Minibatching Offers Improved Generalization Performance for Second Order Optimizers
CoRR(2023)
摘要
Training deep neural networks (DNNs) used in modern machine learning is computationally expensive. Machine learning scientists, therefore, rely on stochastic first-order methods for training, coupled with significant hand-tuning, to obtain good performance. To better understand performance variability of different stochastic algorithms, including second-order methods, we conduct an empirical study that treats performance as a response variable across multiple training sessions of the same model. Using 2-factor Analysis of Variance (ANOVA) with interactions, we show that batch size used during training has a statistically significant effect on the peak accuracy of the methods, and that full batch largely performed the worst. In addition, we found that second-order optimizers (SOOs) generally exhibited significantly lower variance at specific batch sizes, suggesting they may require less hyperparameter tuning, leading to a reduced overall time to solution for model training.
更多查看译文
关键词
improved generalization performance,second order
AI 理解论文
溯源树
样例
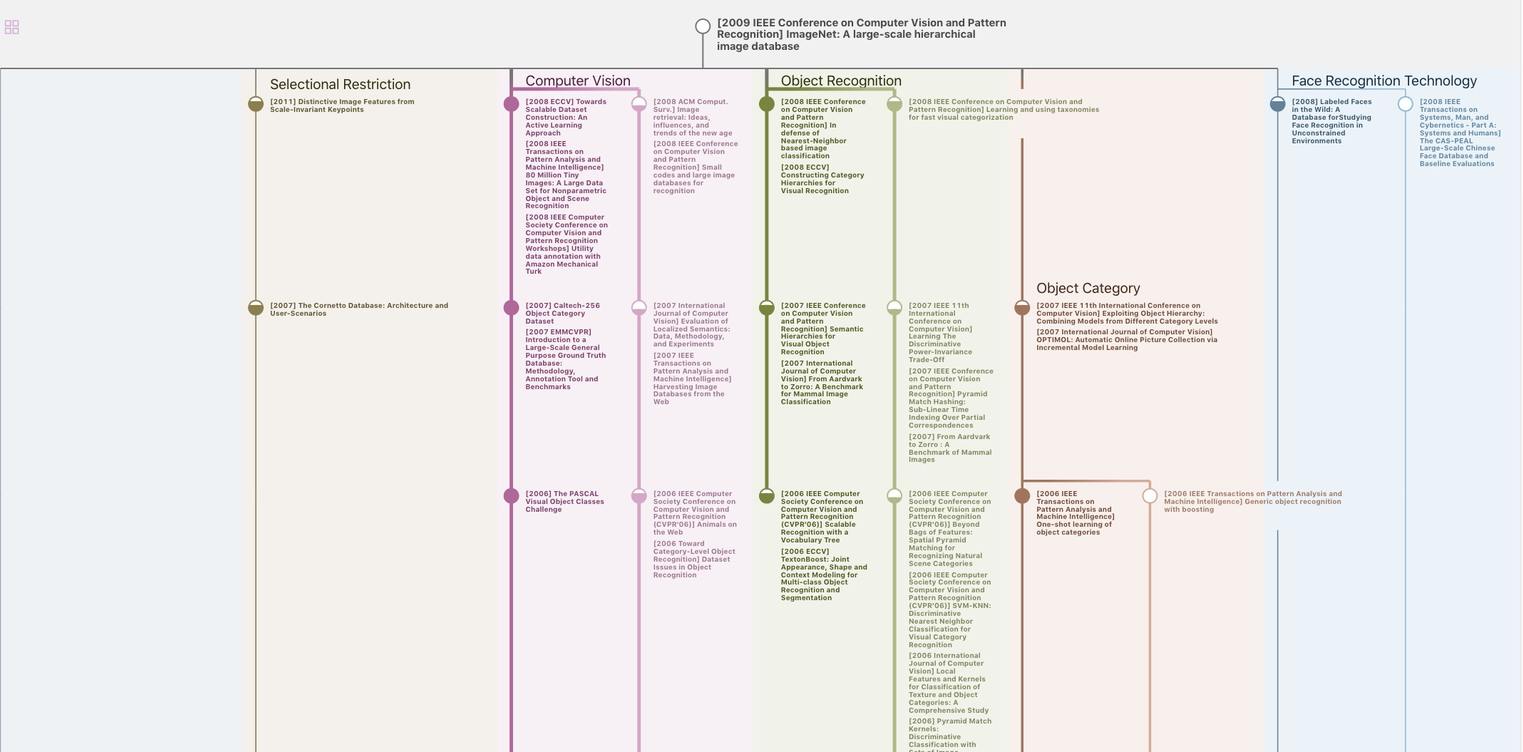
生成溯源树,研究论文发展脉络
Chat Paper
正在生成论文摘要