Information Gained Subgroup Discovery in Datasets
arxiv(2023)
摘要
Lung cancer is the leading cause of cancer death. More than 238,340 new cases of lung cancer patients are expected in 2023, with an estimation of more than 127,070 deaths. Choosing the correct treatment is an important element to enhance the probability of survival and to improve patient's quality of life. Cancer treatments might provoke secondary effects. These toxicities cause different health problems that impact the patient's quality of life. Hence, reducing treatments toxicities while maintaining or improving their effectivenes is an important goal that aims to be pursued from the clinical perspective. On the other hand, clinical guidelines include general knowledge about cancer treatment recommendations to assist clinicians. Although they provide treatment recommendations based on cancer disease aspects and individual patient features, a statistical analysis taking into account treatment outcomes is not provided here. Therefore, the comparison between clinical guidelines with treatment patterns found in clinical data, would allow to validate the patterns found, as well as discovering alternative treatment patterns. In this work, we present Information Gained Subgroup Discovery, a Subgroup Discovery algorithm that aims to find most relevant patterns taking into account Information gain and Odds ratio. Thus, we analyze a dataset containing lung cancer patients information including patients' data, prescribed treatments and their outcomes. Obtained results are validated through clinicians and compared with clinical guidelines. We conclude that this new algorithm achieves highest acceptance of found patterns in this dataset, while also improving indices of Subgroup Discovery.
更多查看译文
AI 理解论文
溯源树
样例
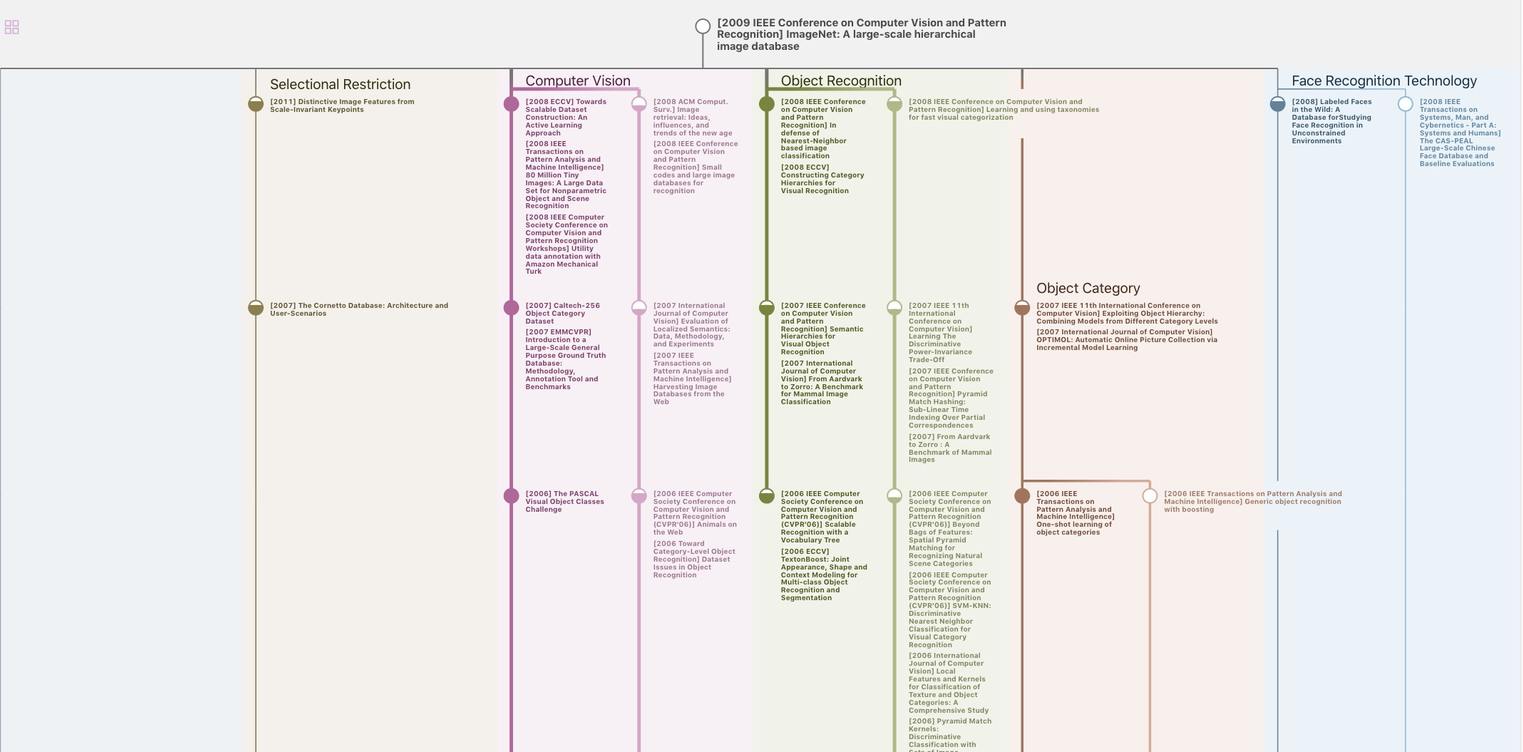
生成溯源树,研究论文发展脉络
Chat Paper
正在生成论文摘要