BERT-PG: a two-branch associative feature gated filtering network for aspect sentiment classification
J. Intell. Inf. Syst.(2023)
摘要
Aspect sentiment classification is an important branch of sentiment classification that has gained increasing attention recently. Existing aspect sentiment classification methods typically use different network branches to encode context and aspect words separately, and then use an attention mechanism to capture their associations. This attention-based approach cannot completely ignore the contexts unrelated to the current aspect words, which brings noise interference. In this paper, a gated filtering network based on BERT is suggested as a solution to this issue. We employ BERT to encode the text semantics of contexts and sentence pairs consisting of context and aspect words respectively, and to extract lexical features as well as associative features of context and aspect words. Based on this, we designed a gating module that, unlike the attention mechanism, uses association features to precisely filter irrelevant contexts. Additionally, because the BERT network parameters are so big, there is a tendency to over-fitting during training. To effectively combat this problem, we developed a loss function with a threshold. We carried out extensive experiments using three benchmark datasets to verify the performance of our proposed model. The experimental results show that the method improves the accuracy by 0.5%, 1.39% and 2.57% on the Laptop, Restaurant and Twitter datasets respectively, and 1.564%, 2.36% and 4.144% on Macro-F1 respectively, compared to the recent RA-CNN (BERT), proving that our method is effective in improving the presentation of aspect sentiment classification in comparison to other cutting-edge sentiment classification methods.
更多查看译文
关键词
aspect,feature,classification,two-branch
AI 理解论文
溯源树
样例
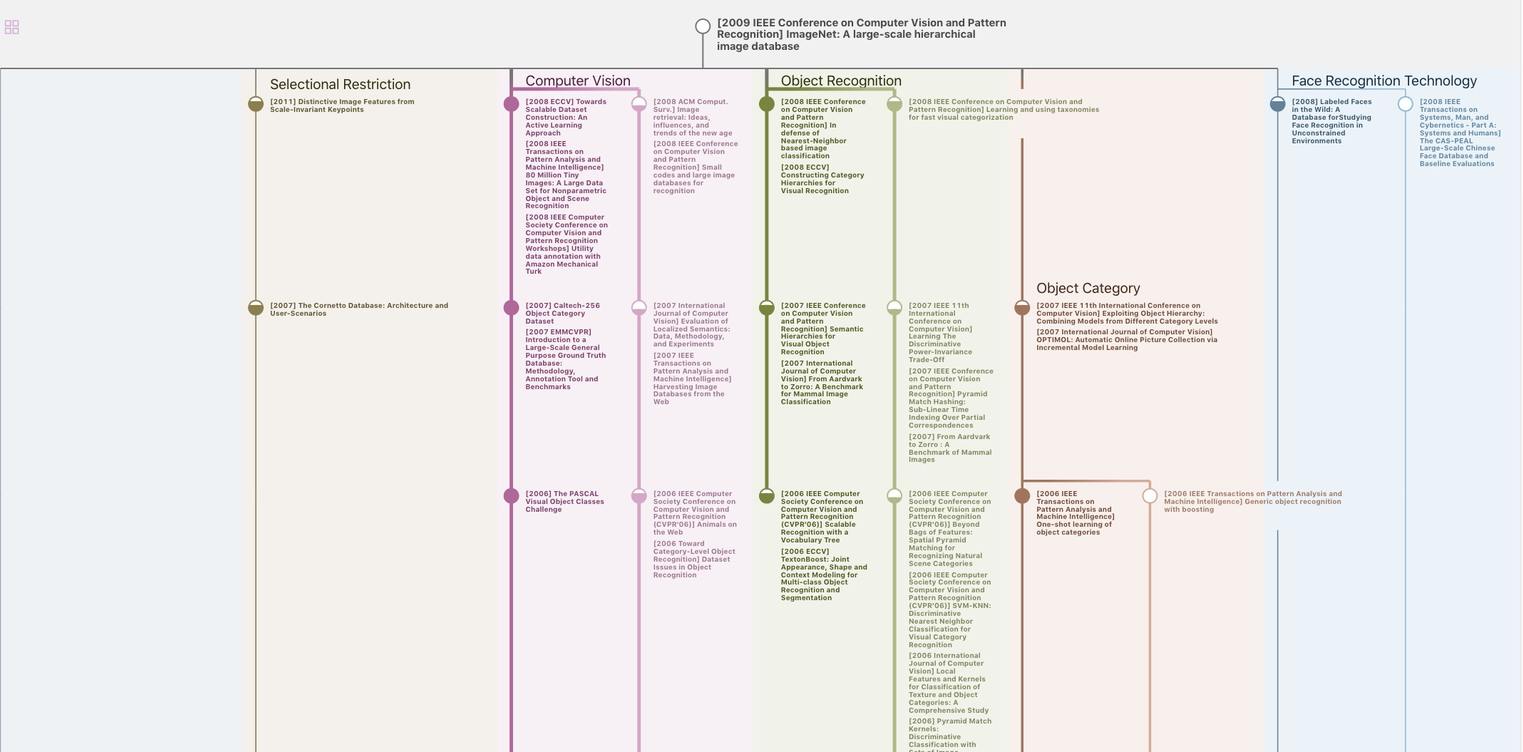
生成溯源树,研究论文发展脉络
Chat Paper
正在生成论文摘要