Fine-Grained Preference-Aware Personalized Federated POI Recommendation with Data Sparsity
PROCEEDINGS OF THE 46TH INTERNATIONAL ACM SIGIR CONFERENCE ON RESEARCH AND DEVELOPMENT IN INFORMATION RETRIEVAL, SIGIR 2023(2023)
摘要
With the raised privacy concerns and rigorous data regulations, federated learning has become a hot collaborative learning paradigm for the recommendation model without sharing the highly sensitive POI data. However, the time-sensitive, heterogeneous, and limited POI records seriously restrict the development of federated POI recommendation. To this end, in this paper, we design the fine-grained preference-aware personalized federated POI recommendation framework, namely PrefFedPOI, under extremely sparse historical trajectories to address the above challenges. In details, PrefFedPOI extracts the fine-grained preference of current time slot by combining historical recent preferences and periodic preferences within each local client. Due to the extreme lack of POI data in some time slots, a data amount aware selective strategy is designed for model parameters uploading. Moreover, a performance enhanced clustering mechanism with reinforcement learning is proposed to capture the preference relatedness among all clients to encourage the positive knowledge sharing. Furthermore, a clustering teacher network is designed for improving efficiency by clustering guidance. Extensive experiments are conducted on two diverse real-world datasets to demonstrate the effectiveness of proposed PrefFedPOI comparing with state-of-the-arts. In particular, personalized PrefFedPOI can achieve 7% accuracy improvement on average among data-sparsity clients.
更多查看译文
关键词
next POI recommendation,federated learning,fine-grained preference,personalized recommendation
AI 理解论文
溯源树
样例
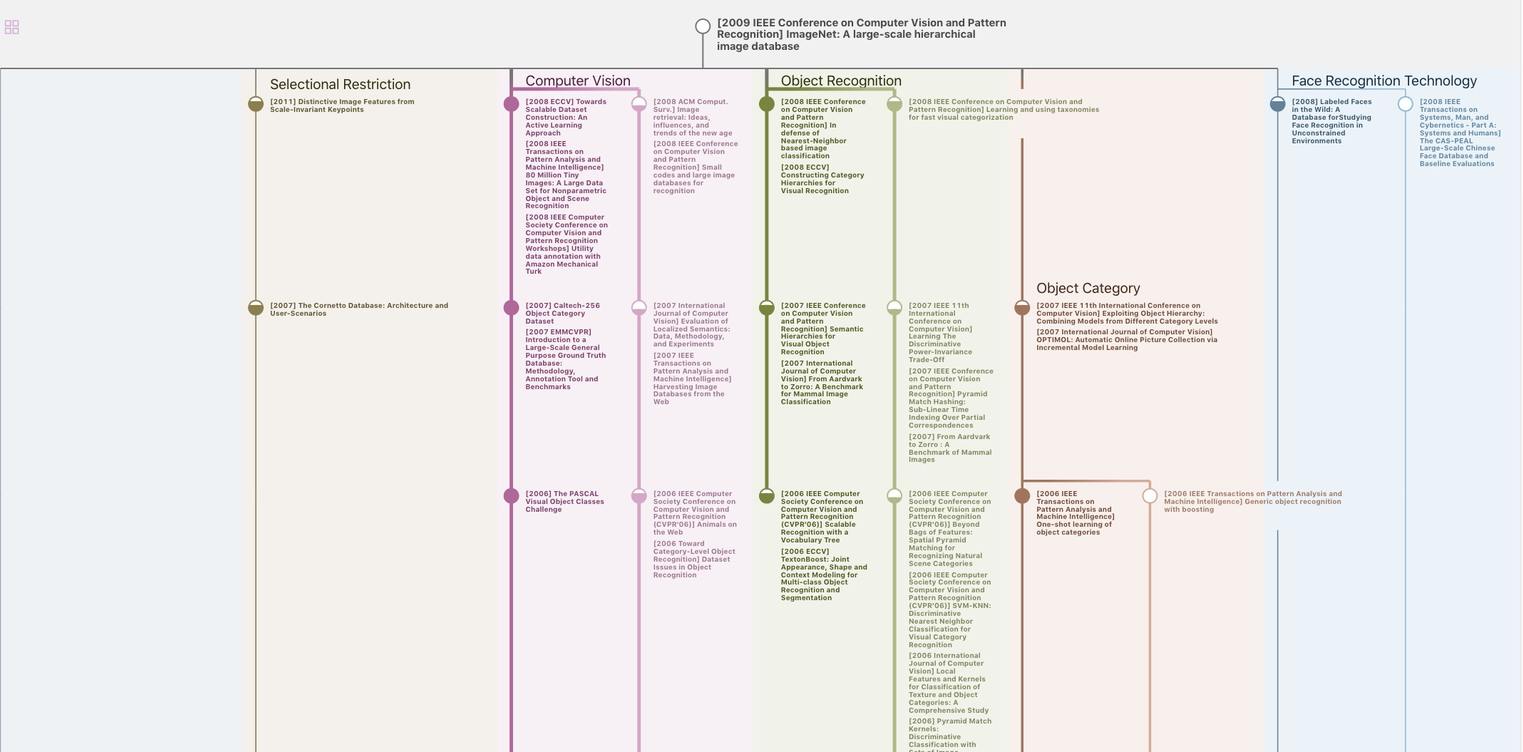
生成溯源树,研究论文发展脉络
Chat Paper
正在生成论文摘要