Going Haywire: False Friends in Federated Learning and How to Find Them.
AsiaCCS(2023)
摘要
Federated Learning (FL) promises to offer a major paradigm shift in the way deep learning models are trained at scale, yet malicious clients can surreptitiously embed backdoors into models via trivial augmentation on their own subset of the data. This is especially true in small- and medium-scale FL systems, which consist of dozens, rather than millions, of clients. In this work, we investigate a novel attack scenario for an FL architecture consisting of multiple noni.i.d. silos of data in which each distribution has a unique backdoor attacker and where the model convergences of adversaries are not more similar than those of benign clients. We propose a newmethod, dubbed Haywire, as a security-in-depth approach to respond to this novel attack scenario. Our defense utilizes a combination of kPCA dimensionality reduction of fully-connected layers in the network, KMeans anomaly detection to drop anomalous clients, and server aggregation robust to outliers via the Geometric Median. Our solution prevents the contamination of the global model despite having no access to the backdoor triggers. We evaluate the performance of Haywire from model-accuracy, defense-performance, and attack-success perspectives against multiple baselines. Through an extensive set of experiments, we find that Haywire produces the best performances at preventing backdoor attacks while simultaneously not unfairly penalizing benign clients. We carried out additional in-depth experiments across multiple runs that demonstrate the reliability of Haywire.
更多查看译文
关键词
federated learning, model poisoning attack, metrics
AI 理解论文
溯源树
样例
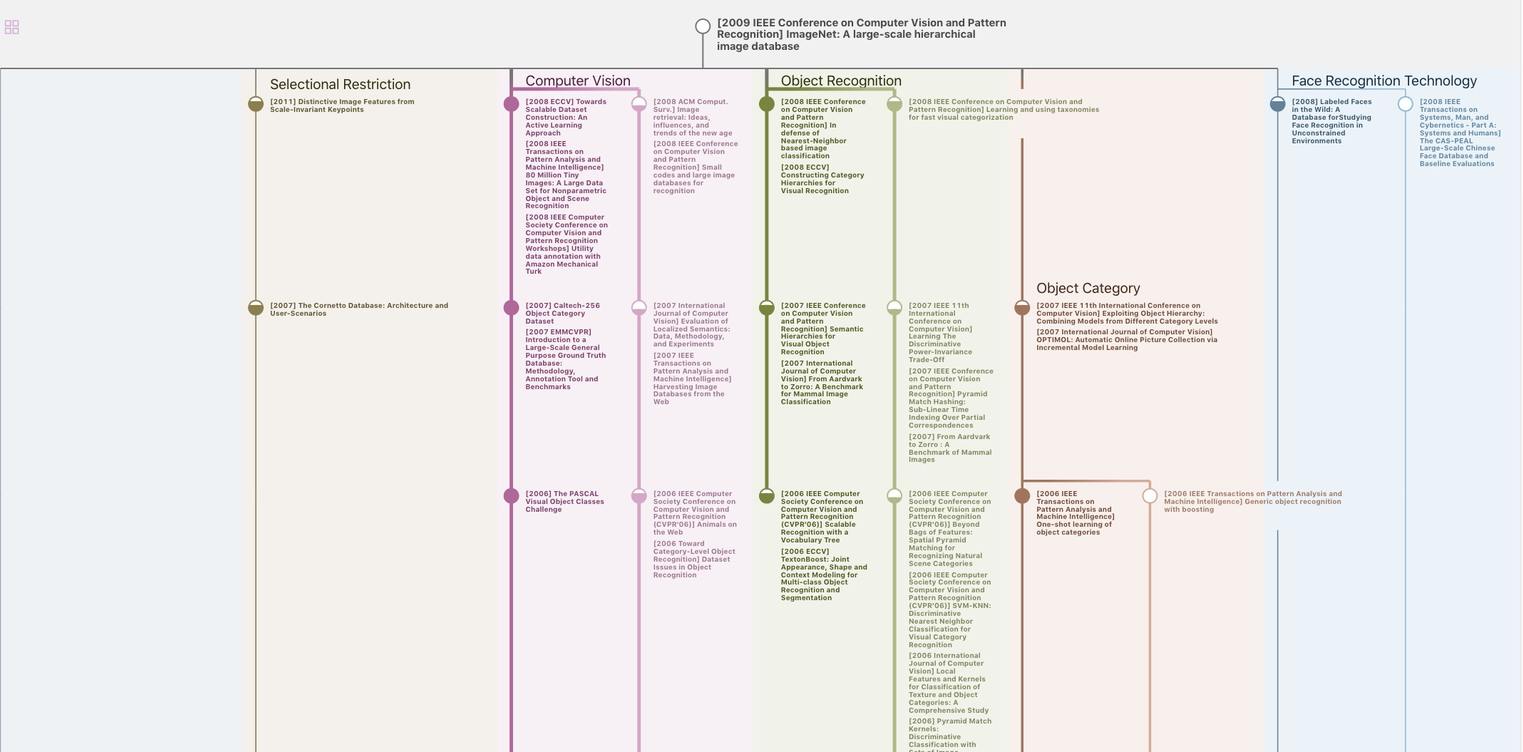
生成溯源树,研究论文发展脉络
Chat Paper
正在生成论文摘要