Spectral-DP: Differentially Private Deep Learning through Spectral Perturbation and Filtering
SP(2023)
摘要
Differential privacy is a widely accepted measure of privacy in the context of deep learning algorithms, and achieving it relies on a noisy training approach known as differentially private stochastic gradient descent (DP-SGD). DP-SGD requires direct noise addition to every gradient in a dense neural network, the privacy is achieved at a significant utility cost. In this work, we present Spectral-DP, a new differentially private learning approach which combines gradient perturbation in the spectral domain with spectral filtering to achieve a desired privacy guarantee with a lower noise scale and thus better utility. We develop differentially private deep learning methods based on Spectral-DP for architectures that contain both convolution and fully connected layers. In particular, for fully connected layers, we combine a block-circulant based spatial restructuring with Spectral-DP to achieve better utility. Through comprehensive experiments, we study and provide guidelines to implement Spectral-DP deep learning on benchmark datasets. In comparison with state-of-the-art DP-SGD based approaches, Spectral-DP is shown to have uniformly better utility performance in both training from scratch and transfer learning settings.
更多查看译文
关键词
private deep learning,deep learning,perturbation
AI 理解论文
溯源树
样例
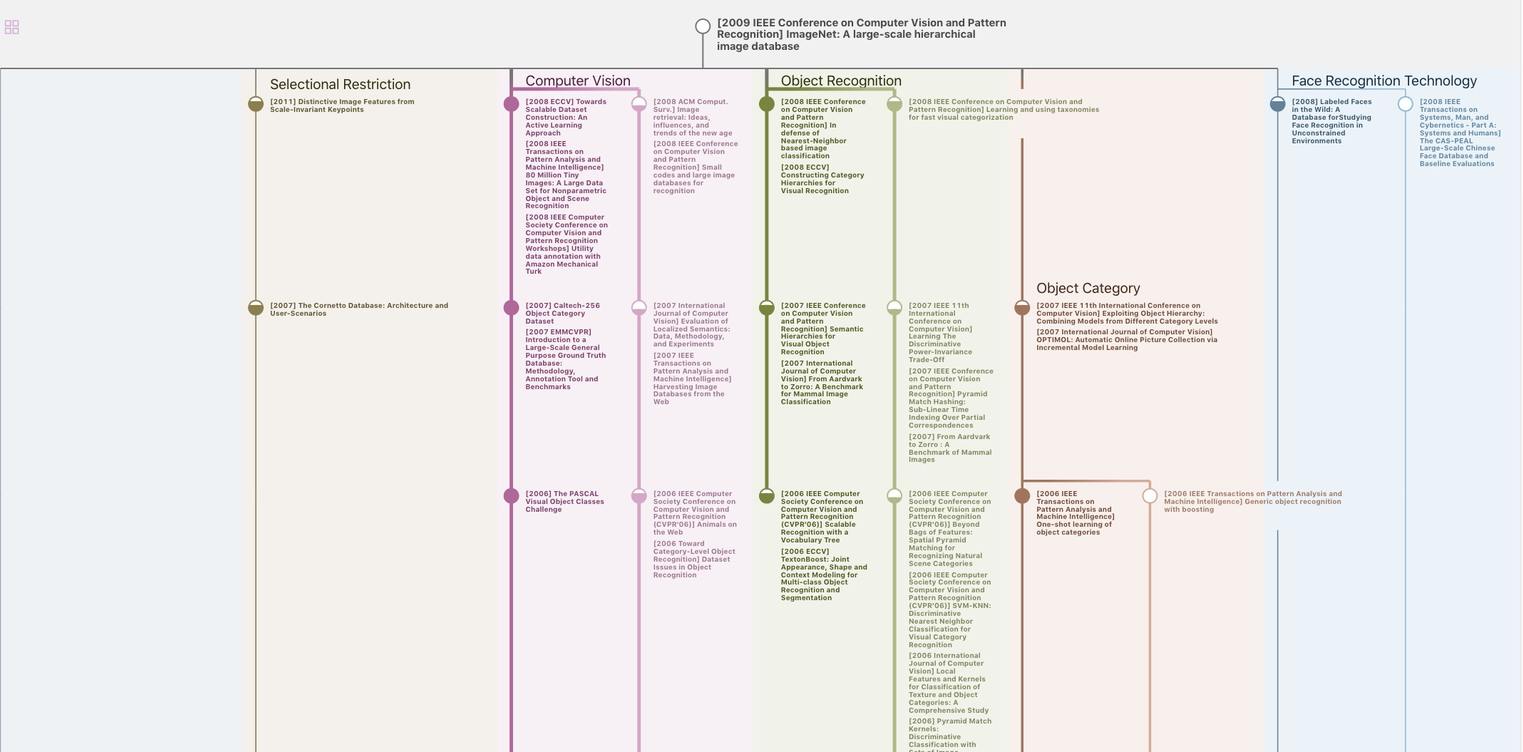
生成溯源树,研究论文发展脉络
Chat Paper
正在生成论文摘要