Hybrid representation-enhanced sampling for Bayesian active learning in musculoskeletal segmentation of lower extremities
International Journal of Computer Assisted Radiology and Surgery(2024)
摘要
Purpose Manual annotations for training deep learning models in auto-segmentation are time-intensive. This study introduces a hybrid representation-enhanced sampling strategy that integrates both density and diversity criteria within an uncertainty-based Bayesian active learning (BAL) framework to reduce annotation efforts by selecting the most informative training samples. Methods The experiments are performed on two lower extremity datasets of MRI and CT images, focusing on the segmentation of the femur, pelvis, sacrum, quadriceps femoris, hamstrings, adductors, sartorius, and iliopsoas, utilizing a U-net-based BAL framework. Our method selects uncertain samples with high density and diversity for manual revision, optimizing for maximal similarity to unlabeled instances and minimal similarity to existing training data. We assess the accuracy and efficiency using dice and a proposed metric called reduced annotation cost (RAC), respectively. We further evaluate the impact of various acquisition rules on BAL performance and design an ablation study for effectiveness estimation. Results In MRI and CT datasets, our method was superior or comparable to existing ones, achieving a 0.8% dice and 1.0% RAC increase in CT (statistically significant), and a 0.8% dice and 1.1% RAC increase in MRI (not statistically significant) in volume-wise acquisition. Our ablation study indicates that combining density and diversity criteria enhances the efficiency of BAL in musculoskeletal segmentation compared to using either criterion alone. Conclusion Our sampling method is proven efficient in reducing annotation costs in image segmentation tasks. The combination of the proposed method and our BAL framework provides a semi-automatic way for efficient annotation of medical image datasets.
更多查看译文
关键词
Active learning,Bayesian deep learning,Image segmentation,Bayesian Uncertainty
AI 理解论文
溯源树
样例
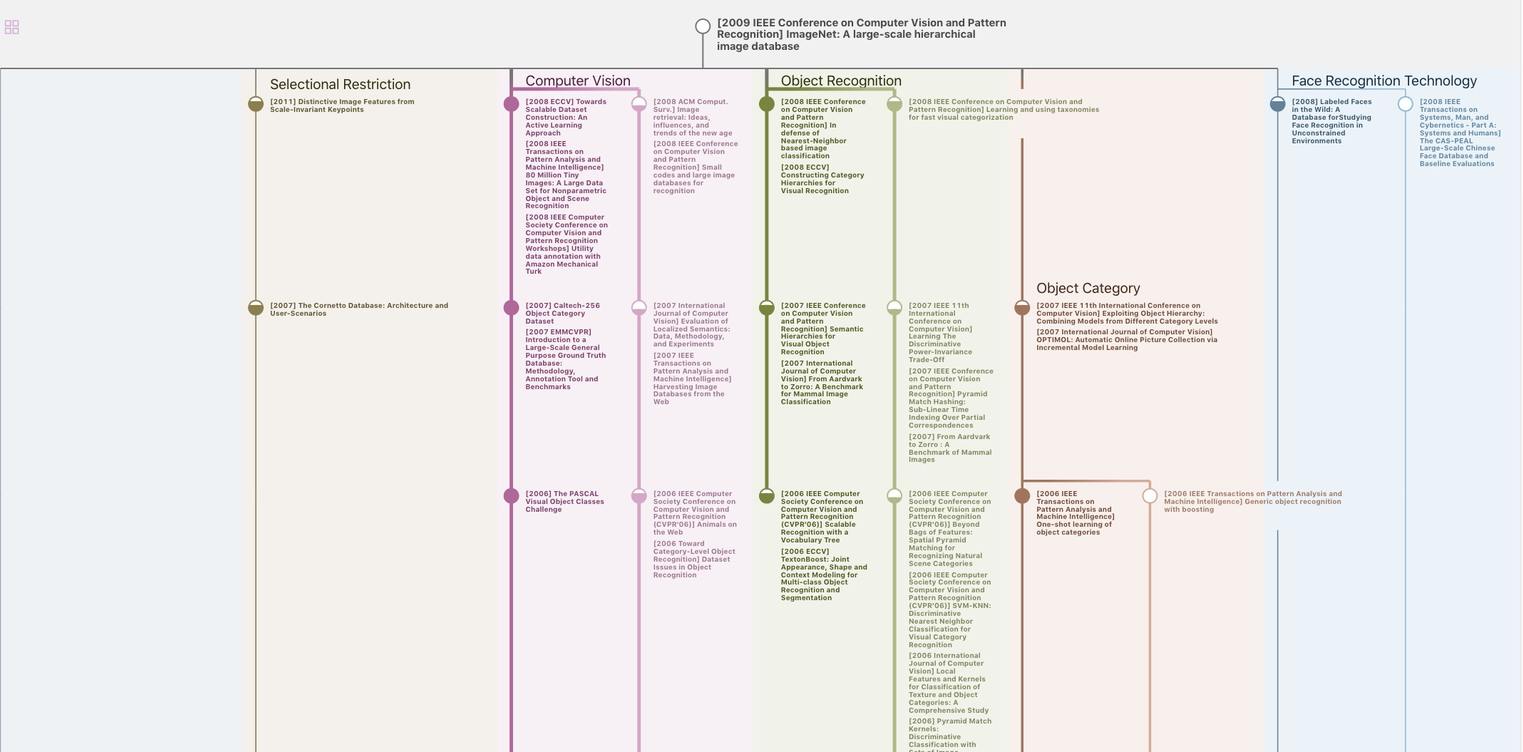
生成溯源树,研究论文发展脉络
Chat Paper
正在生成论文摘要