Physics-Guided Multitask Learning for Estimating Power Generation and CO2 Emissions From Satellite Imagery
IEEE Trans. Geosci. Remote. Sens.(2023)
摘要
Fossil fuel combustion produces large quantities of carbon dioxide (CO2), a major greenhouse gas (GHG), which is one of the main drivers of climate change. A quantitative assessment of GHG emissions is fundamental to predicting climate change effects, enforcing emission regulations, and monitoring pollution trading schemes. Unfortunately, the reporting of GHG emissions is only required in some countries, resulting in insufficient global coverage. At the same time, the transition from fossil fuels to zero carbon to limit climate change is at the heart of several ecological movements, hence the need for quantifying energy production, as well. In this work, we propose an end-to-end method to estimate power generation rates for fossil fuel power plants from satellite images, based on which we approximate GHG (CO2) emission rates. We present a physics-guided multitask deep-learning approach able to simultaneously predict from a single-satellite image of a power plant: 1) the pixel-area covered by plumes; 2) the type of fired fuel; and 3) the power generation rate. To ensure physically realistic predictions from our model we account for environmental conditions and empirical physical constraints. We then convert the predicted power generation rate into estimates for the rate at which CO2 is being emitted, using a fuel-dependent conversion factor. Experimental results show that our multitask learning approach improves the power generation estimation mean absolute error (MAE) by 23% compared to a single-task network trained on the same dataset.
更多查看译文
关键词
estimating power generation,multitask learning,emissions,physics-guided
AI 理解论文
溯源树
样例
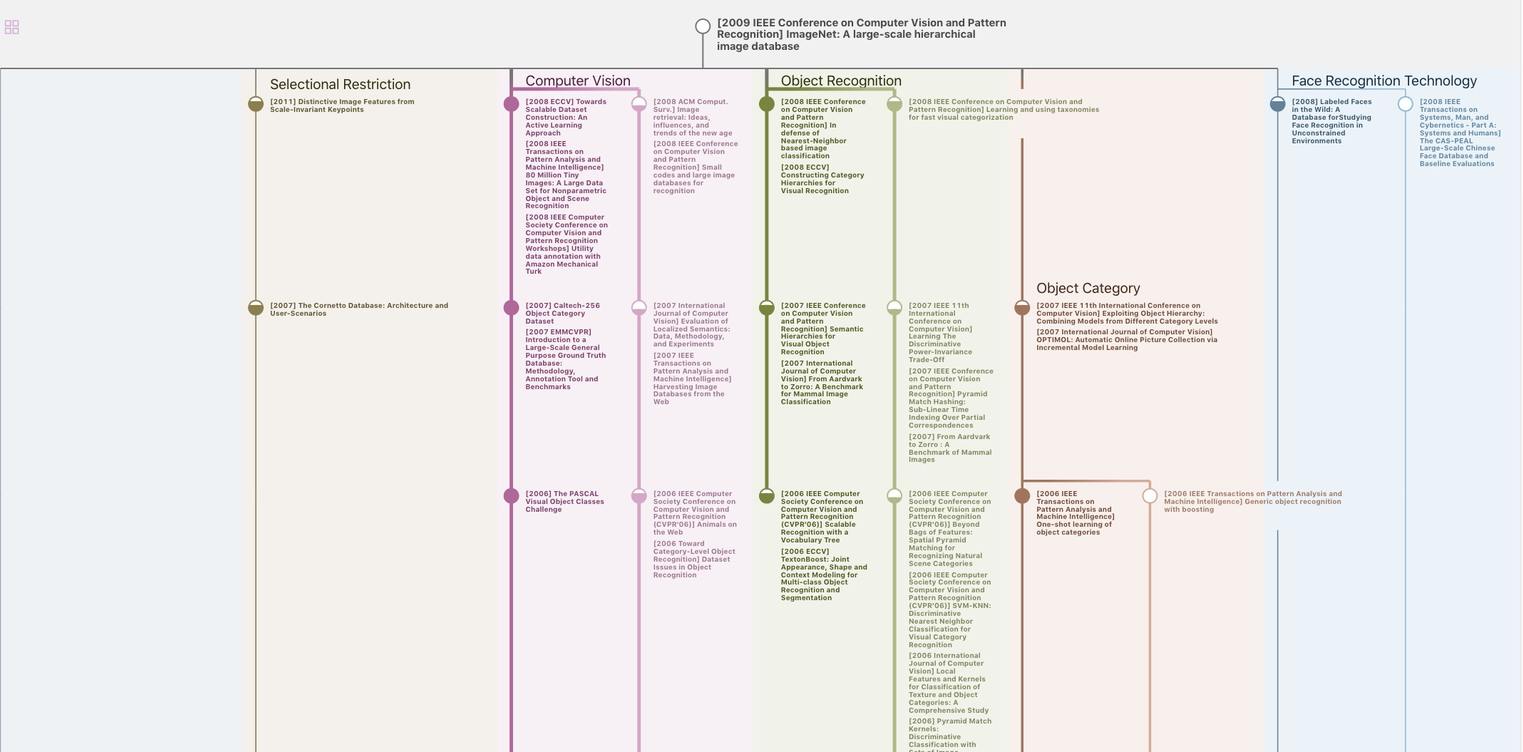
生成溯源树,研究论文发展脉络
Chat Paper
正在生成论文摘要