Electrical Fault Diagnosis via Text Mining: A Weakly-Supervised Learning Model.
CSCWD(2023)
摘要
To automatically and accurately identify electrical fault categories by means of data-driven approaches has attracted much attention in recent years. However, the research on fault diagnosis by mining unstructured data such as text data is far from being perfect. One problem lies in that a large number of human-labeled samples is often costly and difficult to obtain in real applications. In this study, we present a novel Label Embedding joint with Weakly-supervised Classification model (LEMWEC) for predicting fault category given the fault-descriptive corpus. Our model leverages a few labeled documents and a good number of pseudo-labeled documents to learn both sentence embeddings and the multi-label classifier. Then, the model will be iteratively refined by the newly generated pseudo documents. Experiments on a real-life dataset demonstrate the LEMWEC can improve the accuracy of electrical fault classification remarkably compared with a comprehensive set of baselines.
更多查看译文
关键词
Electrical fault identification,weakly-supervised learning,semi-supervised learning,text classification
AI 理解论文
溯源树
样例
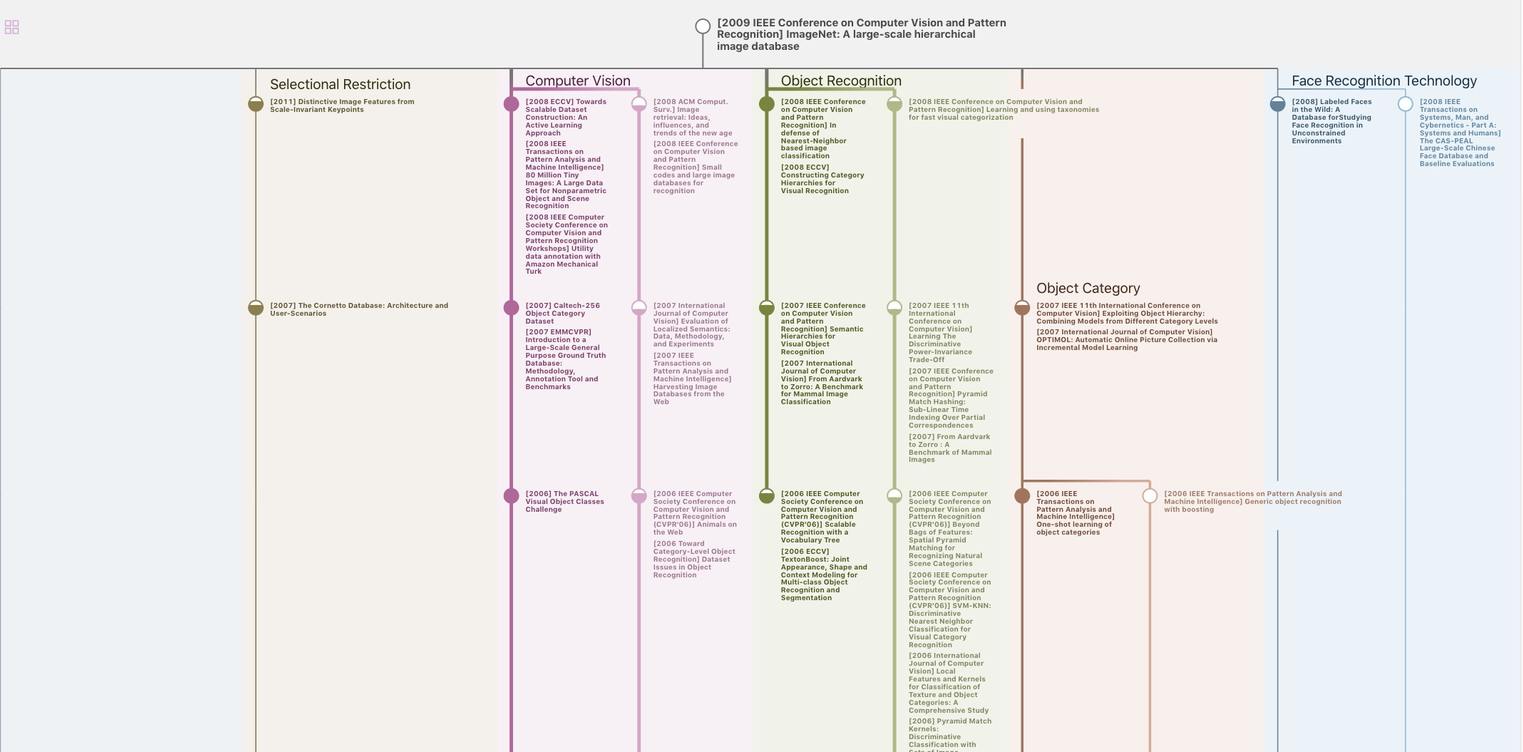
生成溯源树,研究论文发展脉络
Chat Paper
正在生成论文摘要