Robustness Verification of Deep Neural Networks Using Star-Based Reachability Analysis with Variable-Length Time Series Input
FORMAL METHODS FOR INDUSTRIAL CRITICAL SYSTEMS, FMICS 2023(2023)
摘要
Data-driven, neural network (NN) based anomaly detection and predictive maintenance are emerging as important research areas. NN-based analytics of time-series data provide valuable insights and statistical evidence for diagnosing past behaviors and predicting critical parameters like equipment's remaining useful life (RUL), state-of-charge (SOC) of batteries, etc. Unfortunately, input time series data can be exposed to intentional or unintentional noise when passing through sensors, making robust validation and verification of these NNs a crucial task. Using set-based reachability analysis, this paper presents a case study of the formal robustness verification approach for time series regression NNs (TSRegNN). It utilizes variable-length input data to streamline input manipulation and enhance network architecture generalizability. The method is applied to two data sets in the Prognostics and Health Management (PHM) application areas: (1) SOC estimation of a Lithium-ion battery and (2) RUL estimation of a turbine engine. Finally, the paper introduces several performance measures to evaluate the effect of bounded perturbations in the input on network outputs, i.e., future outcomes. Overall, the paper offers a comprehensive case study for validating and verifying NN-based analytics of time-series data in real-world applications, emphasizing the importance of robustness testing for accurate and reliable predictions, especially considering the impact of noise on future outcomes.
更多查看译文
关键词
Predictive Maintenance,Time Series Data,Neural Network Verification,Star-set,Reachability Analysis,Noise,Robustness Verification,Prognostics and Health Management
AI 理解论文
溯源树
样例
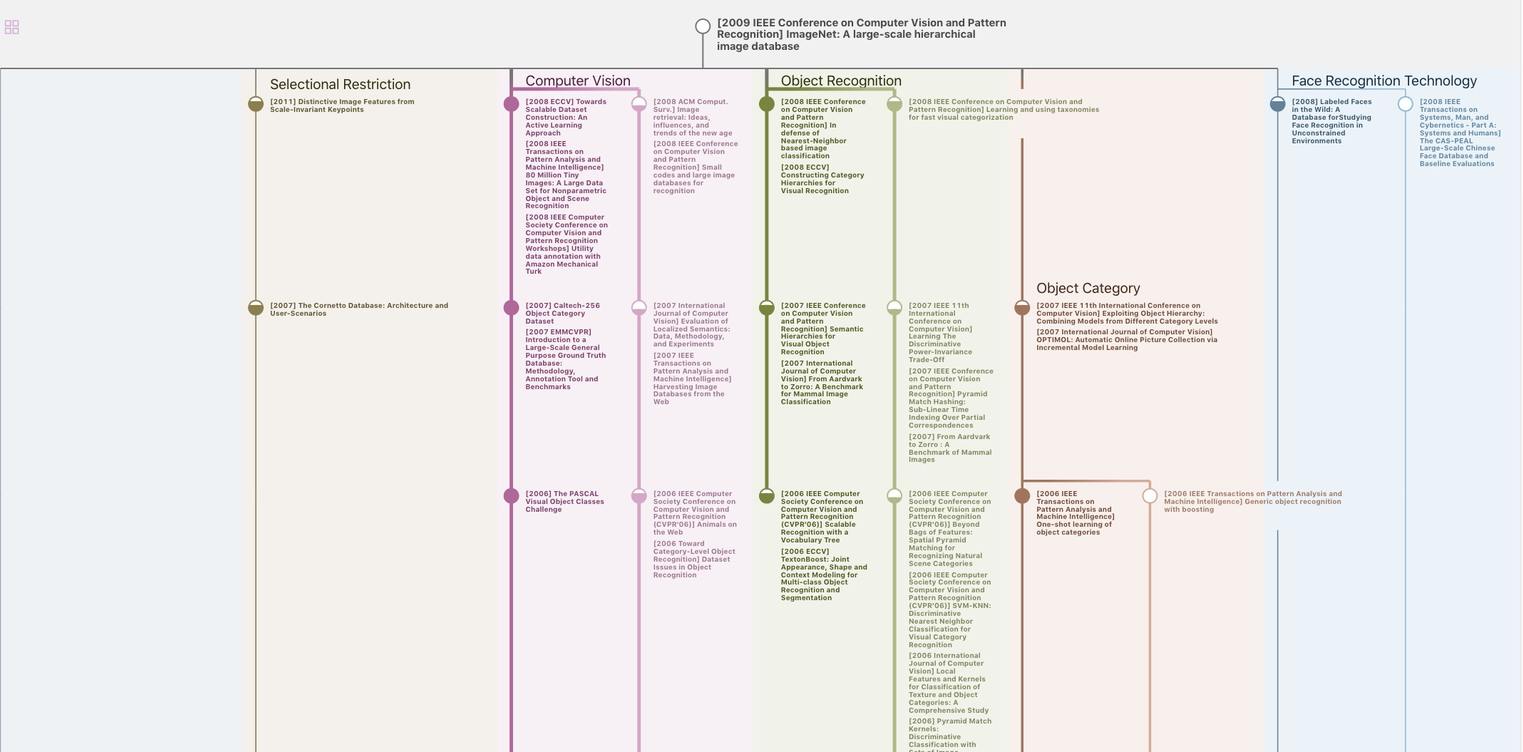
生成溯源树,研究论文发展脉络
Chat Paper
正在生成论文摘要