Quantifying Ranker Coverage of Different Query Subspaces
PROCEEDINGS OF THE 46TH INTERNATIONAL ACM SIGIR CONFERENCE ON RESEARCH AND DEVELOPMENT IN INFORMATION RETRIEVAL, SIGIR 2023(2023)
摘要
The information retrieval community has observed significant performance improvements over various tasks due to the introduction of neural architectures. However, such improvements do not necessarily seem to have happened uniformly across a range of queries. As we will empirically show in this paper, the performance of neural rankers follow a long-tail distribution where there are many subsets of queries, which are not effectively satisfied by neural methods. Despite this observation, performance is often reported using standard retrieval metrics, such as MRR or nDCG, which capture average performance over all queries. As such, it is not clear whether reported improvements are due to incremental boost on a small subset of already well-performing queries or addressing queries that have been difficult to address by existing methods. In this paper, we propose the Task Subspace Coverage (TaSC/tAHsk/) metric, which systematically quantifies whether and to what extent improvements in retrieval effectiveness happen on similar or disparate query subspaces for different rankers. We show that the consideration of our proposed TaSC metric in conjunction with existing ranking metrics provides deeper insight into ranker performance and their contribution to overall advances.
更多查看译文
关键词
Evaluation,Retrieval Effectiveness,Information Retrieval Benchmarks
AI 理解论文
溯源树
样例
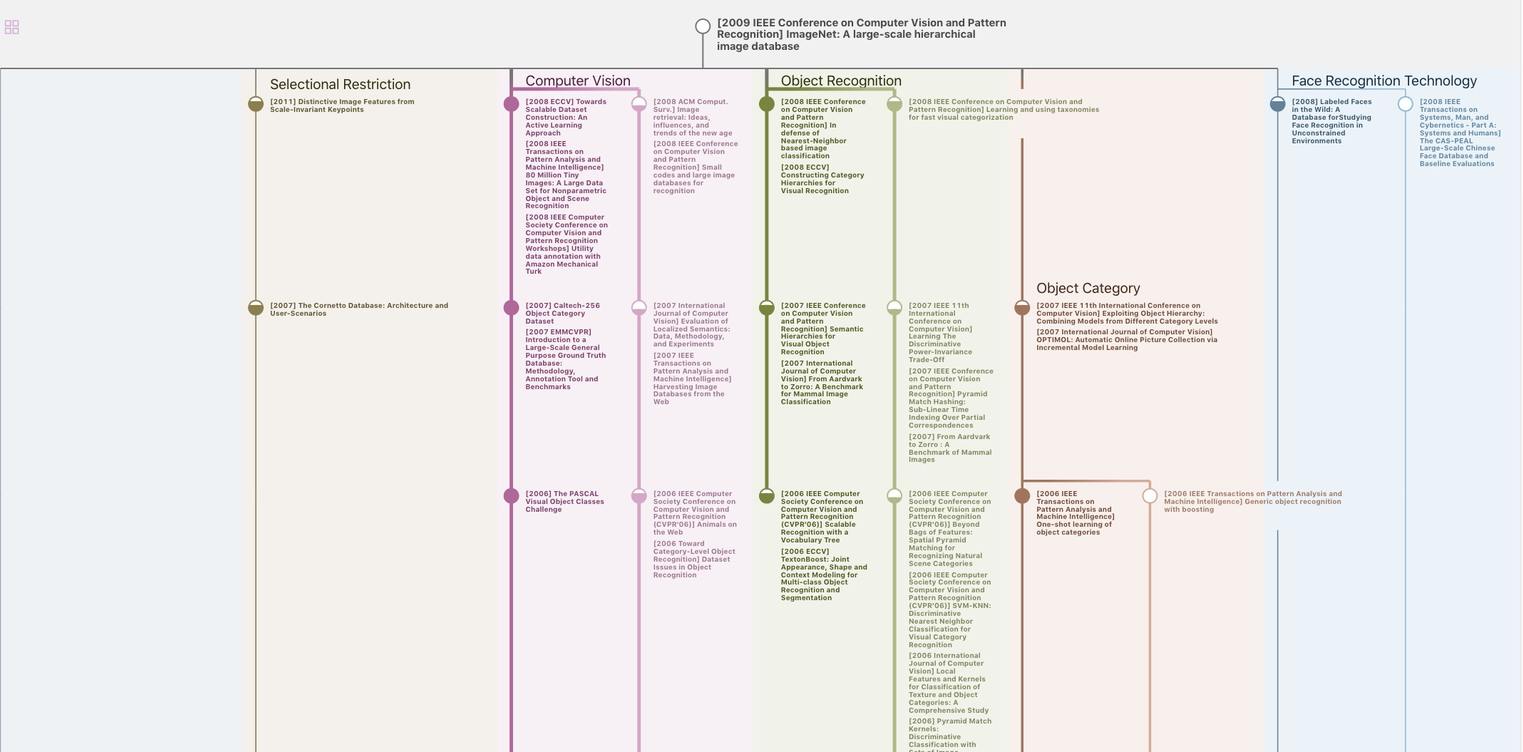
生成溯源树,研究论文发展脉络
Chat Paper
正在生成论文摘要