pCTFusion : Point Convolution-Transformer Fusion with Semantic Aware Loss for Outdoor LiDAR Point Cloud Segmentation
SN Computer Science(2024)
摘要
LiDAR-generated point clouds are crucial for perceiving outdoor environments. The segmentation of point clouds is also essential for many applications. Previous research has focused on using self-attention and convolution (local attention) mechanisms individually in semantic segmentation architectures. However, there is limited work on combining the learned representations of these attention mechanisms to improve performance. Additionally, existing research that combines convolution with self-attention relies on global attention, which is not practical for processing large point clouds. To address these challenges, this study proposes a new architecture, pCTFusion , which combines kernel-based convolutions and self-attention mechanisms for better feature learning and capturing local and global dependencies in segmentation. The proposed architecture employs two types of self-attention mechanisms, local and global, based on the hierarchical positions of the encoder blocks. Furthermore, the existing loss functions do not consider the semantic and position-wise importance of the points, resulting in reduced accuracy, particularly at sharp class boundaries. To overcome this, the study models a novel attention-based loss function called Pointwise Geometric Anisotropy (PGA), which assigns weights based on the semantic distribution of points in a neighborhood. The proposed architecture is evaluated on SemanticKITTI outdoor dataset and showed a 4–5% improvement in performance compared to the state-of-the-art point-based architectures. The results are particularly encouraging for minor classes, often misclassified due to class imbalance, lack of space, and neighbor-aware feature encoding. These developed methods can be leveraged for the segmentation of complex datasets and can drive real-world applications of LiDAR point cloud.
更多查看译文
关键词
Deep learning,LiDAR,Point cloud,Semantic segmentation,Attention mechanisms
AI 理解论文
溯源树
样例
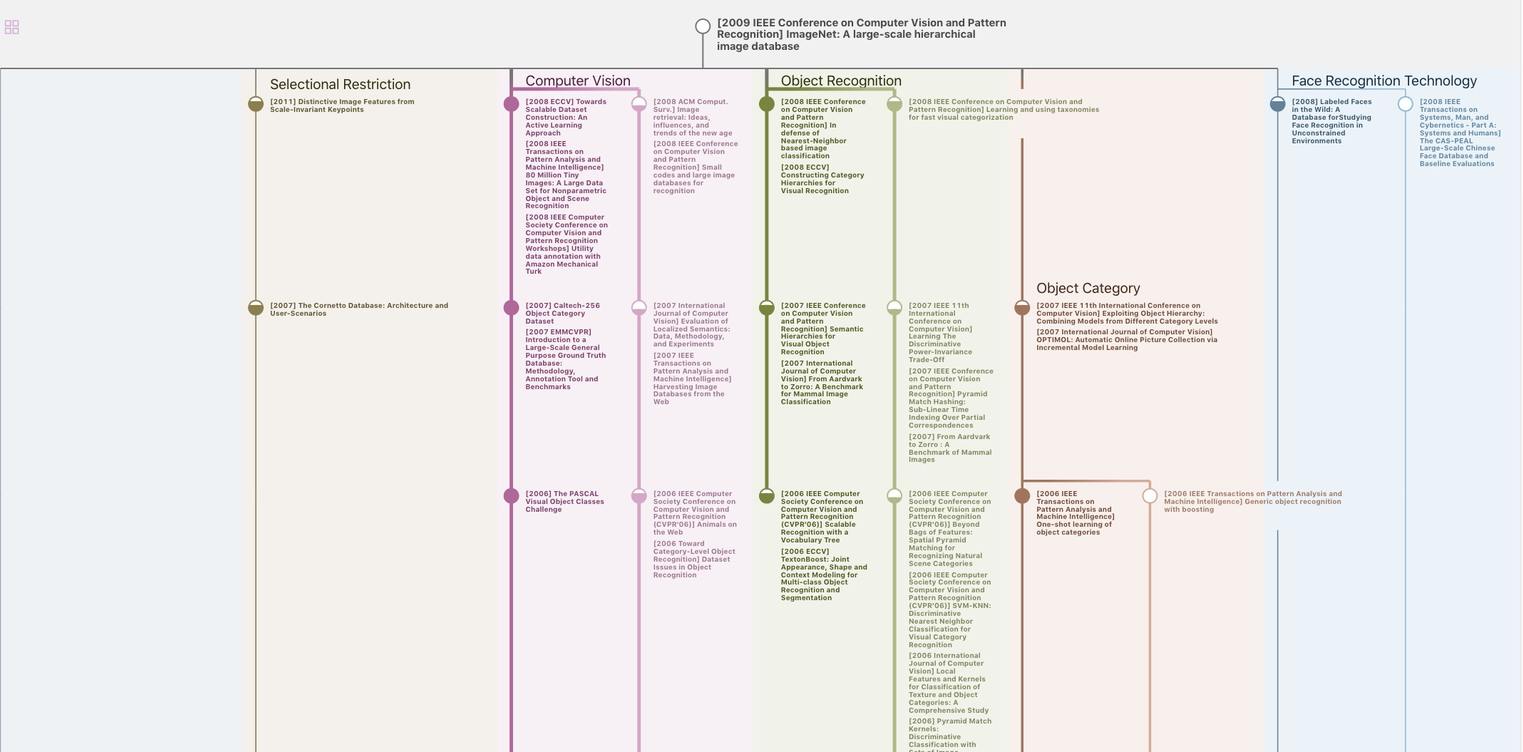
生成溯源树,研究论文发展脉络
Chat Paper
正在生成论文摘要