Efficient Collision Modelling for Numerical Optimal Control
2023 EUROPEAN CONTROL CONFERENCE, ECC(2023)
摘要
Efficiently solving optimal control problems (OCP) is a prerequisite for using methods such as model predictive control (MPC) for real-time control of complex robotic systems. For many applications, it is mandatory to guarantee that the OCP solutions correspond to collision-free motions. However, establishing appropriate constraints for OCP is not straightforward. A main reason is that the function determining the Euclidean distance between two arbitrary convex shapes is often non-differentiable and non-convex. Moreover, this function can generally only be defined implicitly, for example, by an optimization problem. Recently, it was proposed to derive collision constraints by utilizing duality theory of convex optimization as the resulting constraints are differentiable and allow to check the exact distance between arbitrary compact convex sets. These constraints naturally depend on the chosen representation of the sets for which collision avoidance should be established. In this paper, we show that the computational effort of solving OCP including the proposed constraints can be reduced by explicitly considering the type of sets involved and choosing their representation properly. To this end, we consider the two prominent cases where collision avoidance has to be established between two polytopic sets or between a polytopic and an ellipsoidal set. The claim that our proposed constraints allow improved computational performance is supported by numerical experiments with a car model.
更多查看译文
AI 理解论文
溯源树
样例
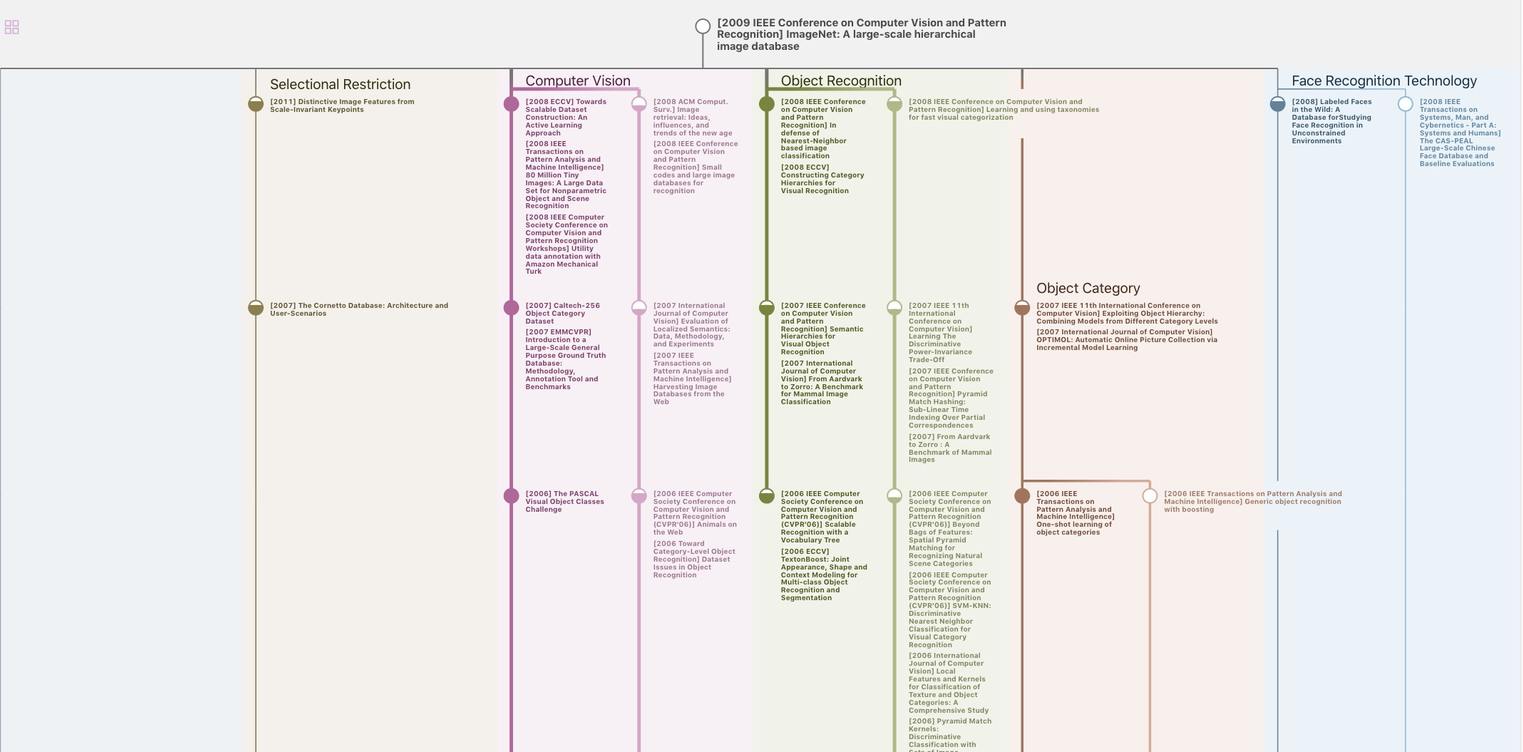
生成溯源树,研究论文发展脉络
Chat Paper
正在生成论文摘要