Lyapunov-Based Long Short-Term Memory (Lb-LSTM) Neural Network-Based Control.
IEEE Control. Syst. Lett.(2023)
摘要
Recurrent neural networks (RNNs) are a dynamic mapping that can capture time-varying, accumulative effects in a sequence that static, feedforward neural networks (NNs) cannot. Long short-term memory (LSTM) NNs are a type of RNN that have gained recent popularity because the cell structure allows them to retain long-term information more efficiently than traditional RNNs. Existing results develop LSTM-based controllers to compensate for uncertainties in nonlinear systems. However, these results use discrete-time LSTMs with offline-trained weights. In this letter, a Lyapunov-based LSTM controller is developed for general Euler-Lagrange systems. Specifically, an Lb-LSTM is implemented in the control design to adaptively estimate uncertain model dynamics, where the weight estimates of the LSTM cell are updated using Lyapunov-based adaptation laws. This allows the LSTM cell to adapt to system uncertainties without requiring offline training. A Lyapunov-based stability analysis yields uniform ultimate boundedness (UUB) of the tracking errors and LSTM state and weight estimation errors. Simulations indicate the developed Lb-LSTM-based controller yielded significant improvement in tracking and function approximation performance when compared to several DNN examples.
更多查看译文
关键词
control,lyapunov-based,short-term,lb-lstm,network-based
AI 理解论文
溯源树
样例
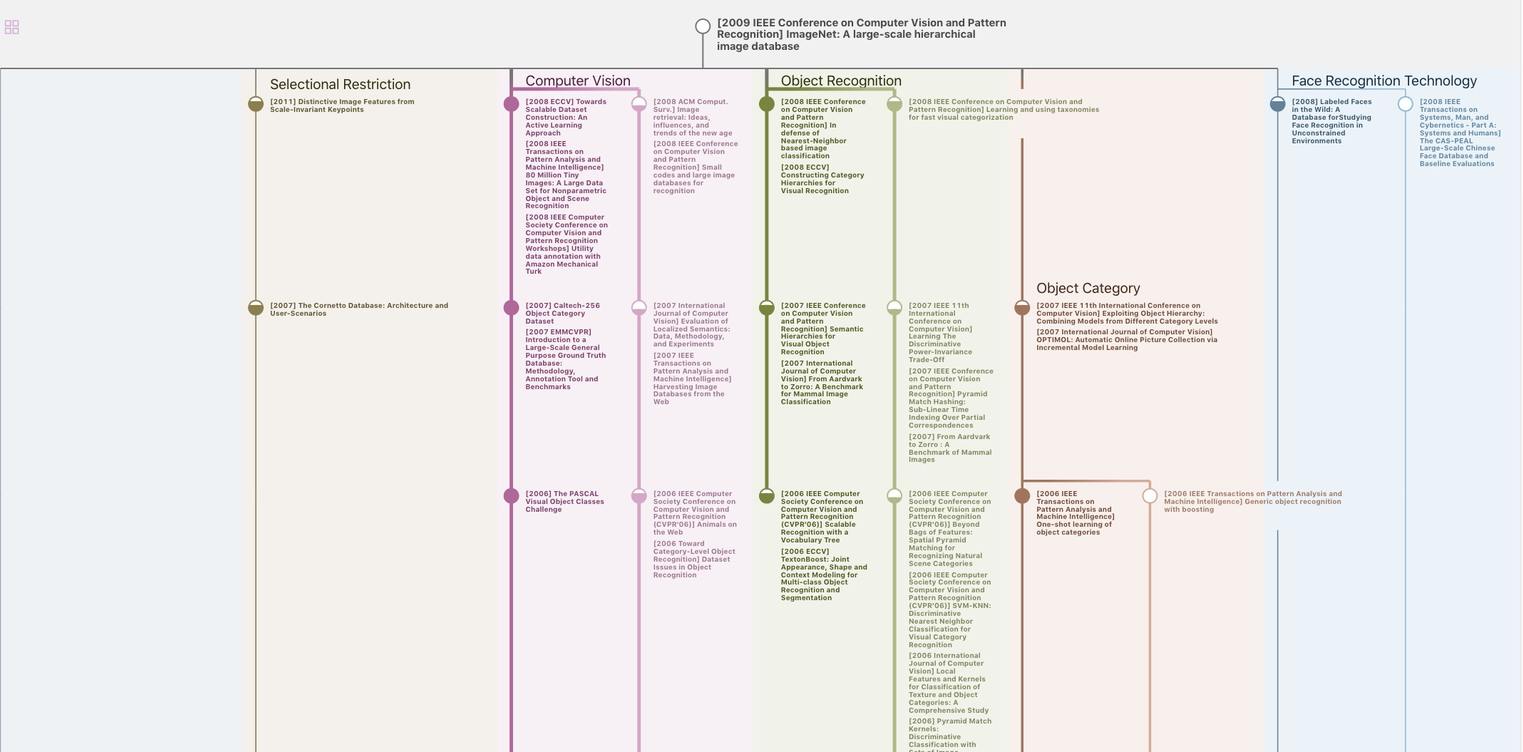
生成溯源树,研究论文发展脉络
Chat Paper
正在生成论文摘要