Is Learning Summary Statistics Necessary for Likelihood-free Inference?
ICML 2023(2023)
摘要
Likelihood-free inference (LFI) is a set of techniques for inference in implicit statistical models. A longstanding question in LFI has been how to design or learn good summary statistics of data, but this might now seem unnecessary due to the advent of recent end-to-end (i.e. neural network-based) LFI methods. In this work, we rethink this question with a new method for learning summary statistics. We show that learning sufficient statistics may be easier than direct posterior inference, as the former problem can be reduced to a set of low-dimensional, easy-to-solve learning problems. This suggests us to explicitly decouple summary statistics learning from posterior inference in LFI. Experiments on diverse inference tasks with different data types validate our hypothesis.
更多查看译文
AI 理解论文
溯源树
样例
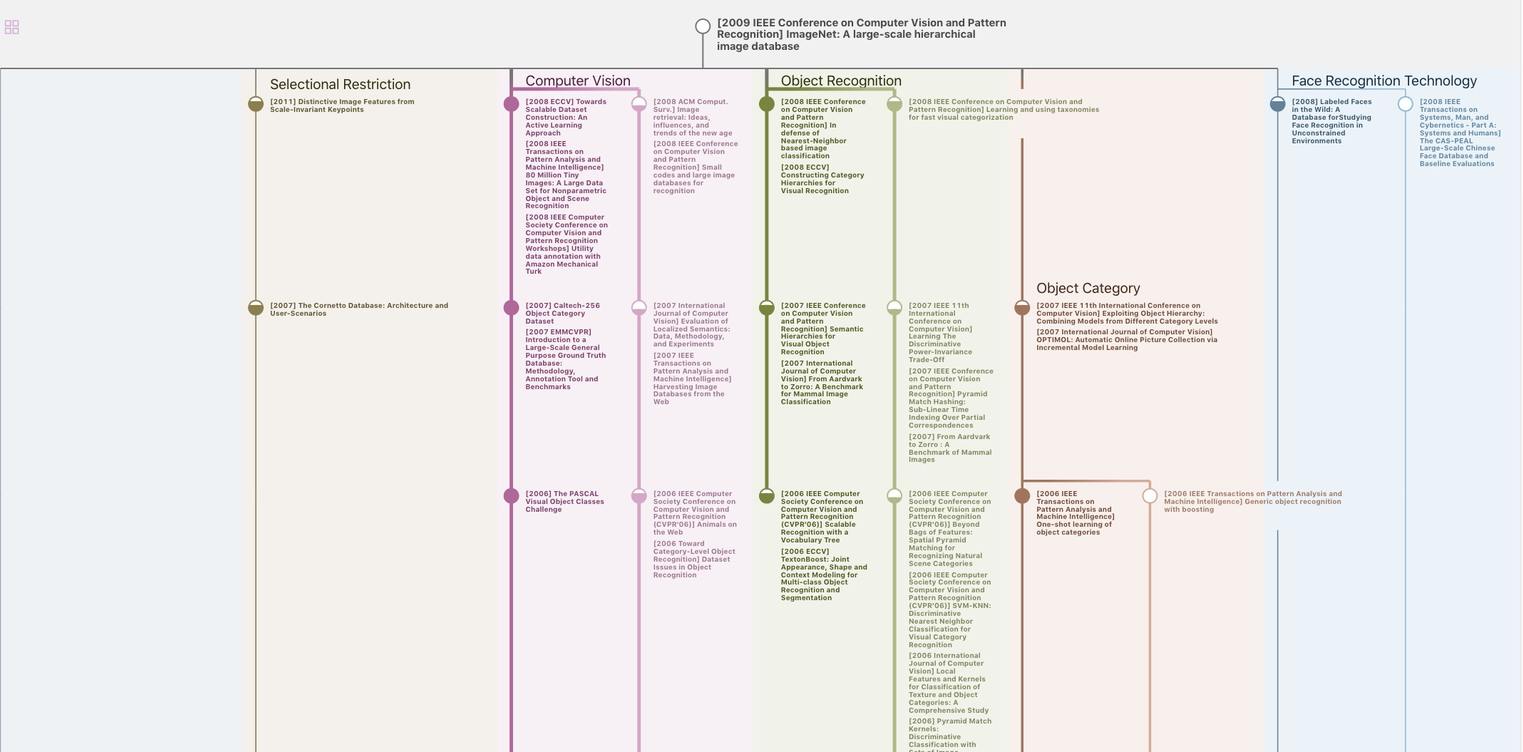
生成溯源树,研究论文发展脉络
Chat Paper
正在生成论文摘要