Sample Less, Learn More: Efficient Action Recognition via Frame Feature Restoration
CoRR(2023)
摘要
Training an effective video action recognition model poses significant computational challenges, particularly under limited resource budgets. Current methods primarily aim to either reduce model size or utilize pre-trained models, limiting their adaptability to various backbone architectures. This paper investigates the issue of over-sampled frames, a prevalent problem in many approaches yet it has received relatively little attention. Despite the use of fewer frames being a potential solution, this approach often results in a substantial decline in performance. To address this issue, we propose a novel method to restore the intermediate features for two sparsely sampled and adjacent video frames. This feature restoration technique brings a negligible increase in computational requirements compared to resource-intensive image encoders, such as ViT. To evaluate the effectiveness of our method, we conduct extensive experiments on four public datasets, including Kinetics-400, ActivityNet, UCF-101, and HMDB-51. With the integration of our method, the efficiency of three commonly used baselines has been improved by over 50%, with a mere 0.5% reduction in recognition accuracy. In addition, our method also surprisingly helps improve the generalization ability of the models under zero-shot settings.
更多查看译文
关键词
efficient action recognition,frame feature
AI 理解论文
溯源树
样例
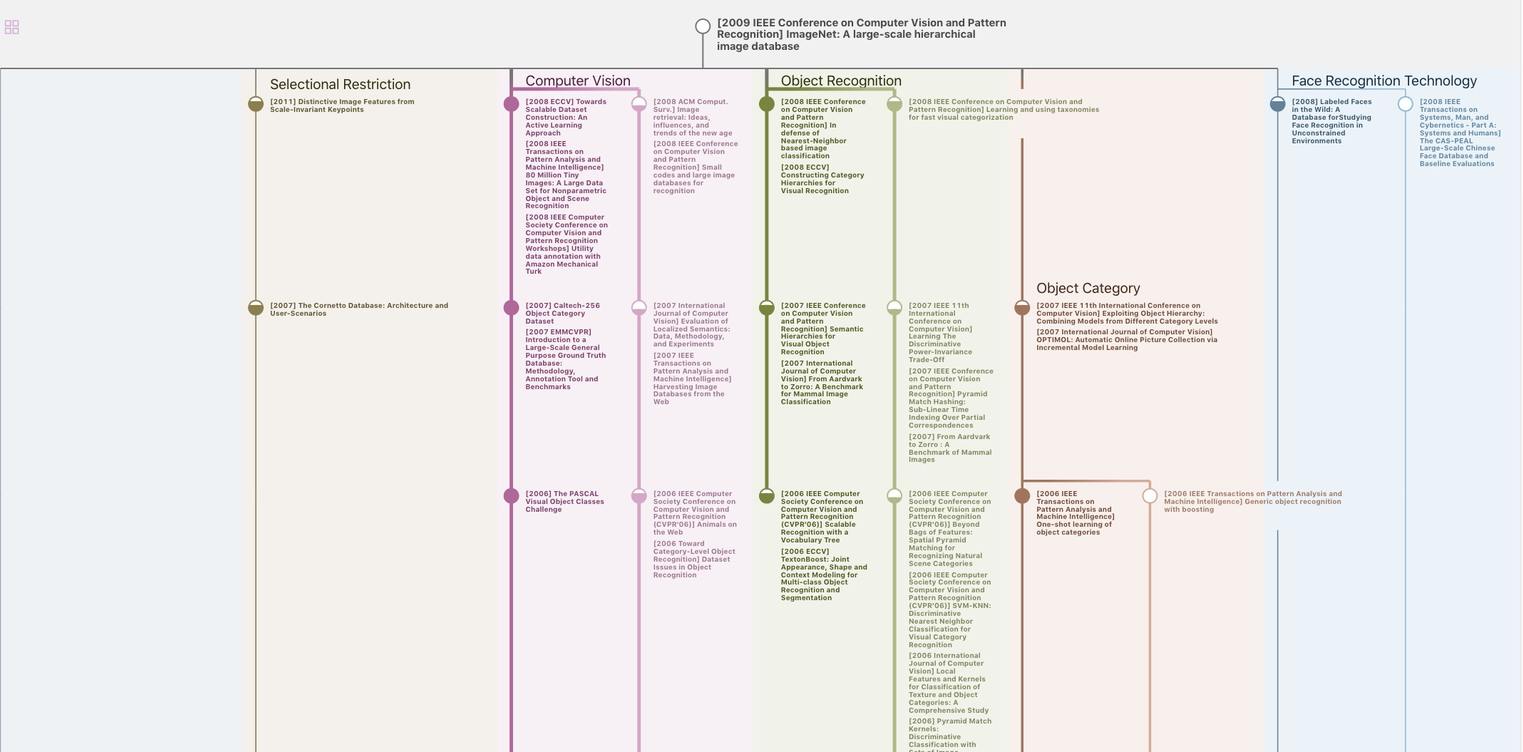
生成溯源树,研究论文发展脉络
Chat Paper
正在生成论文摘要