Optimal Online Generalized Linear Regression with Stochastic Noise and Its Application to Heteroscedastic Bandits
ICML 2023(2023)
摘要
We study the problem of online generalized linear regression in the stochastic setting, where the label is generated from a generalized linear model with possibly unbounded additive noise. We provide a sharp analysis of the classical *follow-the-regularized-leader* (FTRL) algorithm to cope with the label noise. More specifically, for $\sigma$-sub-Gaussian label noise, our analysis provides a regret upper bound of $O(\sigma^2 d \log T) + o(\log T)$, where $d$ is the dimension of the input vector, $T$ is the total number of rounds. We also prove an $\Omega(\sigma^2d\log(T/d))$ lower bound for stochastic online linear regression, which indicates that our upper bound is nearly optimal. In addition, we extend our analysis to a more refined Bernstein noise condition. As an application, we study generalized linear bandits with heterogeneous noise and propose an algorithm based on FTRL to achieve the first variance-aware regret bound.
更多查看译文
关键词
stochastic noise,linear regression,online
AI 理解论文
溯源树
样例
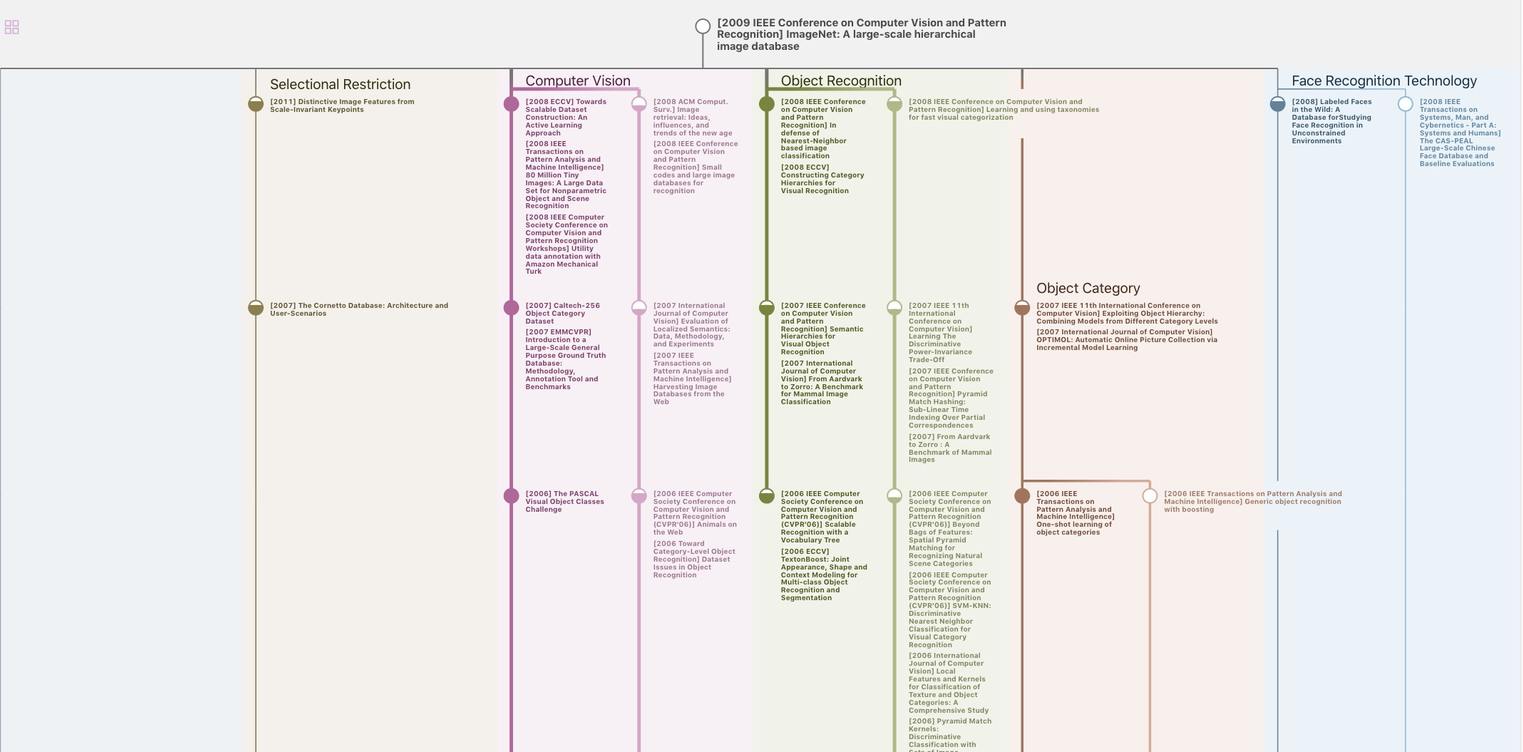
生成溯源树,研究论文发展脉络
Chat Paper
正在生成论文摘要