A $2.81\mu \mathrm{W}$, Energy Efficient MFCC Feature Extractor for Keyword-Spotting in 65nm CMOS.
ICCCS(2023)
摘要
Keyword-spotting (KWS) plays a crucial role in human machine interactions involving smart devices. And in most of the KWS systems, Mel Frequency Cepstral Coefficients (MFCC) acts as the feature extractor and consumes an unneglectable amount of computational load and resource. In this paper, to reach a trade-off between accuracy and power consumption, we proposed a modified MFCC algorithm, the computational load of which can be reduced by 82% for multiplications and 66% for additions. Using the Google Speech Command Dataset (GSCD), 96.09% accuracy is achieved for ten keywords. Then, following a hardware/algorithm co-optimized approach, we design an energy efficient hardware architecture for MFCC. Implemented in SMIC 65nm process, our circuit achieves a power consumption of
$2.81 \mu\mathrm{W}$
while operating at 1.0V supply voltage with 16kHz clock, which is 4.5x less than the related work that under the same process technology.
更多查看译文
关键词
keyword spotting (KWS),Mel Frequency Cepstral Coefficients (MFCC),real-valued fast Fourier transform (RFFT),feature extraction,MFCC hardware
AI 理解论文
溯源树
样例
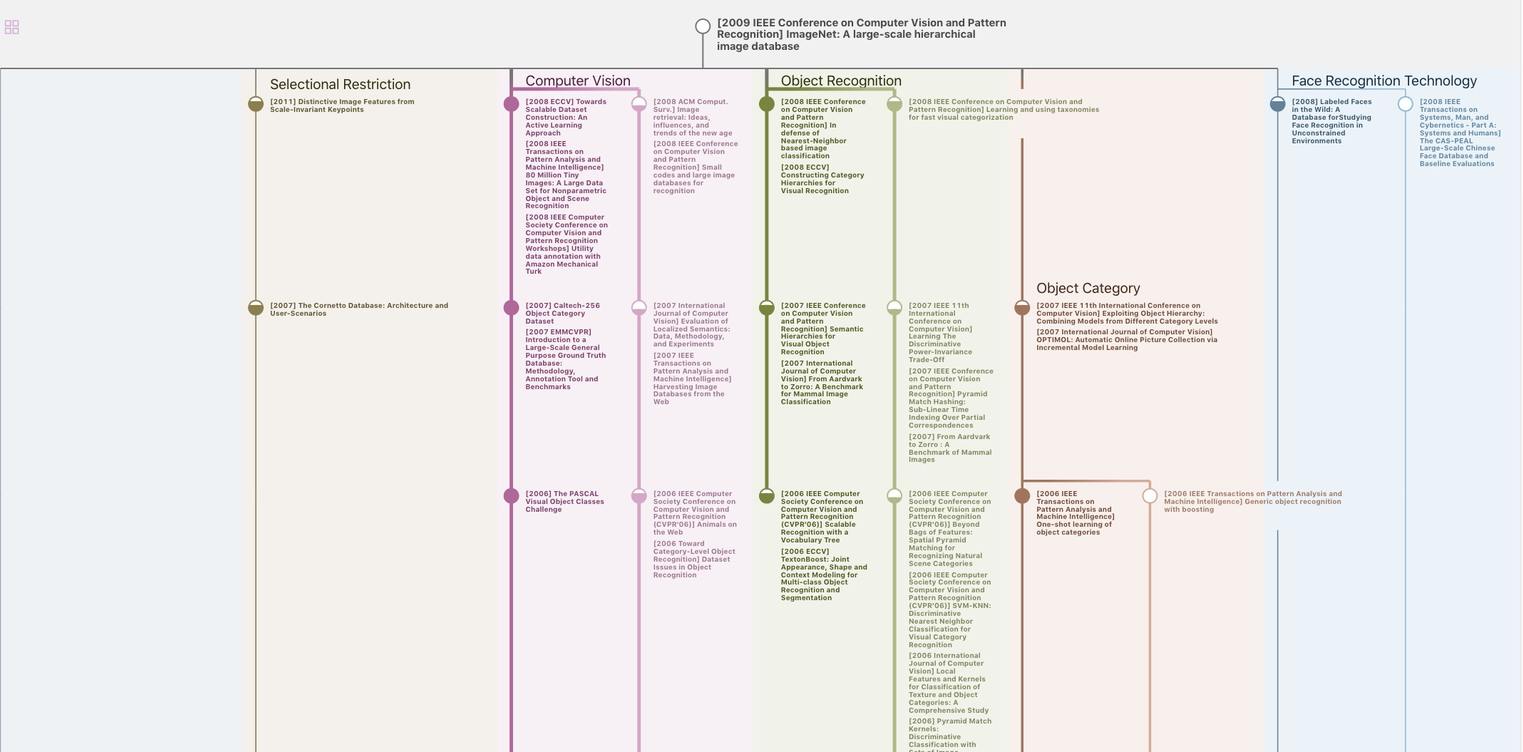
生成溯源树,研究论文发展脉络
Chat Paper
正在生成论文摘要