Alarm Flood Clustering improves Cause and Effect Analysis of Process Alarm Data
2023 EUROPEAN CONTROL CONFERENCE, ECC(2023)
摘要
Cause and effect analyses can reveal useful insights about the information flow within process alarm data, which can, in turn, be used to improve the process performance. Cause and effect analyses require selecting suitable time windows of the historical alarm data, as performing the analysis on the entire time horizon or unsuitable windows might negatively influence the analysis due to noise. This motivates the combination of alarm flood identification, clustering, and cause and effect analysis. Hence, we propose to combine a causal inference analysis based on Direct Transfer Entropy (DTE) with alarm flood detection and clustering to perform the DTE analysis on reasonable time intervals. Our approach performs an alarm data clustering first to find intervals of clusters of similar alarm floods. Afterward, we calculate the DTEs on the found alarm flood cluster intervals. Our results show that the combination of clustering and cause and effect analysis reveals results that were not feasible before. This facilitates analyzing the causal relations in the alarm data. The approach reduces the noisy influence of random alarms on the cause and effect analysis, and provides a clearer structure of the causal relations in the alarm data.
更多查看译文
AI 理解论文
溯源树
样例
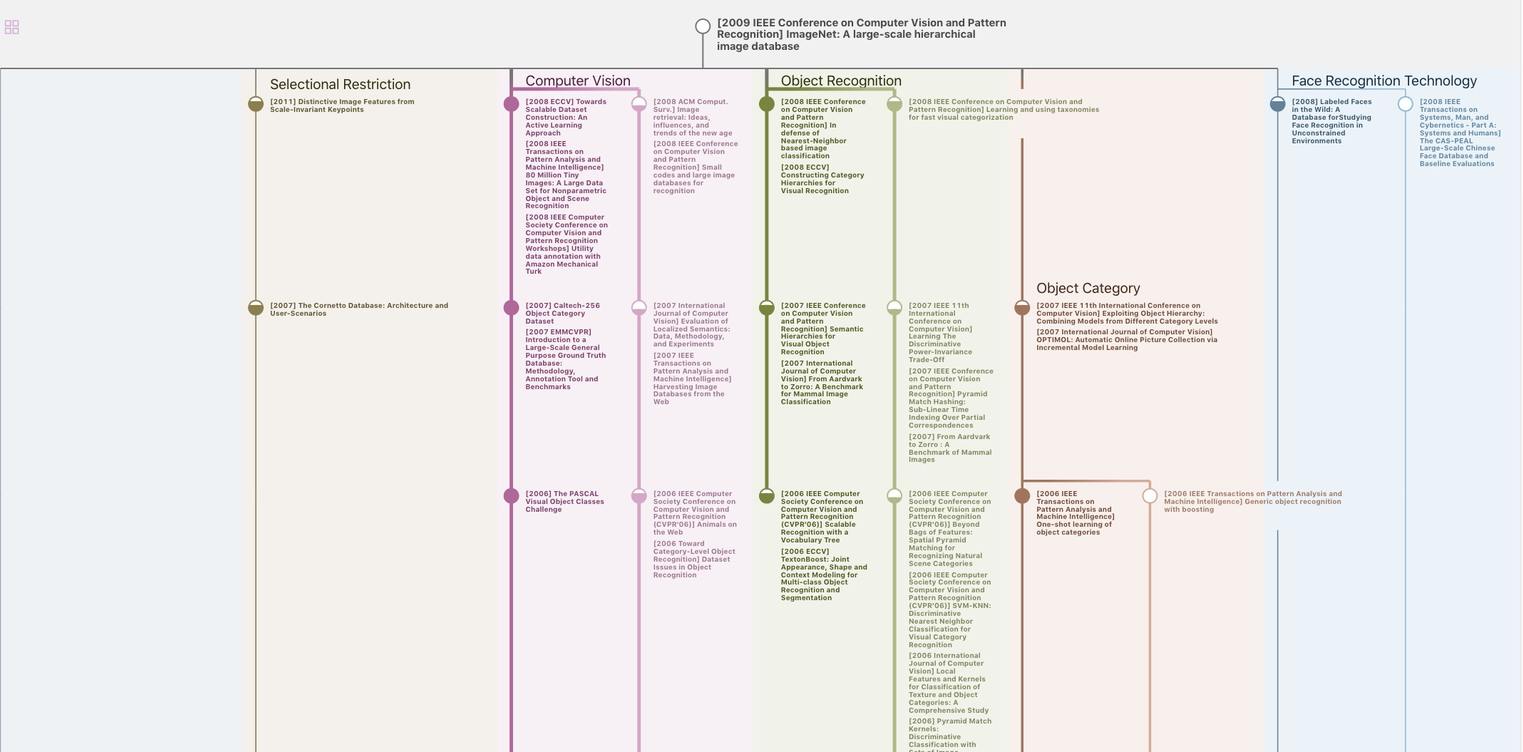
生成溯源树,研究论文发展脉络
Chat Paper
正在生成论文摘要