Self-Supervised Image Denoising Using Implicit Deep Denoiser Prior.
AAAI(2023)
摘要
We devise a new regularization for denoising with self-supervised learning. The regularization uses a deep image prior learned by the network, rather than a traditional predefined prior. Specifically, we treat the output of the network as a "prior" that we again denoise after "re-noising." The network is updated to minimize the discrepancy between the twice-denoised image and its prior. We demonstrate that this regularization enables the network to learn to denoise even if it has not seen any clean images. The effectiveness of our method is based on the fact that CNNs naturally tend to capture low-level image statistics. Since our method utilizes the image prior implicitly captured by the deep denoising CNN to guide denoising, we refer to this training strategy as an Implicit Deep Denoiser Prior (IDDP). IDDP can be seen as a mixture of learning-based methods and traditional modelbased denoising methods, in which regularization is adaptively formulated using the output of the network. We apply IDDP to various denoising tasks using only observed corrupted data and show that it achieves better denoising results than other self-supervised denoising methods.
更多查看译文
关键词
image denoising,self-supervised
AI 理解论文
溯源树
样例
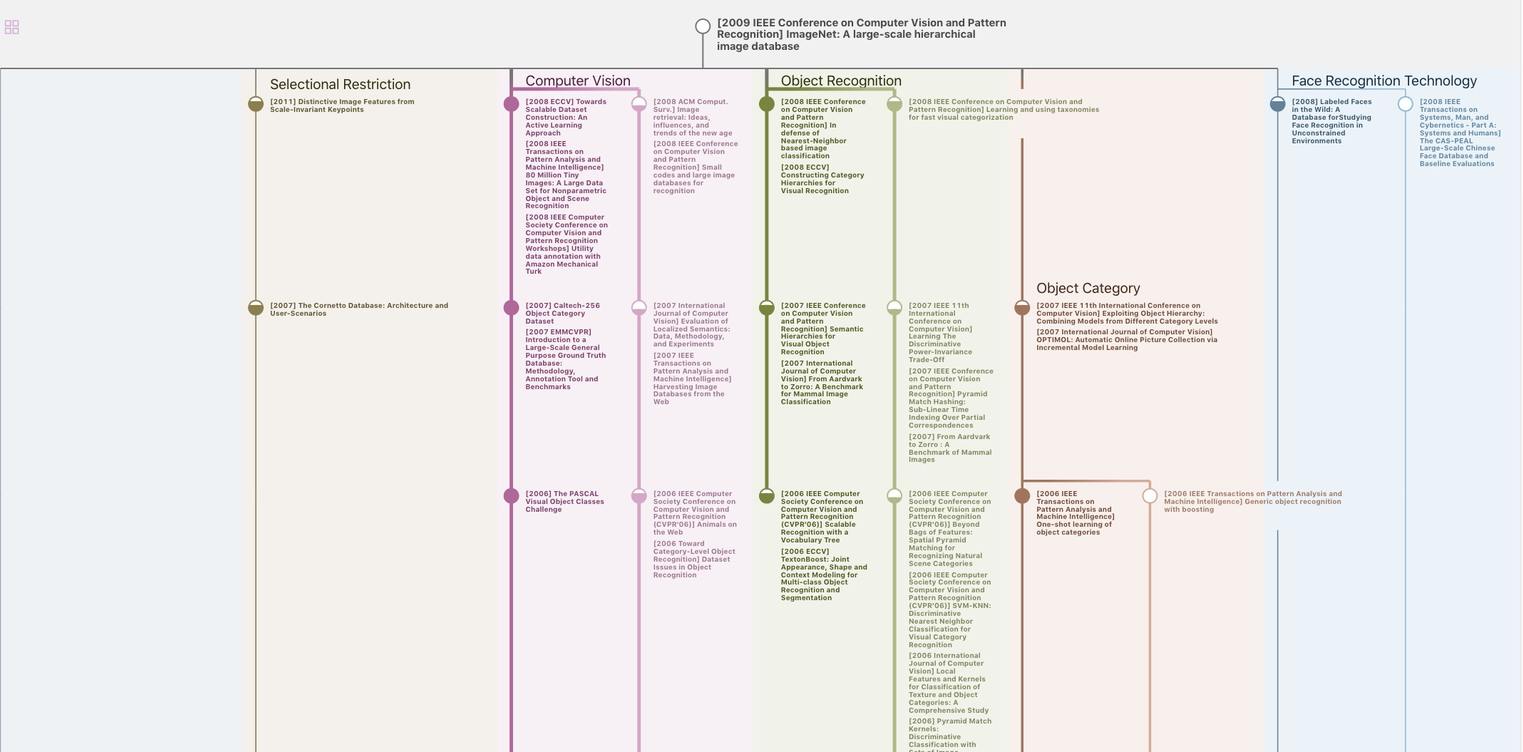
生成溯源树,研究论文发展脉络
Chat Paper
正在生成论文摘要