Deep learning-based automatic detection for pulmonary nodules on chest radiographs: The relationship with background lung condition, nodule characteristics, and location.
European journal of radiology(2023)
摘要
PURPOSE:Computer-aided diagnosis (CAD), which assists in the interpretation of chest radiographs, is becoming common. However, few studies have evaluated the benefits and pitfalls of CAD in the real world. This study aimed to evaluate the independent performance of commercially available deep learning-based automatic detection (DLAD) software, EIRL Chest X-ray Lung Nodule, in a cohort that included patients with background pulmonary abnormalities often encountered in clinical situations.
METHODS:Patients with clinically suspected lung cancer for whom chest radiography was performed within a month before or after CT scan between June 2020 and May 2022 in our institution were enrolled. The reference standard was created using a bounding box annotated by two radiologists with reference to the CT. The visibility score, characteristics, location of the pulmonary nodules, presence of overlapping structures or pulmonary disease, and background lung score were manually determined.
RESULTS:We included 388 patients. The DLAD software detected 222 of the 322 nodules visible on manual evaluation, with a sensitivity of 0.689 and a false-positive rate of 0.168. The detectability of the DLAD software was significantly lower for small and subsolid and nodules with overlapping structures. The visibility score and sensitivity of detection by the DLAD software were positively correlated. The relationship between the background lung score and detection by the DLAD software was unclear.
CONCLUSION:The standalone performance of DLAD in detecting pulmonary nodules exhibited a sensitivity of 0.689 and a false-positive rate of 0.168. Understanding the characteristics of DLAD is crucial when interpreting chest radiographs with the assistance of the DLAD.
更多查看译文
AI 理解论文
溯源树
样例
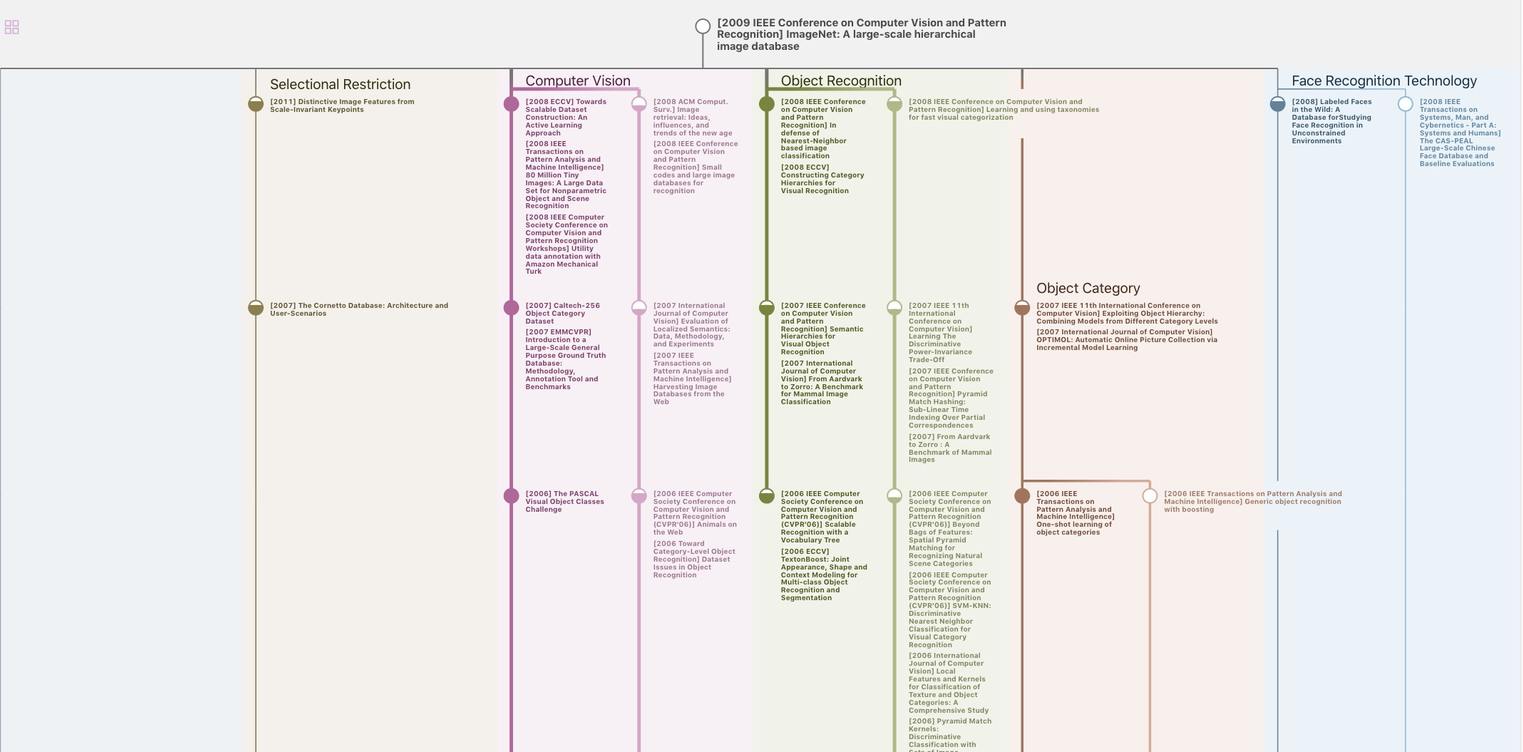
生成溯源树,研究论文发展脉络
Chat Paper
正在生成论文摘要