Rectifying Unfairness in Recommendation Feedback Loop
PROCEEDINGS OF THE 46TH INTERNATIONAL ACM SIGIR CONFERENCE ON RESEARCH AND DEVELOPMENT IN INFORMATION RETRIEVAL, SIGIR 2023(2023)
摘要
The issue of fairness in recommendation systems has recently become a matter of growing concern for both the academic and industrial sectors due to the potential for bias in machine learning models. One such bias is that of feedback loops, where the collection of data from an unfair online system hinders the accurate evaluation of the relevance scores between users and items. Given that recommendation systems often recommend popular content and vendors, the underlying relevance scores between users and items may not be accurately represented in the training data. Hence, this creates a feedback loop in which the user is not longer recommended based on their true relevance score but instead based on biased training data. To address this problem of feedback loops, we propose a two-stage representation learning framework, B-FAIR, aimed at rectifying the unfairness caused by biased historical data in recommendation systems. The framework disentangles the context data into sensitive and non-sensitive components using a variational autoencoder and then applies a novel Balanced Fairness Objective (BFO) to remove bias in the observational data when training a recommendation model. The efficacy of B-FAIR is demonstrated through experiments on both synthetic and real-world benchmarks, showing improved performance over state-of-the-art algorithms.
更多查看译文
关键词
fairness learning,recommendation system,recommendation feed-back loop,user-item fairness,unbias learning
AI 理解论文
溯源树
样例
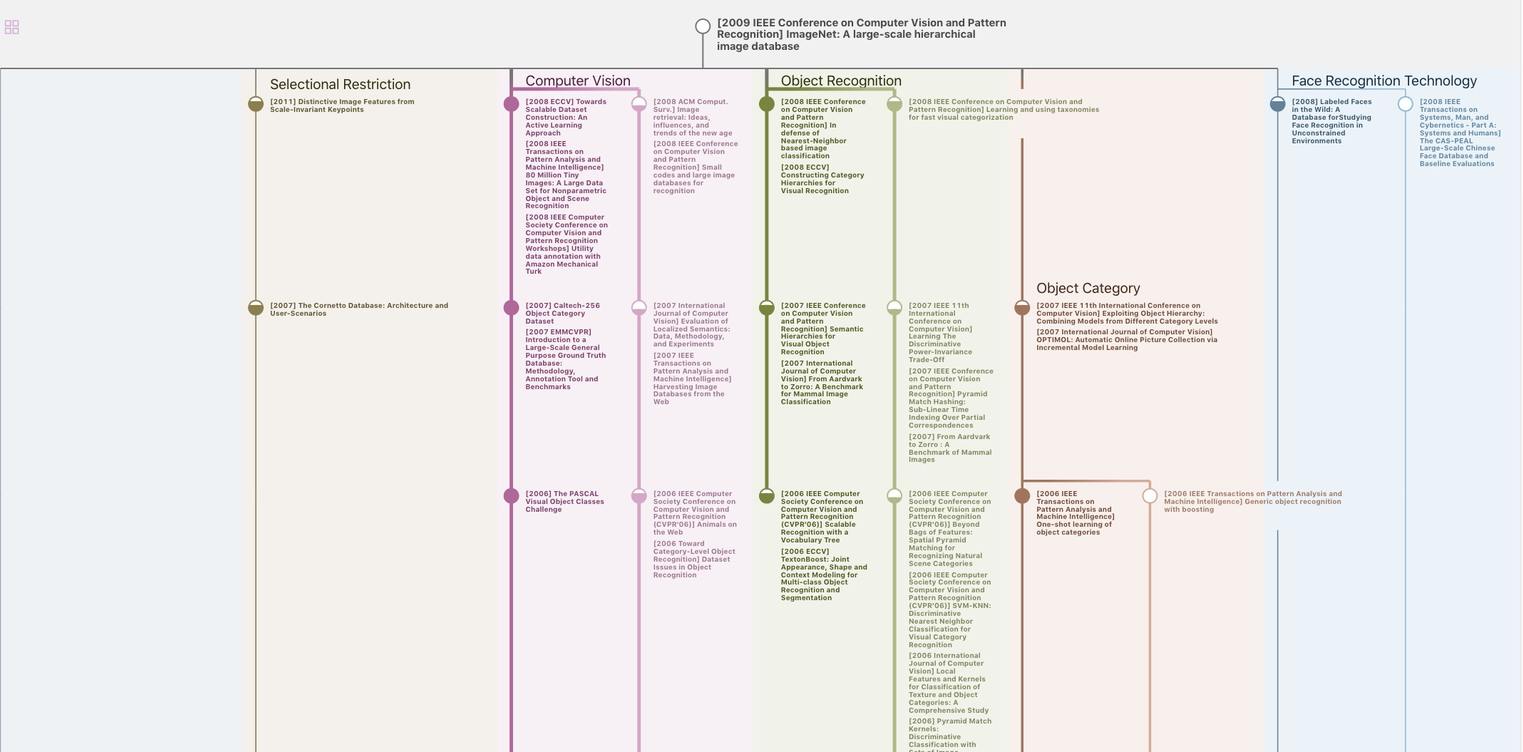
生成溯源树,研究论文发展脉络
Chat Paper
正在生成论文摘要