Payload Level Graph Attention Network for Web Attack Traffic Detection.
ICCS (5)(2023)
摘要
With the popularity of web applications, web attacks have become one of the major threats to cyberspace security. Many studies have focused on applying machine learning techniques for web attack traffic detection. However, past approaches suffer from two shortcomings: firstly, handcrafted feature extraction-based approaches are prone to introduce biases in existing perceptions, and secondly, current end-to-end deep learning approaches treat traffic payloads as non-structured string sequences ignoring their inherent structural characteristics. Therefore, we propose a graph-based web attack traffic detection model to identify the payloads in the traffic requests. Each pre-processed payload is transformed as an independent graph in which the node representations are shared through a global feature matrix. Finally, graph-level classification models are trained with graph attention networks combining global information. Experimental results on four publicly available datasets show that our approach successfully exploits local structural characteristics and global information to achieve state-of-the-art performance.
更多查看译文
关键词
attack,attention,graph,detection
AI 理解论文
溯源树
样例
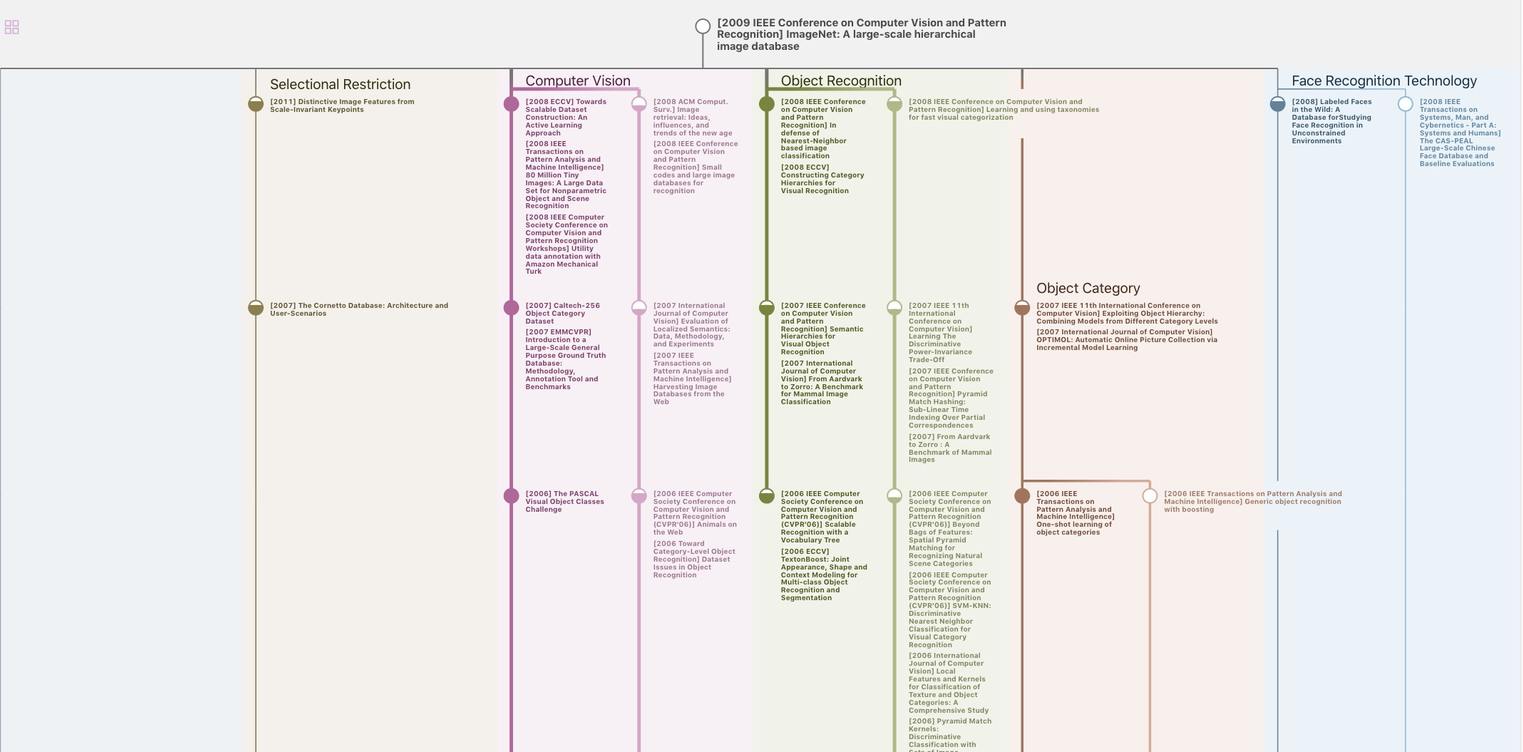
生成溯源树,研究论文发展脉络
Chat Paper
正在生成论文摘要