Removing Influence of MODIS Strip Noise in Spatiotemporal Fusion of Remote Sensing Imagery.
IEEE Geosci. Remote. Sens. Lett.(2023)
摘要
Spatiotemporal fusion (STF) can produce synthetic remote sensing data with both high spatial and high temporal resolution by blending the temporally sparse fine resolution data and temporally dense coarse resolution data, for which it has been adopted in many fields, such as agriculture, environment, and ecology. In the literature, Moderate Resolution Imaging Spectroradiometer (MODIS) images are the most frequently employed as the coarse resolution images in STF due to their high quality and availability. However, the MODIS images contain significant strip noise sometimes, which will lead to unfavorable fusion results. In this letter, a MODIS strip noise removal strategy for STF (NRSTF) is suggested. Specifically, the NRSTF first removes the strip noise of MODIS image of the base time utilizing the bands relationship from the base fine resolution image, then reconstructs that of the predicted time via an adaptive convolution-based noise estimation approach. Finally, the reconstructed, noise-free MODIS images are fused with the base fine resolution image to predict the missing fine resolution images. Four wildly used STF approaches, including spatial and temporal adaptive reflectance fusion method (STARFM), flexible spatiotemporal data fusion (FSDAF) method, Fit-FC, and reliable and adaptive spatiotemporal data fusion (RASDF) are selected to test the NRSTF on an actual Landsat-MODIS dataset, whose MODIS images suffers from serious strip noise in two short wave infrared (SWIR) bands. The experiment results demonstrate that the proposed NRSTF can not only effectively eliminate strip noise, but also significantly enhance the accuracy and applicability of the fusion results. The source code of NRSTF is available at
https://github.com/lijli26/NRSTF.git
.
更多查看译文
关键词
spatiotemporal fusion,remote sensing imagery,remote sensing,modis strip noise
AI 理解论文
溯源树
样例
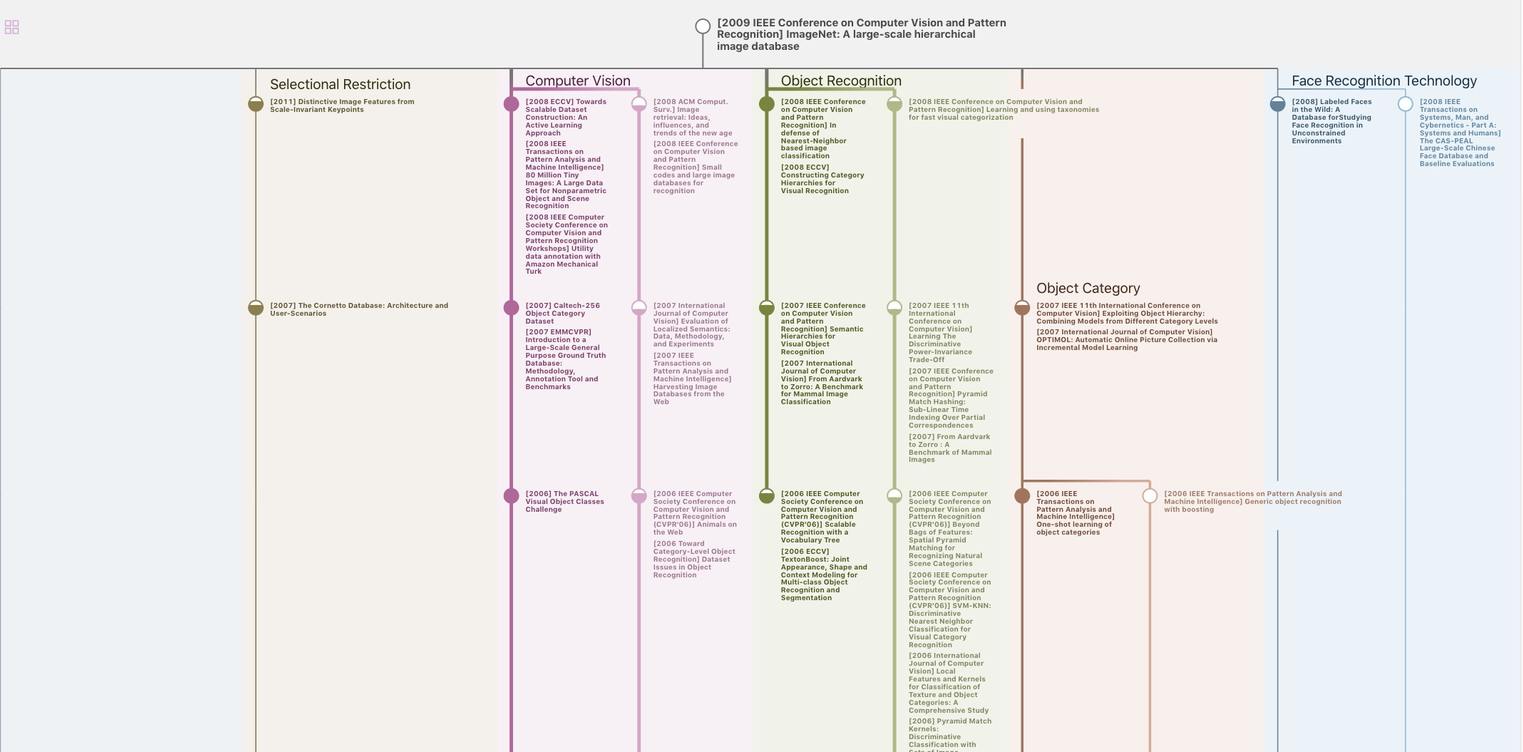
生成溯源树,研究论文发展脉络
Chat Paper
正在生成论文摘要