Topological Similarity and Centrality Driven Hybrid Deep Learning for Temporal Link Prediction
J. Univers. Comput. Sci.(2023)
摘要
Several real-world phenomena, including social, communication, transportation, and biological networks, can be efficiently expressed as graphs. This enables the deployment of graph algorithms to infer information from such complex network interactions to enhance graph applica-tions' accuracy, including link prediction, node classification, and clustering. However, the large size and complexity of the network data limit the efficiency of the learning algorithms in making decisions from such graph datasets. To overcome these limitations, graph embedding techniques are usually adopted. However, many studies not only assume static networks but also pay less attention to preserving the network topological and centrality information, which information is key in analyzing networks. In order to fill these gaps, we propose a novel end-to-end unified Topological Similarity and Centrality driven Hybrid Deep Learning model for Temporal Link Prediction (TSC-TLP). First, we extract topological similarity and centrality-based features from the raw networks. Next, we systematically aggregate these topological and centrality features to act as inputs for the encoder. In addition, we leverage the long short-term memory (LSTM) layer to learn the underlying temporal information in the graph snapshots. Lastly, we impose topological similarity and centrality constraints on the model learning to enforce preserving of topological structure and node centrality role of the input graphs in the learned embeddings. The proposed TSC-TLP is tested on 3 real-world temporal social networks. On average, it exhibits a 4% improvement in link prediction accuracy and a 37% reduction in MSE on centrality prediction over the best benchmark.
更多查看译文
关键词
Topological similarity,centrality,embedding learning,link prediction,deep geometric learning
AI 理解论文
溯源树
样例
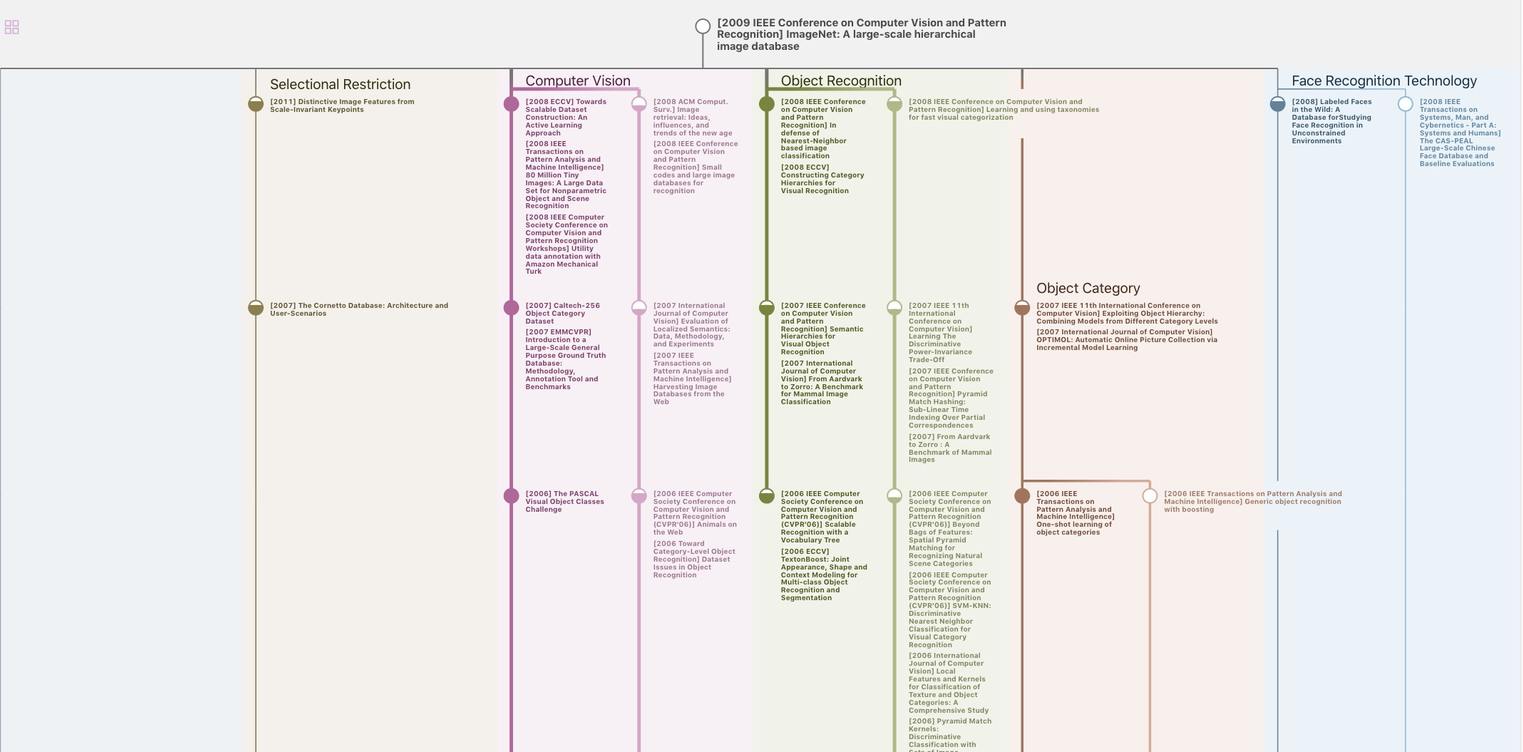
生成溯源树,研究论文发展脉络
Chat Paper
正在生成论文摘要