Multi-Surrogate Assisted PSO with Multiple Exemplars for Expensive Multimodal Multi-Objective Optimization
PROCEEDINGS OF THE 2023 GENETIC AND EVOLUTIONARY COMPUTATION CONFERENCE COMPANION, GECCO 2023 COMPANION(2023)
摘要
Expensive multimodal multi-objective problems widely exit in real-world applications, in which multiple Pareto solutions correspond to the same objective values. To tackle the above-mentioned problems, traditional particle swarm optimization algorithms always select a Pareto solution from the archive based on preferences as an exemplar. However, this exemplar may not be the best exemplar for each particle. Therefore, a multi-surrogate assisted particle swarm optimization with multiple exemplars is proposed. Firstly, a multi-surrogate model based on clustering is constructed to fit the many-to-one mapping relationship. Secondly, a surrogate assisted optimization strategy based on multiple exemplars is developed. In this strategy, each particle performs optimization under the guidance of multiple exemplars in the archive, and the optimal exemplar is selected based on the evaluations of the surrogate models. Finally, an archive update strategy based on crowding distance is proposed to balance the diversity both in the decision and objective spaces. The experimental results show that the proposed algorithm is significantly superior to eight state-of-the-art evolutionary algorithms on chosen benchmark problems.
更多查看译文
关键词
Expensive,Multimodal multi-objective,surrogate,PSO
AI 理解论文
溯源树
样例
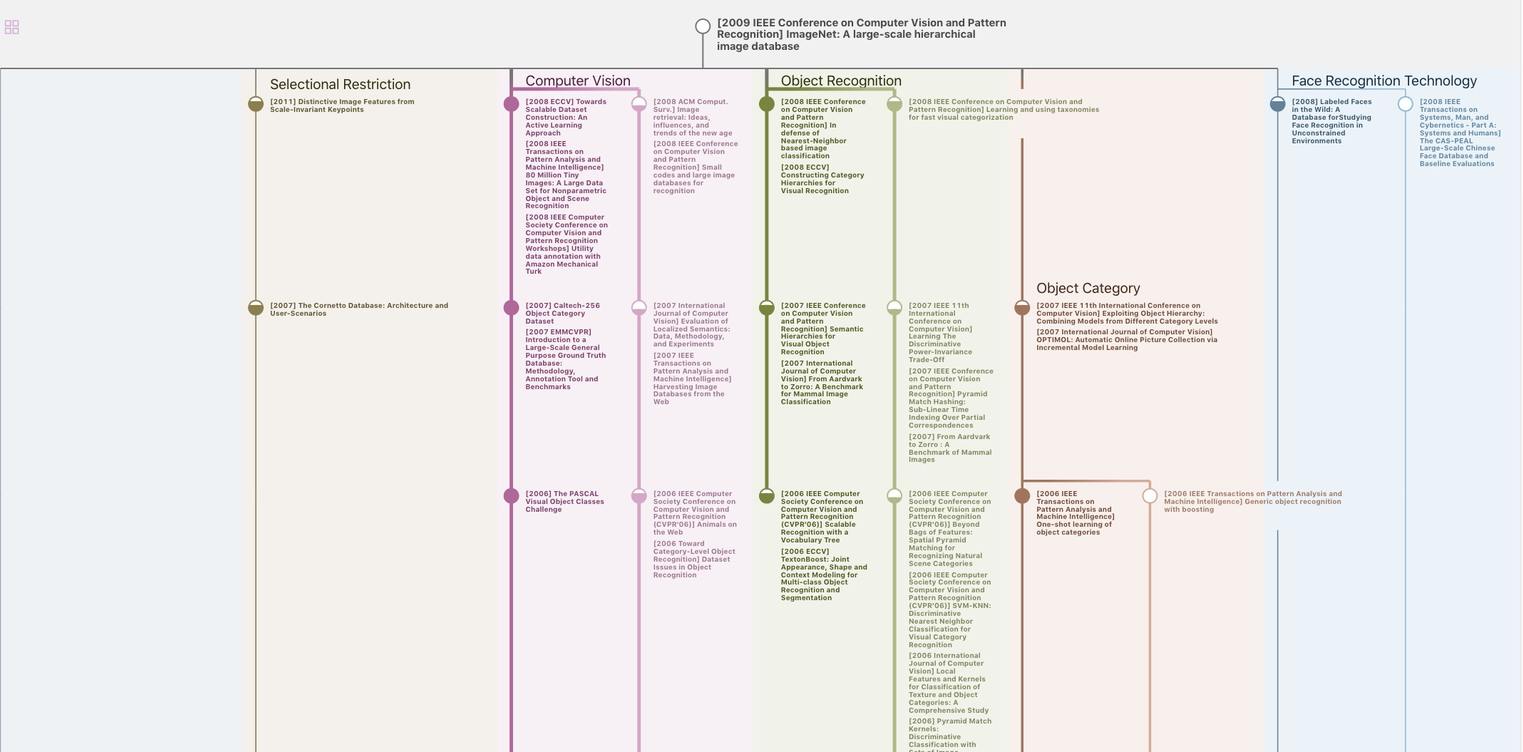
生成溯源树,研究论文发展脉络
Chat Paper
正在生成论文摘要