Benign Overfitting in Two-layer ReLU Convolutional Neural Networks
ICML 2023(2023)
摘要
Modern deep learning models with great expressive power can be trained to overfit the training data but still generalize well. This phenomenon is referred to as benign overfitting. Recently, a few studies have attempted to theoretically understand benign overfitting in neural networks. However, these works are either limited to neural networks with smooth activation functions or to the neural tangent kernel regime. How and when benign overfitting can occur in ReLU neural networks remains an open problem. In this work, we seek to answer this question by establishing algorithm-dependent risk bounds for learning two-layer ReLU convolutional neural networks with label-flipping noise. We show that, under mild conditions, the neural network trained by gradient descent can achieve near-zero training loss and Bayes optimal test risk. Our result also reveals a sharp transition between benign and harmful overfitting under different conditions on data distribution in terms of test risk. Experiments on synthetic data back up our theory.
更多查看译文
关键词
networks,two-layer
AI 理解论文
溯源树
样例
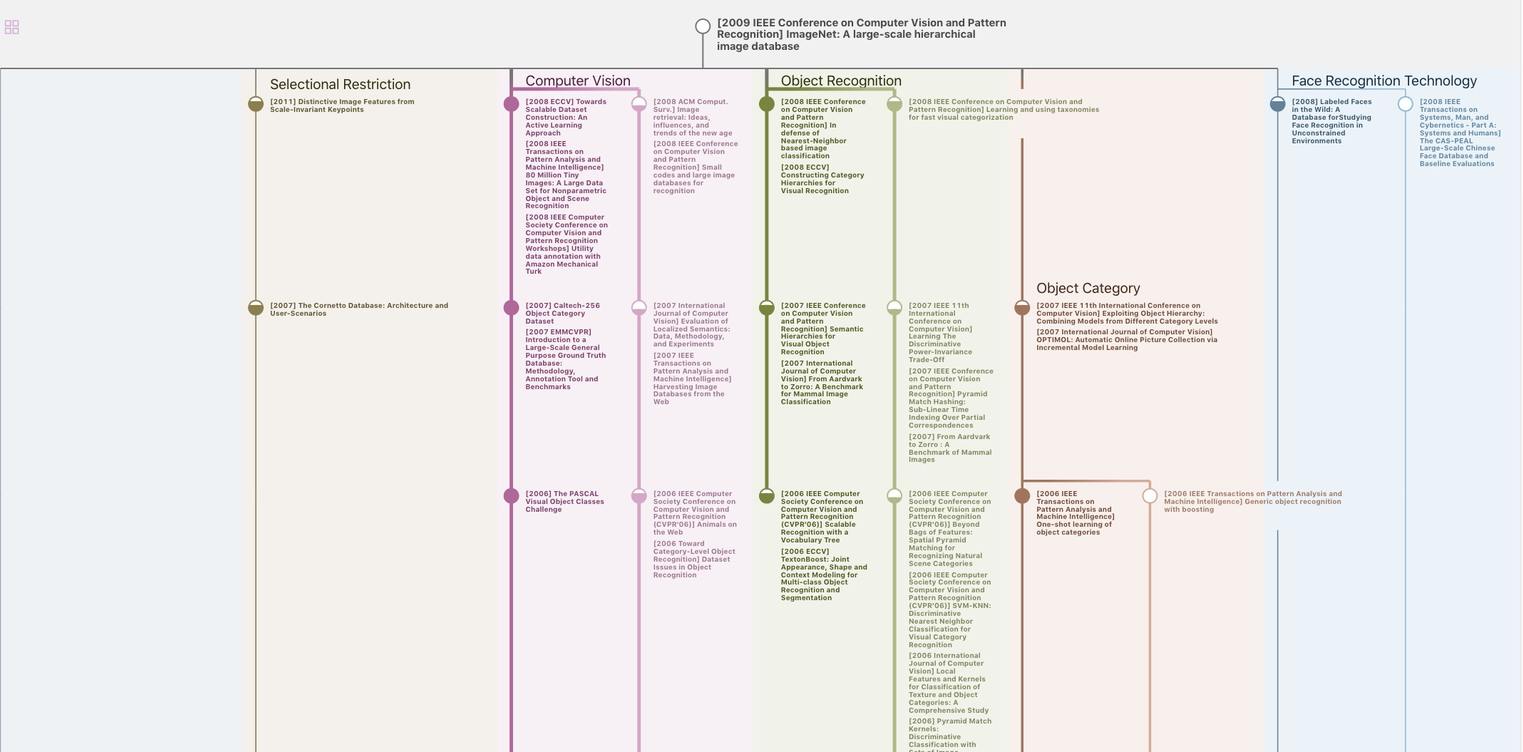
生成溯源树,研究论文发展脉络
Chat Paper
正在生成论文摘要