Multiscale Video Pretraining for Long-Term Activity Forecasting
CoRR(2023)
摘要
Long-term activity forecasting is an especially challenging research problem because it requires understanding the temporal relationships between observed actions, as well as the variability and complexity of human activities. Despite relying on strong supervision via expensive human annotations, state-of-the-art forecasting approaches often generalize poorly to unseen data. To alleviate this issue, we propose Multiscale Video Pretraining (MVP), a novel self-supervised pretraining approach that learns robust representations for forecasting by learning to predict contextualized representations of future video clips over multiple timescales. MVP is based on our observation that actions in videos have a multiscale nature, where atomic actions typically occur at a short timescale and more complex actions may span longer timescales. We compare MVP to state-of-the-art self-supervised video learning approaches on downstream long-term forecasting tasks including long-term action anticipation and video summary prediction. Our comprehensive experiments across the Ego4D and Epic-Kitchens-55/100 datasets demonstrate that MVP out-performs state-of-the-art methods by significant margins. Notably, MVP obtains a relative performance gain of over 20% accuracy in video summary forecasting over existing methods.
更多查看译文
关键词
activity,video,long-term
AI 理解论文
溯源树
样例
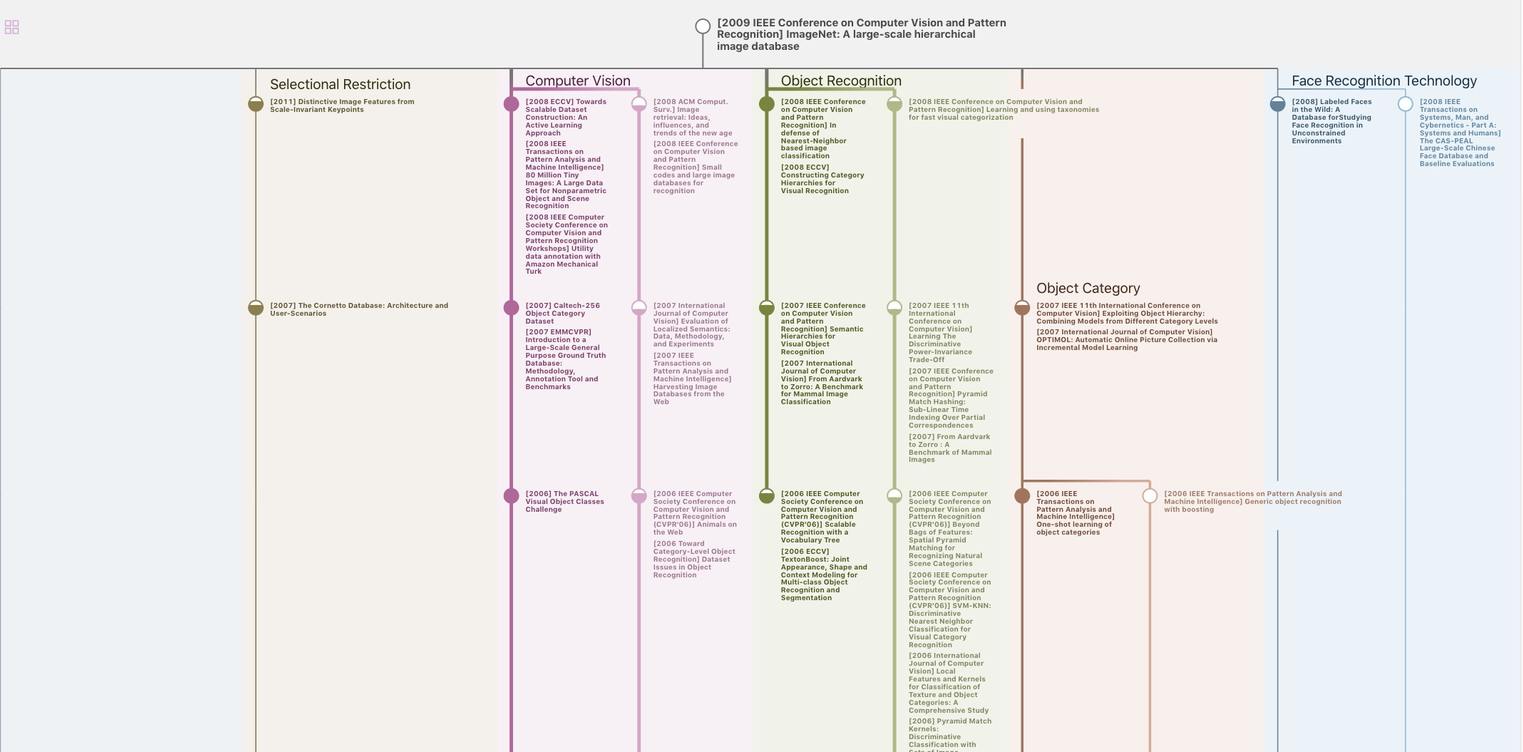
生成溯源树,研究论文发展脉络
Chat Paper
正在生成论文摘要