Online Identification of Cascading Events in Power Systems With Renewable Generation Using Measurement Data and Machine Learning.
IEEE Access(2023)
摘要
This paper introduces a framework for online identification of cascading events in power systems with renewable generation, based on supervised machine learning techniques and measurement data. Cascading events are low-probability, high-impact events, the propagation of which can lead even to large-scale blackouts, with severe consequences to society. The proposed methodology is based on Long-short term memory networks, considering uncertainties associated with renewable generation, system loading and initial contingencies. By utilizing time-series measurement data, the proposed method can predict the appearance of cascading events, as defined by the discrete action of protection devices which can capture voltage, frequency or transient instability related dynamic phenomena. The proposed framework is applied on a modified version of the IEEE-39 bus model incorporating detailed dynamic renewable generation and protection devices implementations. Results highlight that the suggested method can successfully identify cases with cascading events with up to 95.6% accuracy and with an average inference time of 0.042s, taking into account practical considerations related to phasor measurement units, such as availability and noise in measurement data.
更多查看译文
关键词
machine learning,power systems,renewable generation,measurement data
AI 理解论文
溯源树
样例
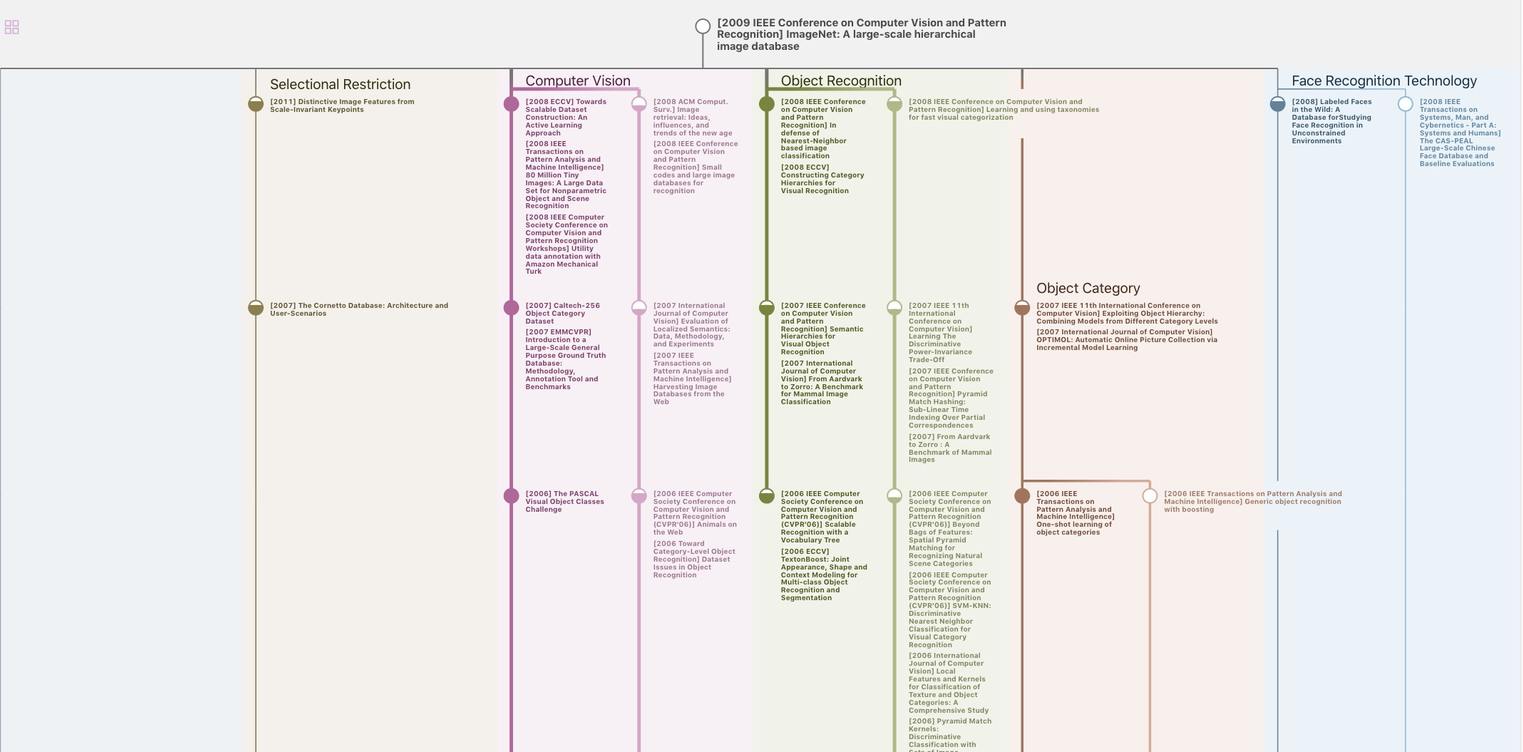
生成溯源树,研究论文发展脉络
Chat Paper
正在生成论文摘要