Deep Learning-Based Symptomizing Cyber Threats Using Adaptive 5G Shared Slice Security Approaches.
Future Internet(2023)
摘要
In fifth Generation (5G) networks, protection from internal attacks, external breaches, violation of confidentiality, and misuse of network vulnerabilities is a challenging task. Various approaches, especially deep-learning (DL) prototypes, have been adopted in order to counter such challenges. For 5G network defense, DL module are recommended here in order to symptomize suspicious NetFlow data. This module behaves as a virtual network function (VNF) and is placed along a 5G network. The DL module as a cyber threat-symptomizing (CTS) unit acts as a virtual security scanner along the 5G network data analytic function (NWDAF) to monitor the network data. When the data were found to be suspicious, causing network bottlenecks and let-downs of end-user services, they were labeled as "Anomalous". For the best proactive and adaptive cyber defense system (PACDS), a logically organized modular approach has been followed to design the DL security module. In the application context, improvements have been made to input features dimension and computational complexity reduction with better response times and accuracy in outlier detection. Moreover, key performance indicators (KPIs) have been proposed for security module placement to secure interslice and intraslice communication channels from any internal or external attacks, also suggesting an adaptive defense mechanism and indicating its placement on a 5G network. Among the chosen DL models, the CNN model behaves as a stable model during behavior analysis in the results. The model classifies botnet-labeled data with 99.74% accuracy and higher precision.
更多查看译文
关键词
threats,learning-based
AI 理解论文
溯源树
样例
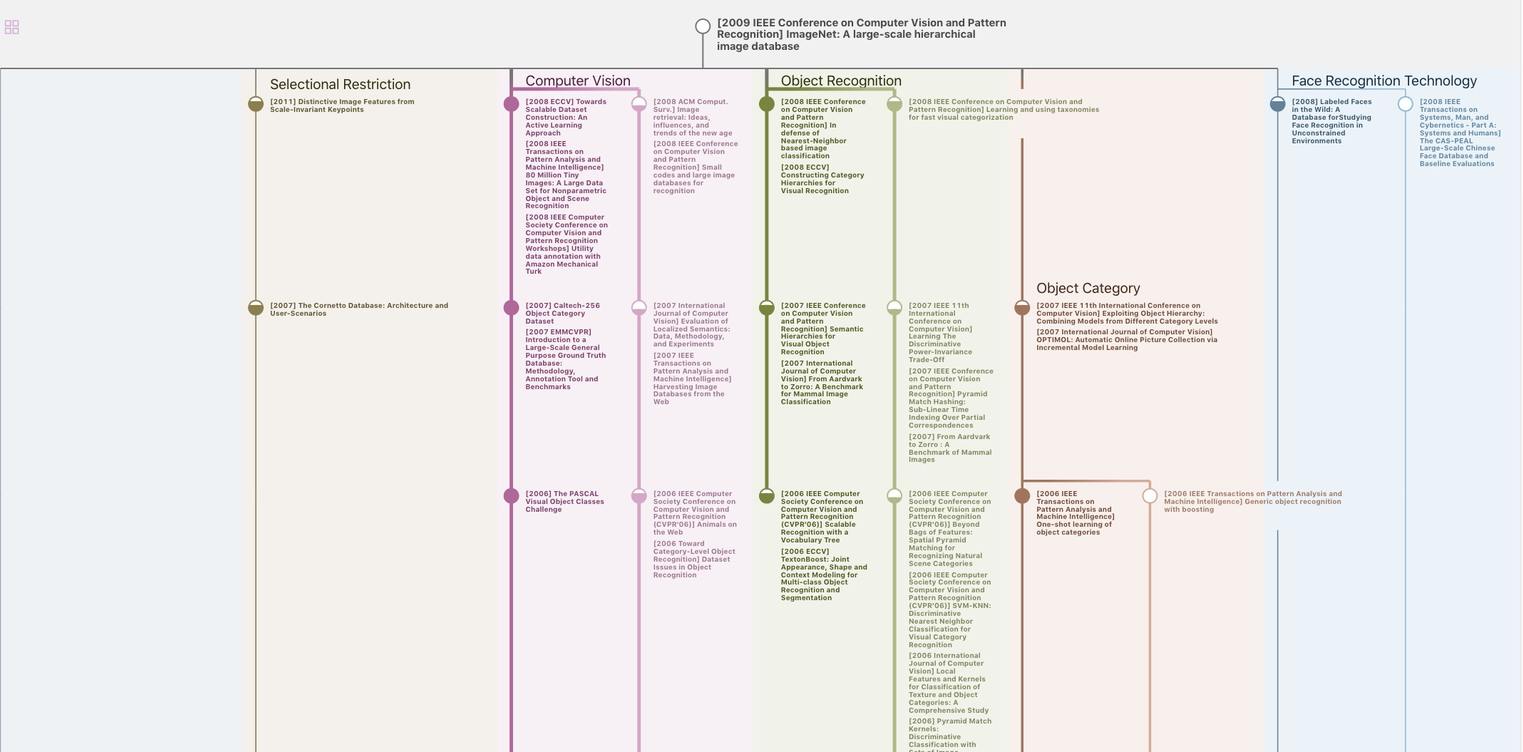
生成溯源树,研究论文发展脉络
Chat Paper
正在生成论文摘要