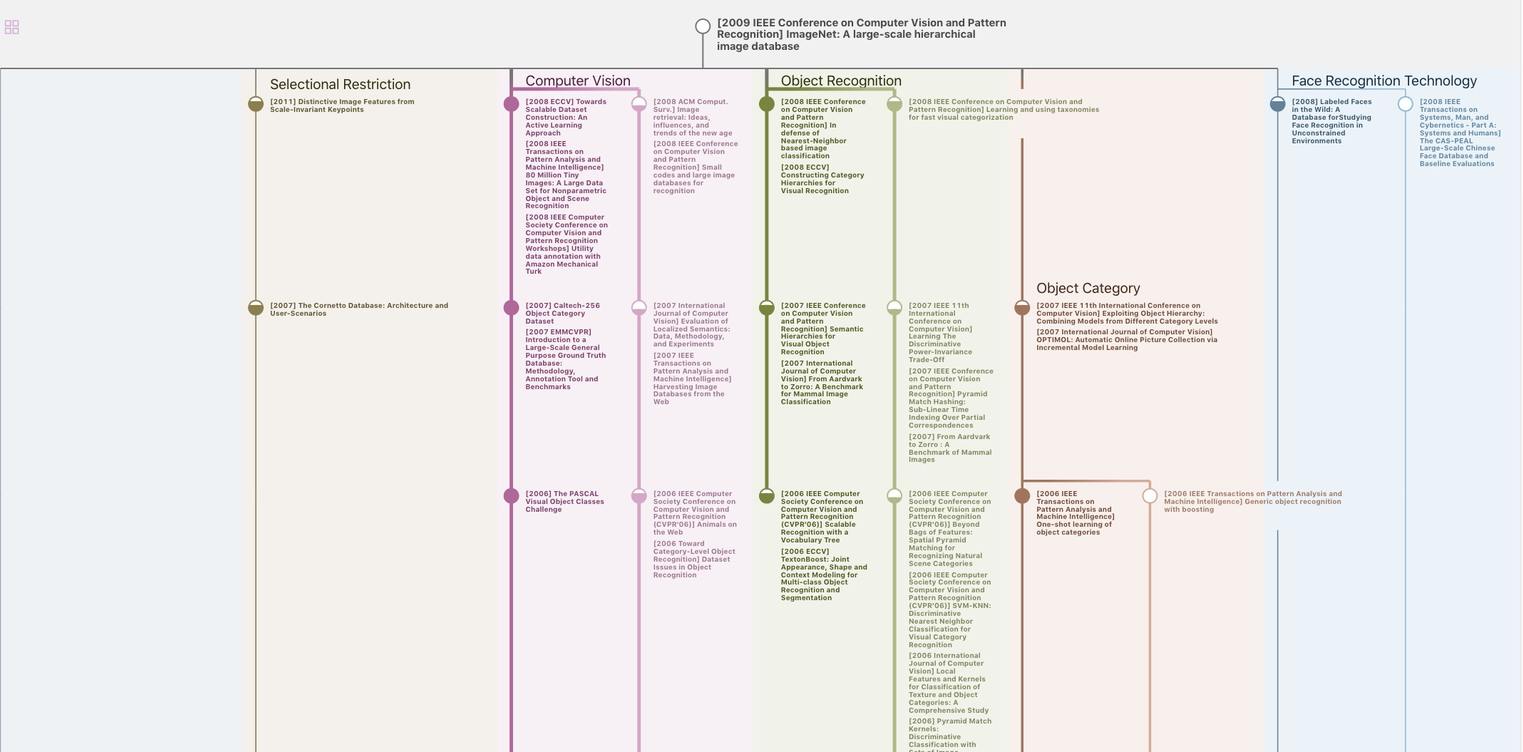
生成溯源树,研究论文发展脉络
Chat Paper
正在生成论文摘要