Physics-Driven Probabilistic Deep Learning for the Inversion of Physical Models With Application to Phenological Parameter Retrieval From Satellite Times Series.
IEEE Trans. Geosci. Remote. Sens.(2023)
摘要
Recent Sentinel satellite constellations and deep learning methods offer great possibilities for estimating the states and dynamics of physical parameters on a global scale. Such parameters and their corresponding uncertainties can be retrieved by machine learning methods solving probabilistic inverse problems. Nevertheless, the scarcity of reference data to train supervised methodologies is a well-known constraint for remote sensing applications. To address such limitations, this work presents a new generic physics-guided probabilistic deep learning methodology to invert physical models. The presented methodology proposes a new strategy to combine probabilistic deep learning methods and physical models avoiding simulation-driven machine learning. The inverse problem is addressed through a Bayesian inference framework by proposing a new physically constrained self-supervised representation learning methodology. To show interest in the proposed strategy, the methodology is applied to the retrieval of phenological parameters from normalized difference vegetation index (NDVI) time series. As a result, the probability distributions of the intrinsic phenological model parameters are inferred. The feasibility of the method is evaluated on both simulated and real Sentinel-2 data and compared with different standard algorithms. Promising results show satisfactory accuracy predictions and low inference times for real applications.
更多查看译文
关键词
Autoencoders (AEs),Bayesian physics-guided learning,generative models,inverse problems,large scale,phenology monitoring,satellite image time series (SITS),self-supervised representation learning
AI 理解论文
溯源树
样例
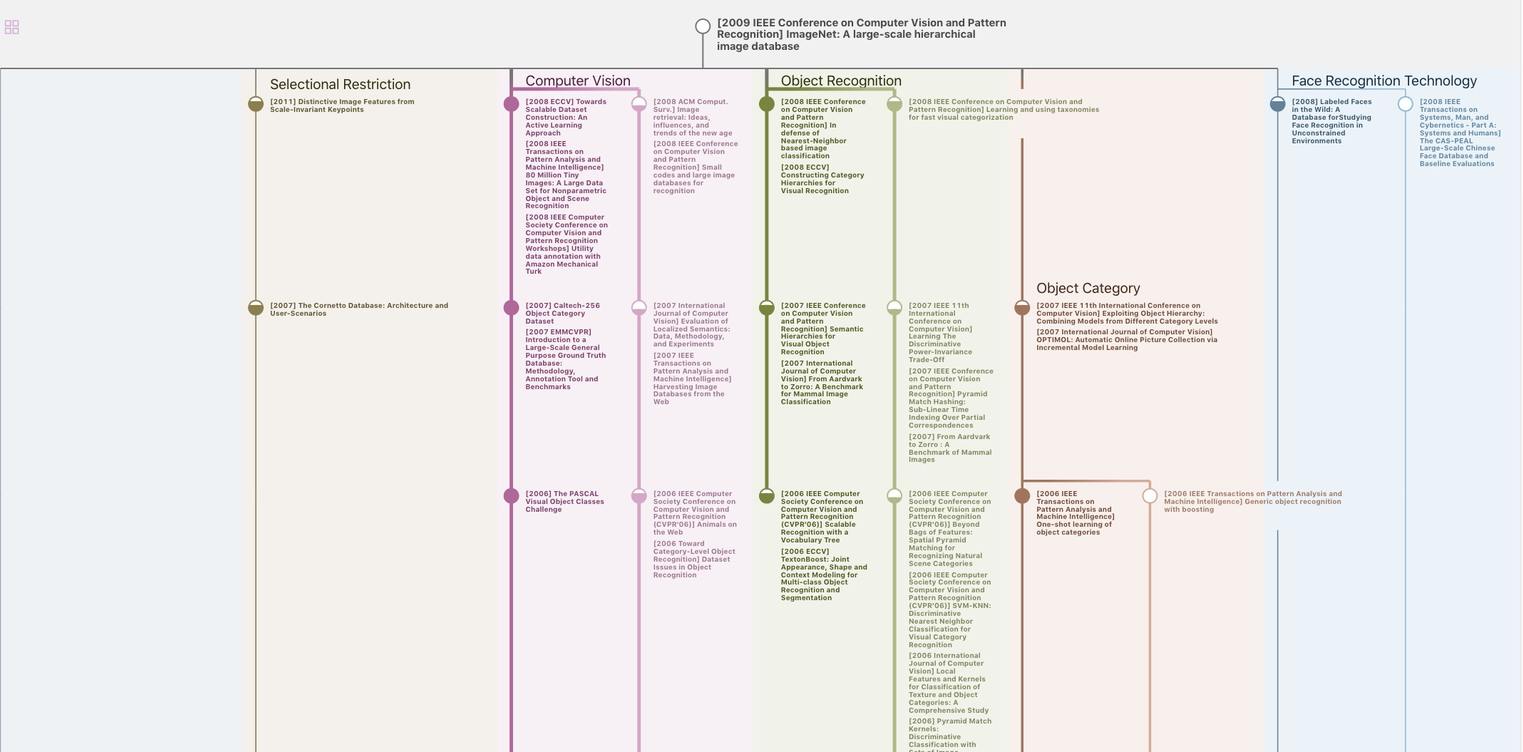
生成溯源树,研究论文发展脉络
Chat Paper
正在生成论文摘要