Elastic Data Binning for Transient Pattern Analysis in Time-Domain Astrophysics
38TH ANNUAL ACM SYMPOSIUM ON APPLIED COMPUTING, SAC 2023(2023)
摘要
Time-domain astrophysics analysis (TDAA) involves observational surveys of celestial phenomena that may contain irrelevant information because of several factors, one of which is the sensitivity of the optical telescopes. Data binning is a typical technique for removing inconsistencies and clarifying the main characteristic of the original data in astrophysics analysis. It splits the data sequence into smaller bins with a fixed size and subsequently sketches them into a new representation form. In this study, we introduce a novel approach, called elastic data binning (EBinning), to automatically adjust each bin size using two statistical metrics based on the Student's t-test for linear regression and Hoeffding inequality. We demonstrate the successful representation of various characteristics in the light curve data gathered from the Kiso Schmidt telescope using our approach and the applicability of our approach for transient pattern analysis using real world data.
更多查看译文
关键词
Data binning,Data sketching,Hoeffding inequality,Student's t-test,Lightcurve
AI 理解论文
溯源树
样例
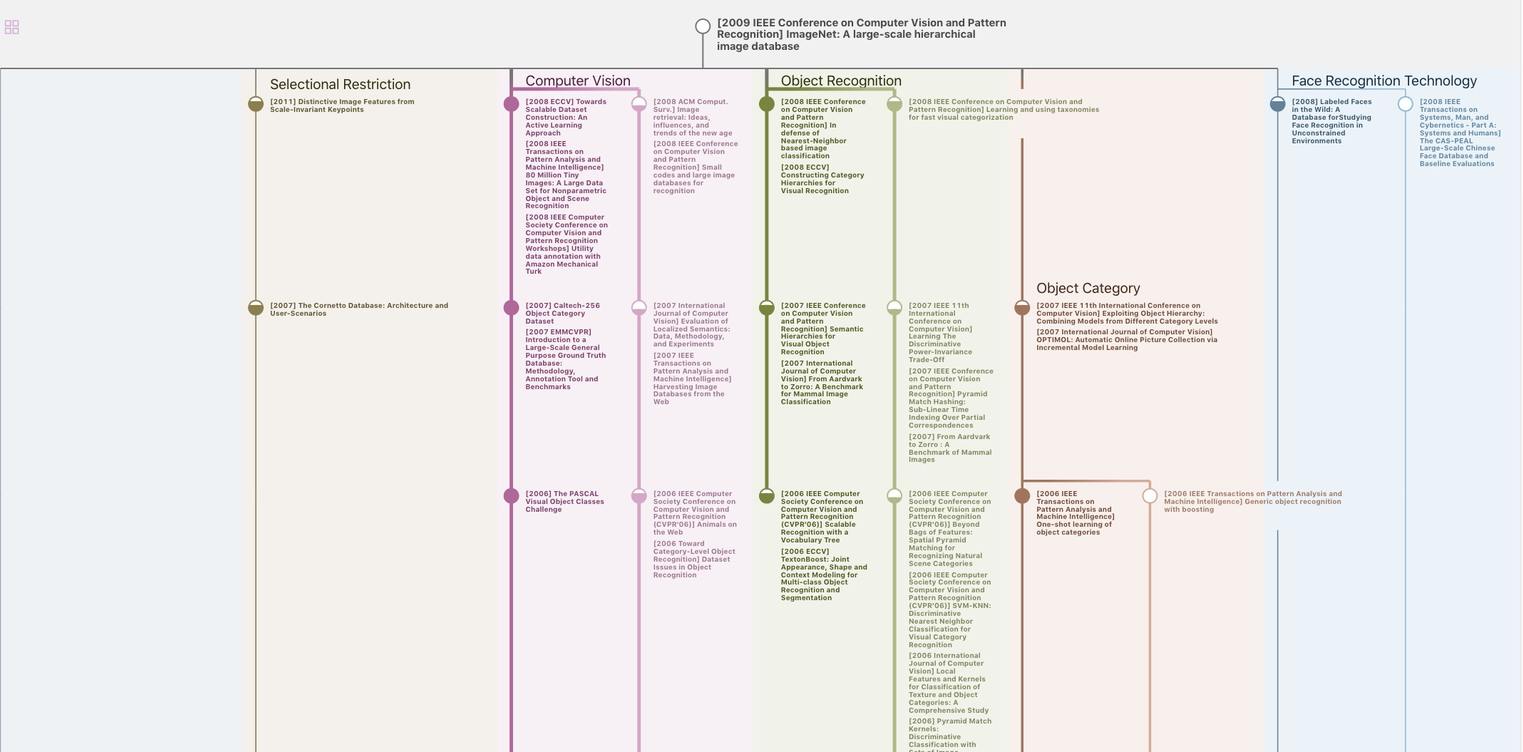
生成溯源树,研究论文发展脉络
Chat Paper
正在生成论文摘要