CMNN-RADC: A Crowdsensing Convolutional-based Mixer Neural Network Road Anomalies Detector and Classifier.
Internet Things(2023)
摘要
The ability of classifying road anomalies plays a crucial role in monitoring road conditions and the maintenance process. Modern techniques utilize the acceleration signal resulting from the interaction between the passing vehicles and the road anomalies to classify their type. Most of the previous methods depended on calculating handcrafted features from only the z acceleration signal to classify road anomalies. However, this does not guarantee that the calculated set of features is sufficient to distinguish between the different anomalies. Therefore, in this paper, we propose an efficient crowdsensing system, called Convolutional-based Mixer Neural Network Road Anomalies Detector and Classifier (CMNN- RADC), for detecting and classifying the road anomalies. CMNN-RADC is based on mixing the low and high frequency automatically extracted features from the three acceleration signals (i.e. x, y, and z) for detecting and classifying road anomalies. A simple two-stages Convolutional-based Mixer Neural Network (CMNN) is designed in the proposed system. The first stage identifies the presence of anomalies, while the second stage distinguishes the identifying anomaly into one of three types (pothole, asphalt bump, or metal bump). This saves the consumption power and computational time of the system. Different experiments were carried out to optimize the classifier parameters and test its performance. The results show the superiority of the CMNN-RADC over the previous methods because it has the ability to detect 99.3% of the anomalies. Moreover, it classifies anomalies with an F1 score of 98.42, 97.68, and 99.05% for metal bump, pothole, and asphalt bump, respectively.
更多查看译文
关键词
Internet of Things,Crowdsensing convolution neural network,Road anomalies
AI 理解论文
溯源树
样例
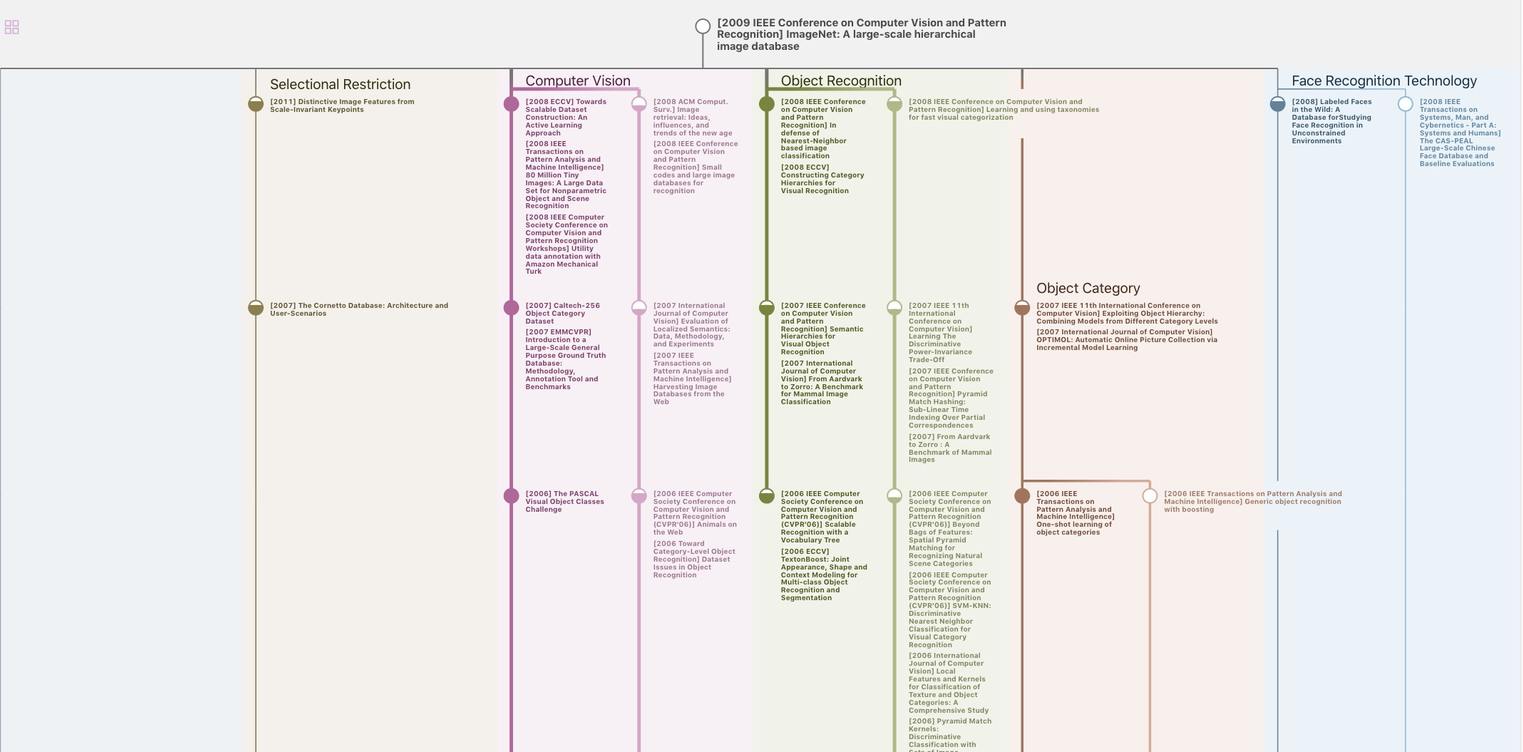
生成溯源树,研究论文发展脉络
Chat Paper
正在生成论文摘要