Predictive Analytics for University Student Admission: A Literature Review.
ICBL(2023)
摘要
This paper presents a literature review on the use of learning analytics to support prediction in university student admission. The review covers four areas: types of research issues examined, types of data, analytical techniques, and performance metrics used. A total of 59 research articles published between 2013 and 2022 in relation to the use of predictive learning analytics for student admission were collected from Scopus for analysis. The findings show the major types of research issues including admission outcome, academic performance, admission yield, chance of admission, and suitable major/field of study. The types of data frequently used include academic performance, educational background, socio-demographic data, admission-related data, and application-related data. The findings also show that logistic regression, decision tree, random forest, support vector machine, and neural network are the most commonly adopted analytical techniques, whereas accuracy, recall, precision, F-measure, and R-squared are the most frequently used performance metrics. The results contribute to identifying the features and patterns of predictive learning analytics with respect to university student admission.
更多查看译文
关键词
university student admission,analytics
AI 理解论文
溯源树
样例
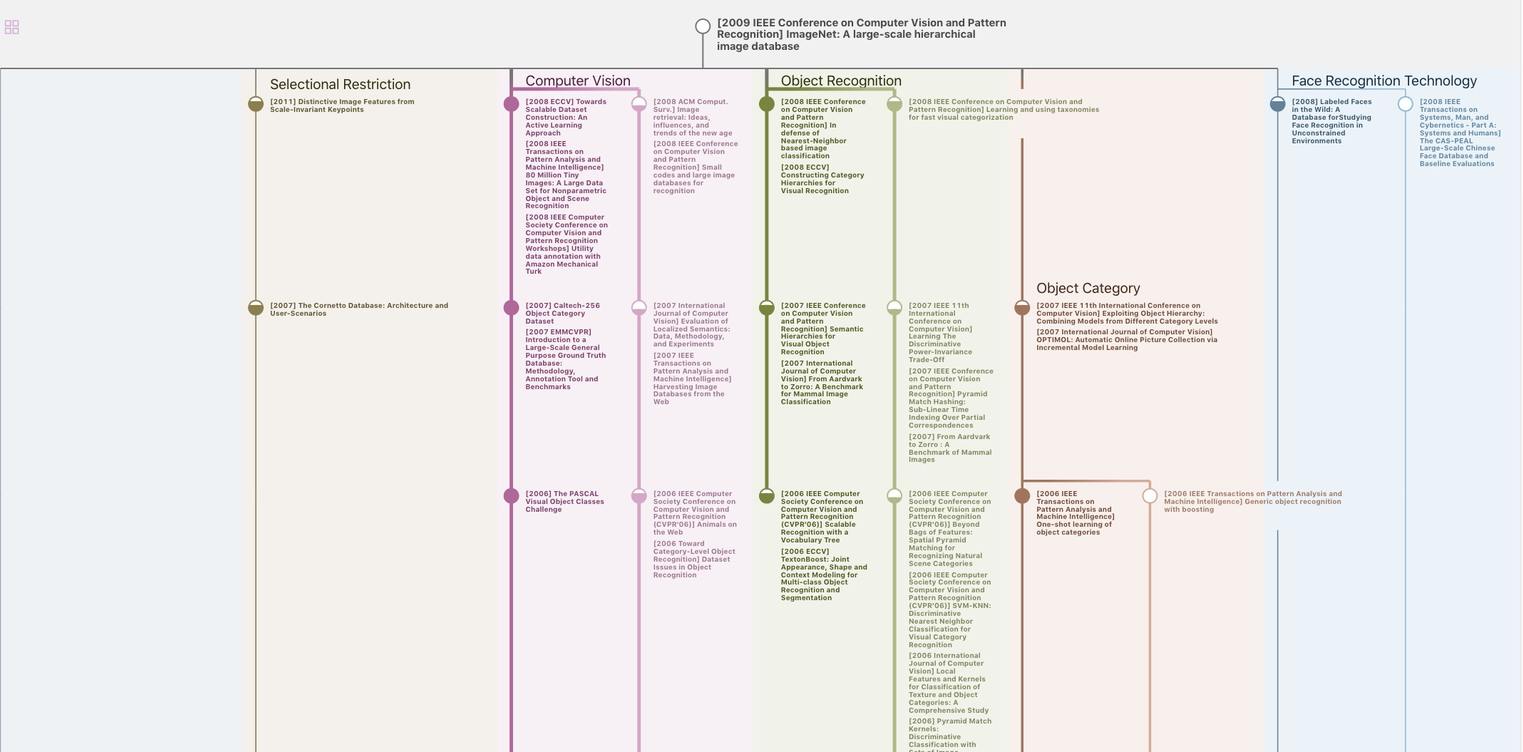
生成溯源树,研究论文发展脉络
Chat Paper
正在生成论文摘要