Effects of Including Optimal Solutions in the Initial Population on Evolutionary Multi-objective Optimization
GECCO(2023)
摘要
A long-standing question in the evolutionary multi-objective (EMO) community is how to generate a good initial population for EMO algorithms. Intuitively, as the starting point of optimization, a good initial population can have positive effects on the performance of EMO algorithms. However, in most existing EMO algorithms, one of the commonly-used initialization methods is to randomly generate a set of solutions as an initial population. One possible approach to improve random initialization is to include one or more Pareto optimal (near Pareto optimal) solution(s) in the initial population, which are expected to provide useful information and knowledge on the optimized problem. In this paper, to investigate the effectiveness of this initialization idea, we examine and quantify the effects of including one or more Pareto optimal solution(s) in the initial population on the performance of EMO algorithms. Experimental results demonstrate that it is worthwhile to first obtain and then include some Pareto optimal solutions in the initial population. Through a number of experiments and algorithm behavior analysis, this study provides supports and insights into EMO algorithm design and motivates further research on population initialization for EMO algorithms.
更多查看译文
关键词
Evolutionary computation,EMO algorithms,Initialization method,Multi-objective optimization
AI 理解论文
溯源树
样例
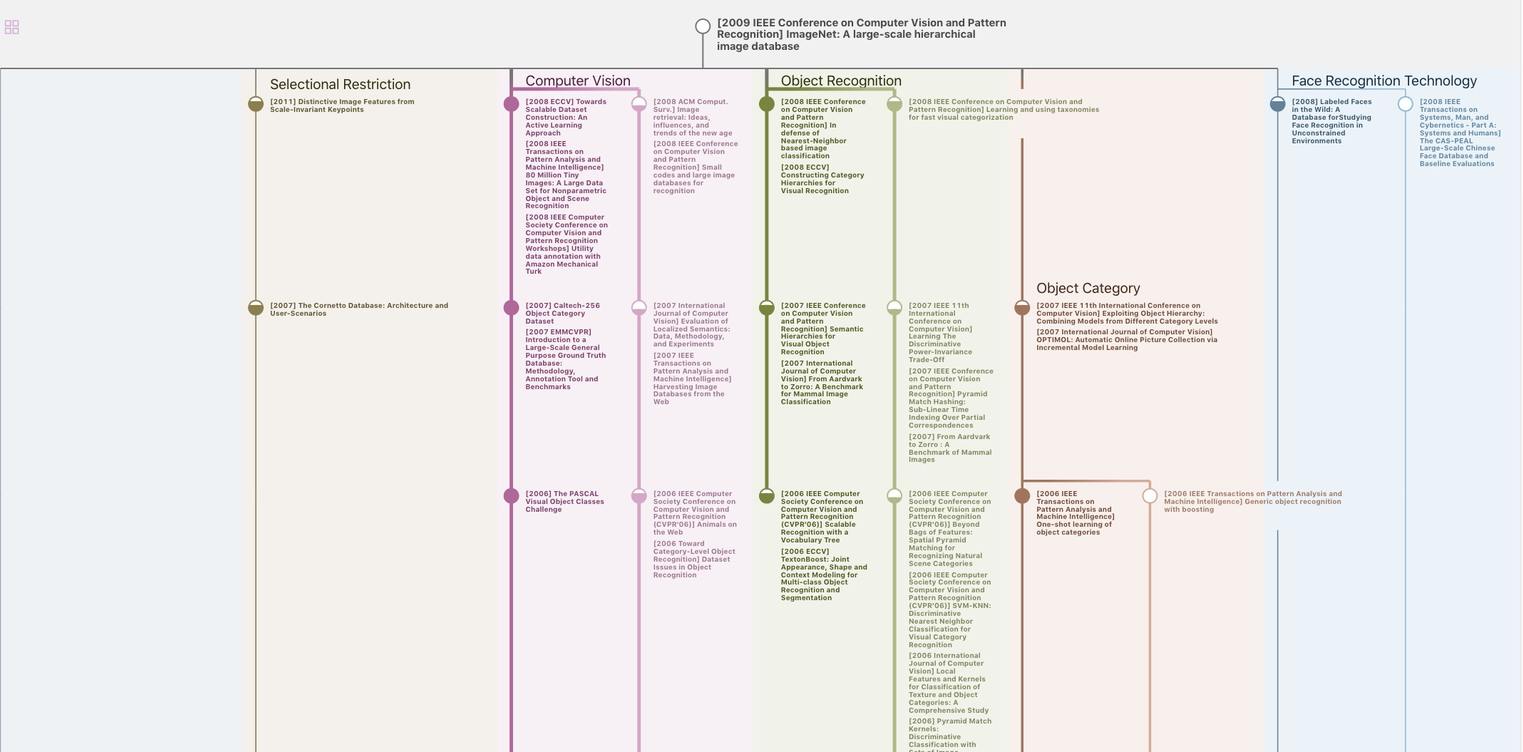
生成溯源树,研究论文发展脉络
Chat Paper
正在生成论文摘要