Multifidelity Covariance Estimation via Regression on the Manifold of Symmetric Positive Definite Matrices
CoRR(2023)
摘要
We introduce a multifidelity estimator of covariance matrices formulated as the solution to a regression problem on the manifold of symmetric positive definite matrices. The estimator is positive definite by construction, and the Mahalanobis distance minimized to obtain it possesses properties which enable practical computation. We show that our manifold regression multifidelity (MRMF) covariance estimator is a maximum likelihood estimator under a certain error model on manifold tangent space. More broadly, we show that our Riemannian regression framework encompasses existing multifidelity covariance estimators constructed from control variates. We demonstrate via numerical examples that our estimator can provide significant decreases, up to one order of magnitude, in squared estimation error relative to both single-fidelity and other multifidelity covariance estimators. Furthermore, preservation of positive definiteness ensures that our estimator is compatible with downstream tasks, such as data assimilation and metric learning, in which this property is essential.
更多查看译文
关键词
symmetric positive definite matrices,manifold,regression,estimation
AI 理解论文
溯源树
样例
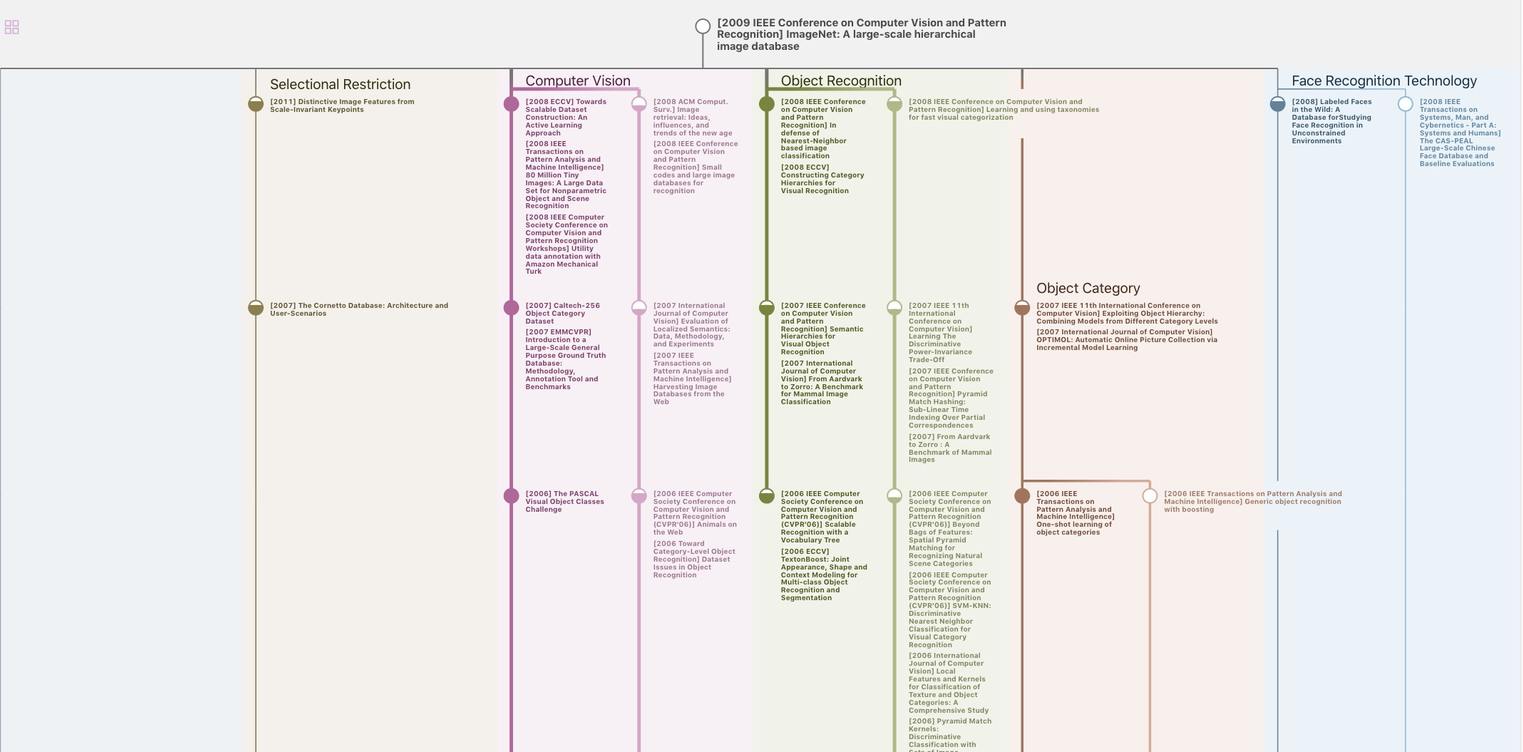
生成溯源树,研究论文发展脉络
Chat Paper
正在生成论文摘要