Is Attention all You Need in Medical Image Analysis? A Review.
IEEE journal of biomedical and health informatics(2024)
摘要
Medical imaging is a key component in clinical diagnosis, treatment planning and clinical trial design, accounting for almost 90% of all healthcare data. CNNs achieved performance gains in medical image analysis (MIA) over the last years. CNNs can efficiently model local pixel interactions and be trained on small-scale MI data. Despite their important advances, typical CNN have relatively limited capabilities in modelling "global" pixel interactions, which restricts their generalisation ability to understand out-of-distribution data with different "global" information. The recent progress of Artificial Intelligence gave rise to Transformers, which can learn global relationships from data. However, full Transformer models need to be trained on large-scale data and involve tremendous computational complexity. Attention and Transformer compartments ("Transf/Attention") which can well maintain properties for modelling global relationships, have been proposed as lighter alternatives of full Transformers. Recently, there is an increasing trend to co-pollinate complementary local-global properties from CNN and Transf/Attention architectures, which led to a new era of hybrid models. The past years have witnessed substantial growth in hybrid CNN-Transf/Attention models across diverse MIA problems. In this systematic review, we survey existing hybrid CNN-Transf/Attention models, review and unravel key architectural designs, analyse breakthroughs, and evaluate current and future opportunities as well as challenges. We also introduced an analysis framework on generalisation opportunities of scientific and clinical impact, based on which new data-driven domain generalisation and adaptation methods can be stimulated.
更多查看译文
关键词
Attention,Computed tomography,Convolutional neural networks,Magnetic resonance imaging,Medical image analysis,Positron emission tomography,Retinal imaging,Transformers
AI 理解论文
溯源树
样例
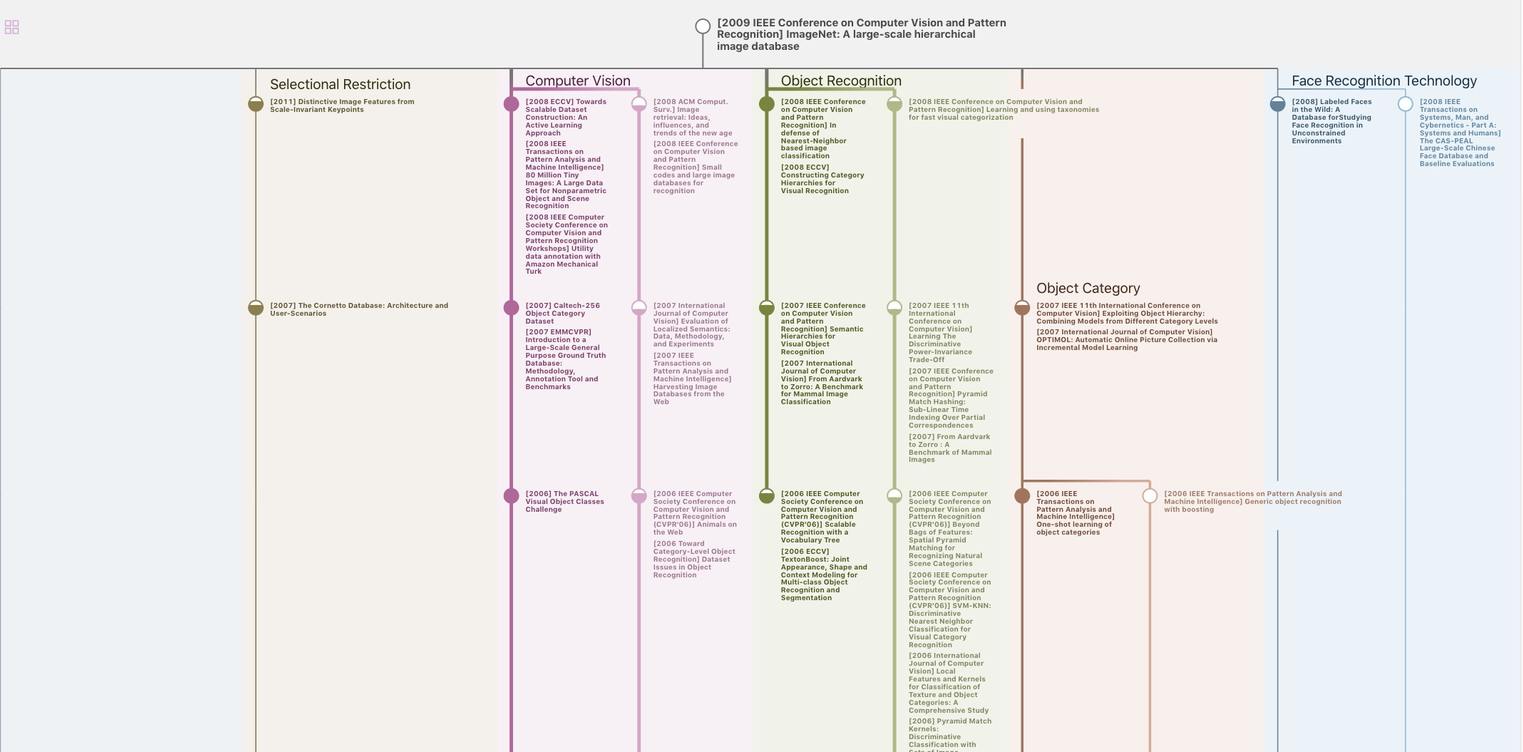
生成溯源树,研究论文发展脉络
Chat Paper
正在生成论文摘要