Development of an Adversarial Transfer Learning-Based Soft Sensor in Industrial Systems.
IEEE Trans. Instrum. Meas.(2023)
摘要
Data-driven soft sensors are usually used to predict quality-related but hard-to-measure variables in industrial systems. The acceptable prediction performance, however, mainly relies on the premise that training data are sufficient for model training. To acquire more training data, this article proposes an adversarial transfer learning (ATL) methodology to enhance soft sensor learning. First, a hierarchical transfer learning algorithm, which integrates a feature extraction method with model-based transfer learning, is proposed to refine the useful hidden information from both historical variables and samples. Second, a novel adversarial learning network is designed to prevent the deterioration of transferred results at each transfer learning stage. Third, a Granger causality analysis (GCA)-based rationale analyzer is added to unfold the internal causality among input variables and between input and output variables simultaneously. Finally, the effectiveness of the proposed soft sensor and the rationale analyzer is validated in a simulated wastewater plant, benchmark simulation model No.2 (BSM2), and a full-scale oxidation ditch (OD) wastewater plant. The experimental results demonstrate that the ATL-based soft sensor can achieve more accurate prediction in terms of root-mean-square error (RMSE) and R, and the GCA-based rationale analyzer can provide a visual explanation for the corresponding model and prediction results.
更多查看译文
关键词
Adversarial transfer learning (ATL),Granger causality analysis (GCA),historical data,industrial systems,soft sensor
AI 理解论文
溯源树
样例
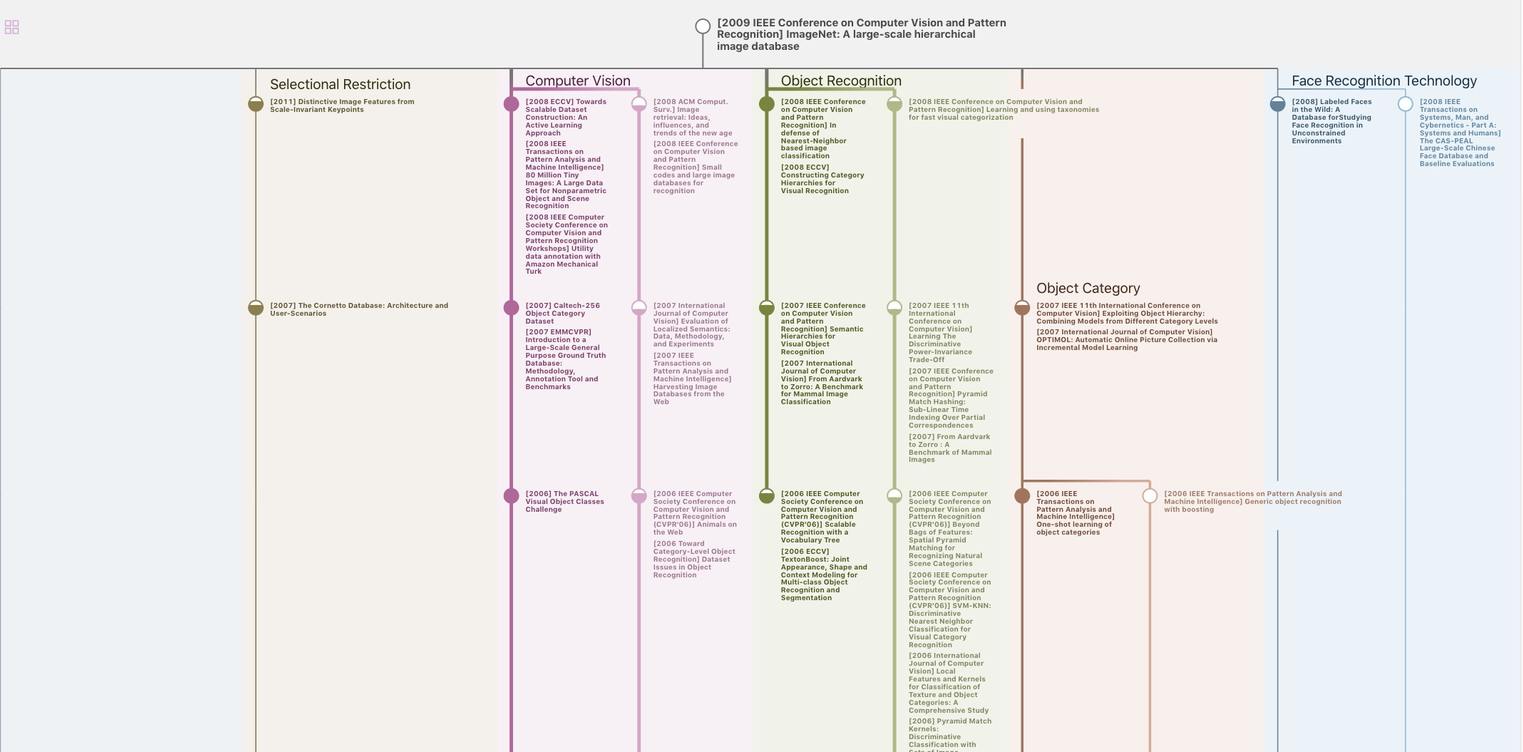
生成溯源树,研究论文发展脉络
Chat Paper
正在生成论文摘要