An Empirical Study of Large-Scale Data-Driven Full Waveform Inversion
arxiv(2023)
摘要
This paper investigates the impact of big data on deep learning models to
help solve the full waveform inversion (FWI) problem. While it is well known
that big data can boost the performance of deep learning models in many tasks,
its effectiveness has not been validated for FWI. To address this gap, we
present an empirical study that investigates how deep learning models in FWI
behave when trained on OpenFWI, a collection of large-scale, multi-structural,
synthetic datasets published recently. In particular, we train and evaluate the
FWI models on a combination of 10 2D subsets in OpenFWI that contain 470K pairs
of seismic data and velocity maps in total. Our experiments demonstrate that
training on the combined dataset yields an average improvement of 13.03
MAE, 7.19
average improvement of 28.60
generalization test. We further demonstrate that model capacity needs to scale
in accordance with data size for optimal improvement, where our largest model
yields an average improvement of 20.06
smallest one.
更多查看译文
AI 理解论文
溯源树
样例
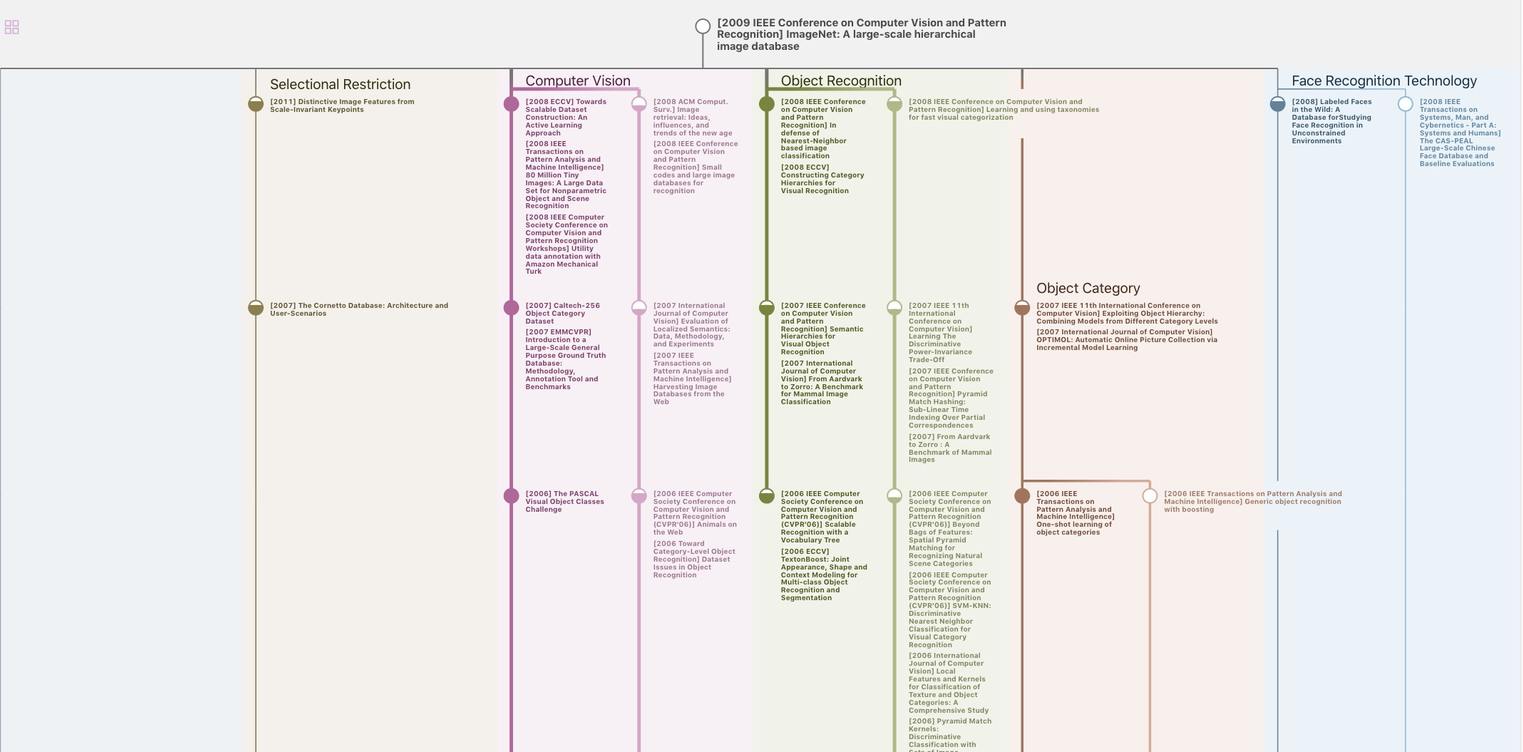
生成溯源树,研究论文发展脉络
Chat Paper
正在生成论文摘要