Candidate-aware Graph Contrastive Learning for Recommendation
PROCEEDINGS OF THE 46TH INTERNATIONAL ACM SIGIR CONFERENCE ON RESEARCH AND DEVELOPMENT IN INFORMATION RETRIEVAL, SIGIR 2023(2023)
摘要
Recently, Graph Neural Networks (GNNs) have become a mainstream recommender system method, where it captures high-order collaborative signals between nodes by performing convolution operations on the user-item interaction graph to predict user preferences for different items. However, in real scenarios, the useritem interaction graph is extremely sparse, which means numerous users only interact with a small number of items, resulting in the inability of GNN in learning high-quality node embeddings. To alleviate this problem, the Graph Contrastive Learning (GCL)-based recommender system method is proposed. GCL improves embedding quality by maximizing the similarity of the positive pair and minimizing the similarity of the negative pair. However, most GCL-based methods use heuristic data augmentation methods, i.e., random node/edge drop and attribute masking, to construct contrastive pairs, resulting in the loss of important information. To solve the problems in GCL-based methods, we propose a novel method, Candidate-aware Graph Contrastive Learning for Recommendation, called CGCL. In CGCL, we explore the relationship between the user and the candidate item in the embedding at different layers and use similar semantic embeddings to construct contrastive pairs. By our proposed CGCL, we construct structural neighbor contrastive learning objects, candidate contrastive learning objects, and candidate structural neighbor contrastive learning objects to obtain high-quality node embeddings. To validate the proposed model, we conducted extensive experiments on three publicly available datasets. Compared with various state-of-the-art DNN-, GNN- and GCL-based methods, our proposed CGCL achieved significant improvements in all indicators(1).
更多查看译文
关键词
Recommendation System,Graph Neural Network,Contrastive Learning,Candidate
AI 理解论文
溯源树
样例
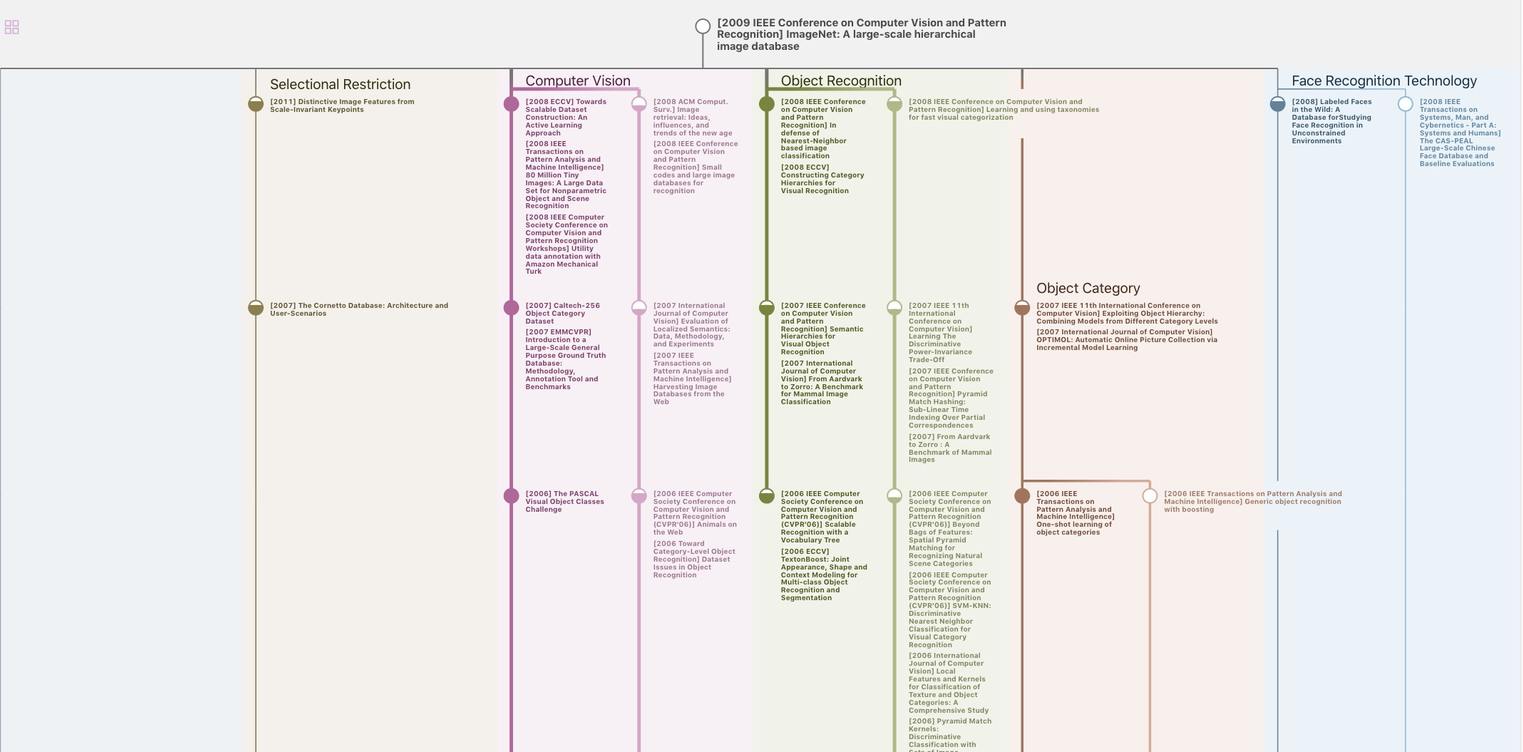
生成溯源树,研究论文发展脉络
Chat Paper
正在生成论文摘要