Improved Vector Quantization For Dense Retrieval with Contrastive Distillation
PROCEEDINGS OF THE 46TH INTERNATIONAL ACM SIGIR CONFERENCE ON RESEARCH AND DEVELOPMENT IN INFORMATION RETRIEVAL, SIGIR 2023(2023)
摘要
Recent work has identified that distillation can be used to create vector quantization based ANN indexes by learning the inverted file index and product quantization. The argued advantage of using a fixed teacher encoder for queries and documents is that the scores produced by the teacher can be used instead of the label judgements that are required when using traditional supervised learning, such as contrastive learning. However, current work only distills the teacher encoder outputs of dot products between quantized query embedddings and product quantized document embeddings. Our work combines the benefits of contrastive learning and distillation by using contrastive distillation whereby the teacher outputs contrastive scores that the student learns from. Our experimental results on MSMARCO passage retrieval and NQ open question answering datasets show that contrastive distillation improves over current state of the art for vector quantized dense retrieval.
更多查看译文
关键词
dense retrieval,contrastive learning,distillation,quantization
AI 理解论文
溯源树
样例
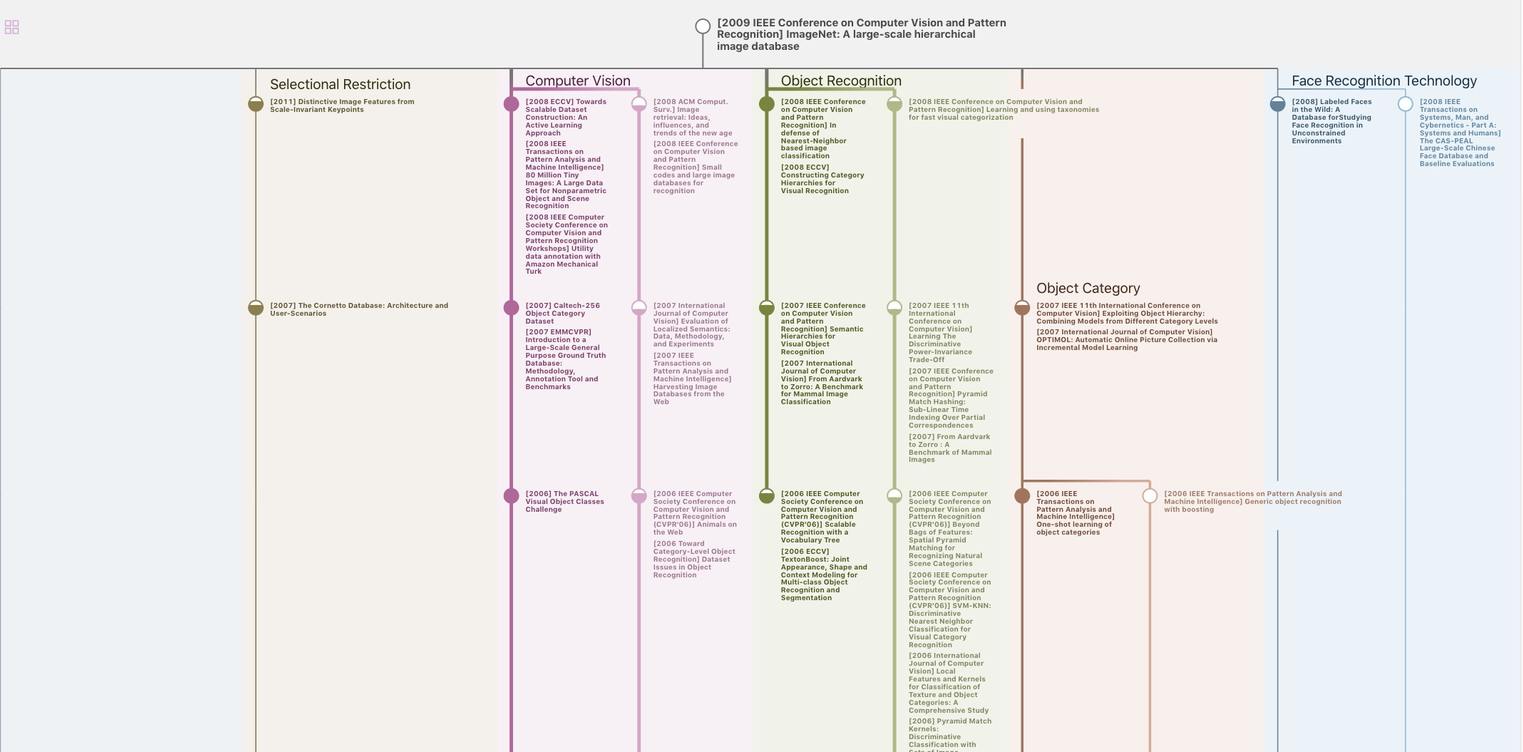
生成溯源树,研究论文发展脉络
Chat Paper
正在生成论文摘要