Semantic Segmentation of Spectral LiDAR Point Clouds Based on Neural Architecture Search.
IEEE Trans. Geosci. Remote. Sens.(2023)
摘要
Multispectral light detection and ranging (LiDAR) point clouds, as a new kind of data, can be characterized by high consistency and integrity of spectral information geometric data. which makes it beneficial for land use classification. However, direct classification of multispectral LiDAR data remains challenging, since the data are irregular and unordered. By describing the point cloud in the form of graph data and using graph convolution to naturally model local geometric structures' representation, higher processing accuracy and efficiency can be achieved. Notably, however, due to the complexity of graph data, using only a single graph convolution to learn the data features may limit the capacity of the architecture; in addition, selecting appropriate graph convolutions requires human expertise and massive numbers of trials. In this article, we propose a network that combines several outstanding graph convolutions by adapting neural architecture search (NAS) for point cloud segmentation, an approach referred to as GCNAS, which can adaptively achieve different levels of semantic expression. In the search module, we use the Monte Carlo tree search (MCTS) method to select the layerwise graph convolution kernels. Experiments on the Titan multispectral LiDAR dataset have verified the effectiveness of GCNAS.
更多查看译文
关键词
Point cloud compression, Laser radar, Convolution, Computer architecture, Three-dimensional displays, Semantics, Feature extraction, Automated machine learning (AutoML), graph convolution, multispectral light detection and ranging (LiDAR), neural architecture search (NAS), semantic segmentation
AI 理解论文
溯源树
样例
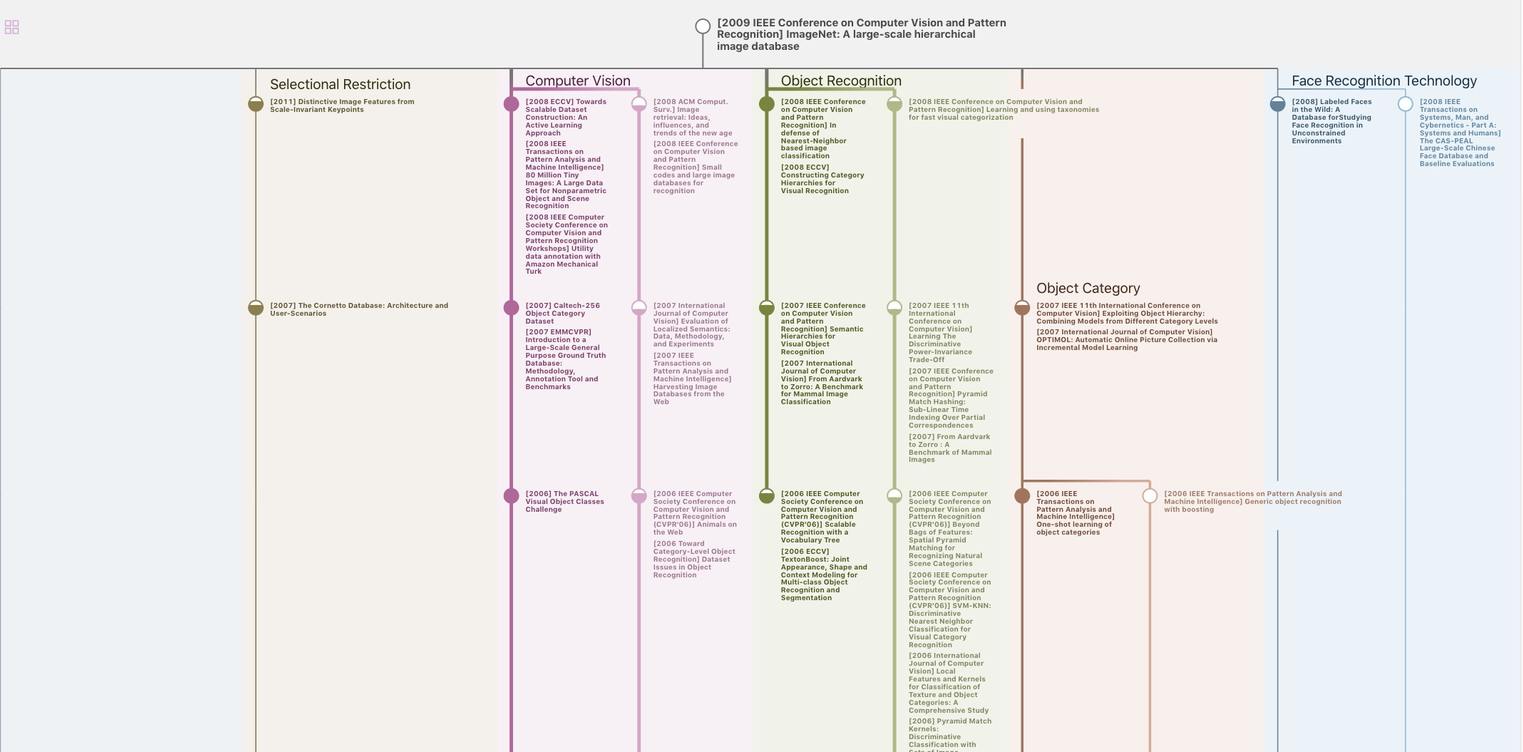
生成溯源树,研究论文发展脉络
Chat Paper
正在生成论文摘要