Privileged Label Enhancement with Adaptive Graph.
CSCWD(2023)
摘要
Label distribution learning has gained an increasing amount of attention in comparison to single-label and multi-label learning due to its more universal capacity to communicate label ambiguity. Unfortunately, label distribution learning cannot be used directly in many real tasks, because it is very difficult to obtain the label distribution datasets, and many training sets only contain simple logical labels. To resolve this problem and recover the label distributions from the logical labels, label enhancement is proposed. This paper proposes a novel label enhancement algorithm called Privileged Label Enhancement with Adaptive Graph(PLEAG). PLEAG first apply adaptive graph to capture the hidden information between instances and treat it as privileged information. As a result, the similarity matrix of instances is not only influenced by the feature space, but is also adaptively modified in accordance with the degree of similarity between instances in the label space. Then, we adopt RSVM+ model in the paradigm of LUPI (learning with privileged information) to handle the new dataset with privileged information in order to gain better learning effect. Our comparison experiments on 12 datasets show that our proposed algorithm PLEAG , is more accurate than prior label enhancement algorithms for recovering label distribution from logical labels.
更多查看译文
关键词
label enhancement,label distribution learning,adaptive graph,big data
AI 理解论文
溯源树
样例
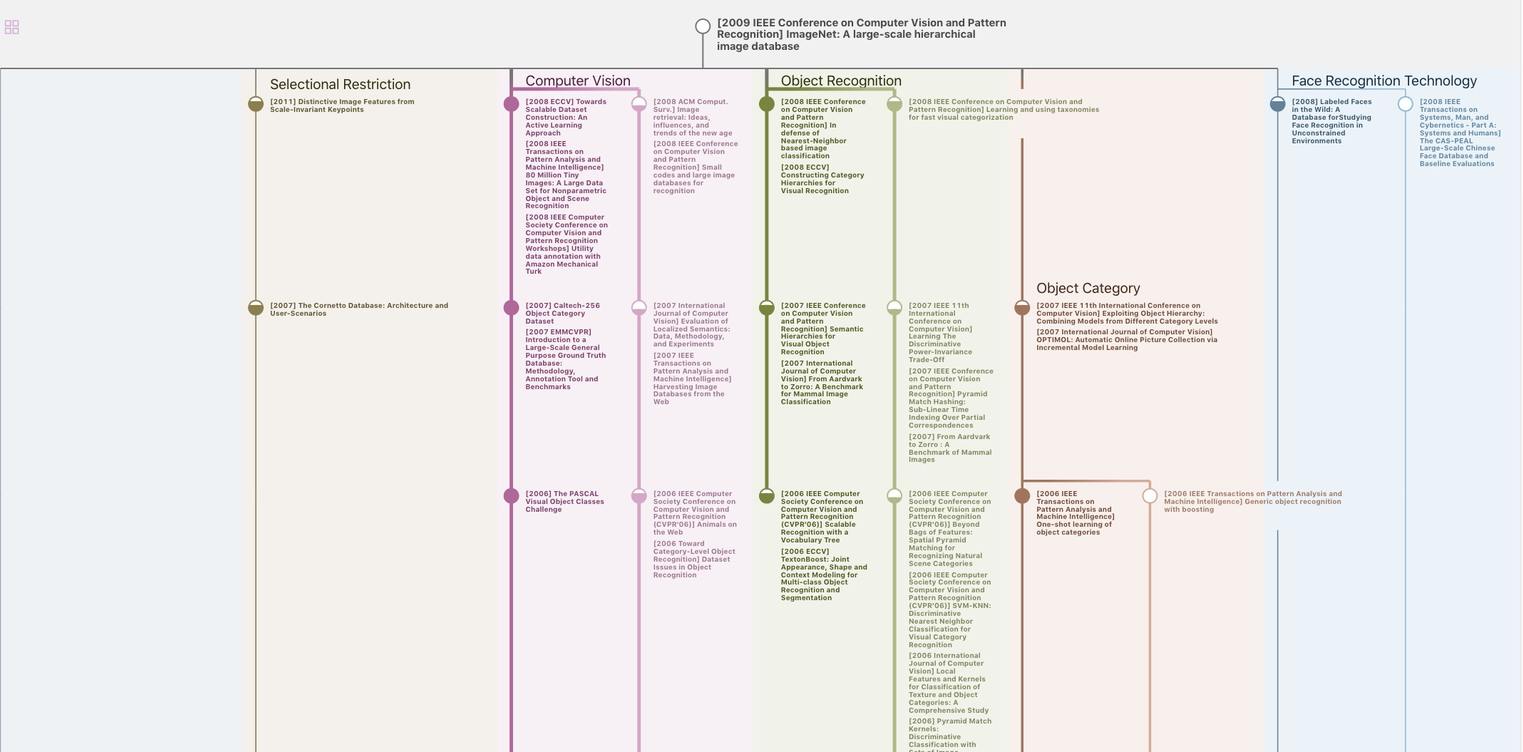
生成溯源树,研究论文发展脉络
Chat Paper
正在生成论文摘要