Hierarchical Manta Ray Foraging Optimization with Weighted Fitness-Distance Balance Selection
Int. J. Comput. Intell. Syst.(2023)
摘要
Manta ray foraging optimization (MRFO) tends to get trapped in local optima as it relies on the direction provided by the previous individual and the best individual as guidance to search for the optimal solution. As enriching population diversity can effectively solve this problem, in this paper, we introduce a hierarchical structure and weighted fitness-distance balance selection to improve the population diversity of the algorithm. The hierarchical structure allows individuals in different groups of the population to search for optimal solutions in different places, expanding the diversity of solutions. In MRFO, greedy selection based solely on fitness can lead to local solutions. We innovatively incorporate a distance metric into the selection strategy to increase selection diversity and find better solutions. A hierarchical manta ray foraging optimization with weighted fitness-distance balance selection (HMRFO) is proposed. Experimental results on IEEE Congress on Evolutionary Computation 2017 (CEC2017) functions show the effectiveness of the proposed method compared to seven competitive algorithms, and the proposed method has little effect on the algorithm complexity of MRFO. The application of HMRFO to optimize real-world problems with large dimensions has also obtained good results, and the computational time is very short, making it a powerful alternative for very high-dimensional problems. Finally, the effectiveness of this method is further verified by analyzing the population diversity of HMRFO.
更多查看译文
关键词
optimization,selection,fitness-distance
AI 理解论文
溯源树
样例
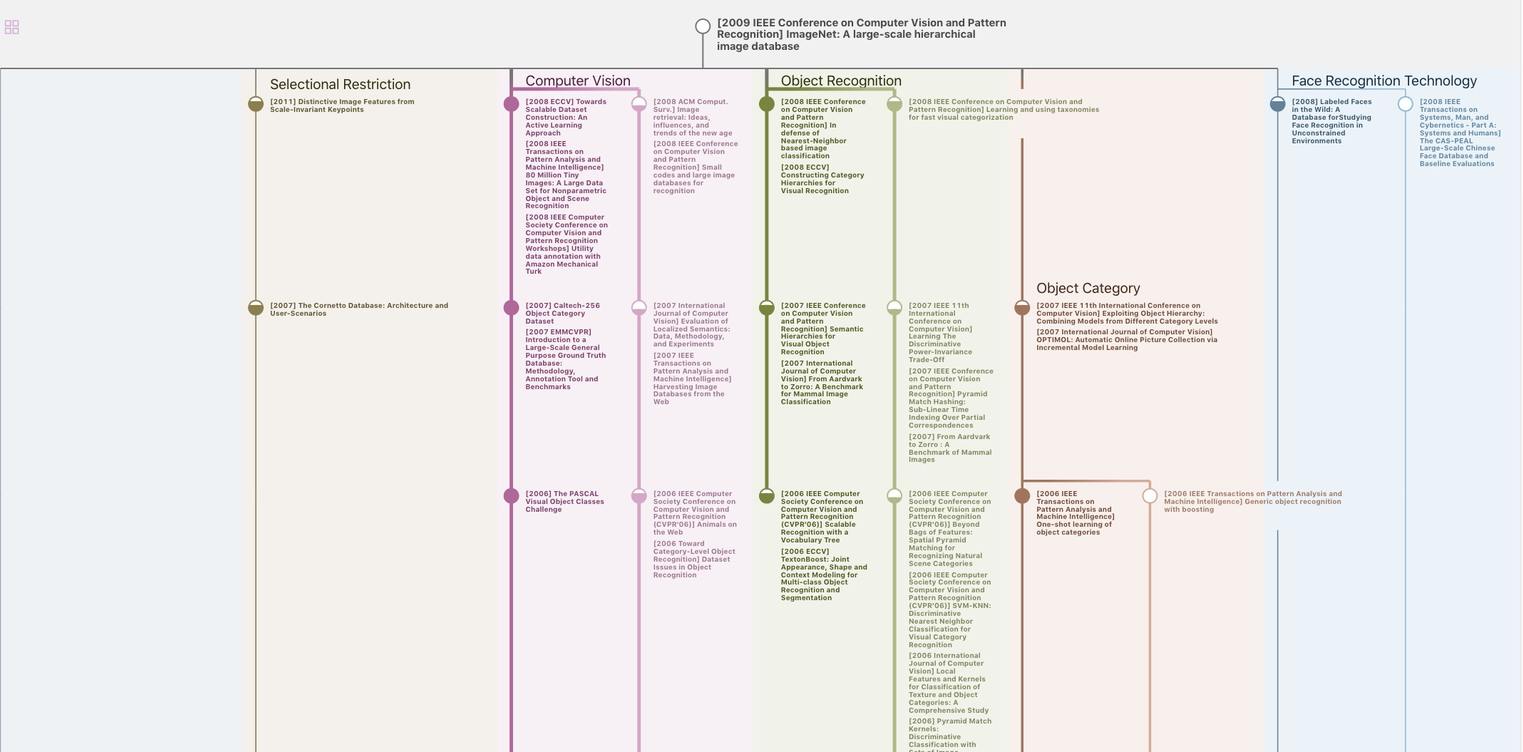
生成溯源树,研究论文发展脉络
Chat Paper
正在生成论文摘要