MatSpectNet: Material Segmentation Network with Domain-Aware and Physically-Constrained Hyperspectral Reconstruction
CoRR(2023)
摘要
Achieving accurate material segmentation for 3-channel RGB images is challenging due to the considerable variation in a material's appearance. Hyperspectral images, which are sets of spectral measurements sampled at multiple wavelengths, theoretically offer distinct information for material identification, as variations in intensity of electromagnetic radiation reflected by a surface depend on the material composition of a scene. However, existing hyperspectral datasets are impoverished regarding the number of images and material categories for the dense material segmentation task, and collecting and annotating hyperspectral images with a spectral camera is prohibitively expensive. To address this, we propose a new model, the MatSpectNet to segment materials with recovered hyperspectral images from RGB images. The network leverages the principles of colour perception in modern cameras to constrain the reconstructed hyperspectral images and employs the domain adaptation method to generalise the hyperspectral reconstruction capability from a spectral recovery dataset to material segmentation datasets. The reconstructed hyperspectral images are further filtered using learned response curves and enhanced with human perception. The performance of MatSpectNet is evaluated on the LMD dataset as well as the OpenSurfaces dataset. Our experiments demonstrate that MatSpectNet attains a 1.60% increase in average pixel accuracy and a 3.42% improvement in mean class accuracy compared with the most recent publication. The project code is attached to the supplementary material and will be published on GitHub.
更多查看译文
关键词
material segmentation network,hyperspectral reconstruction,domain-aware,physically-constrained
AI 理解论文
溯源树
样例
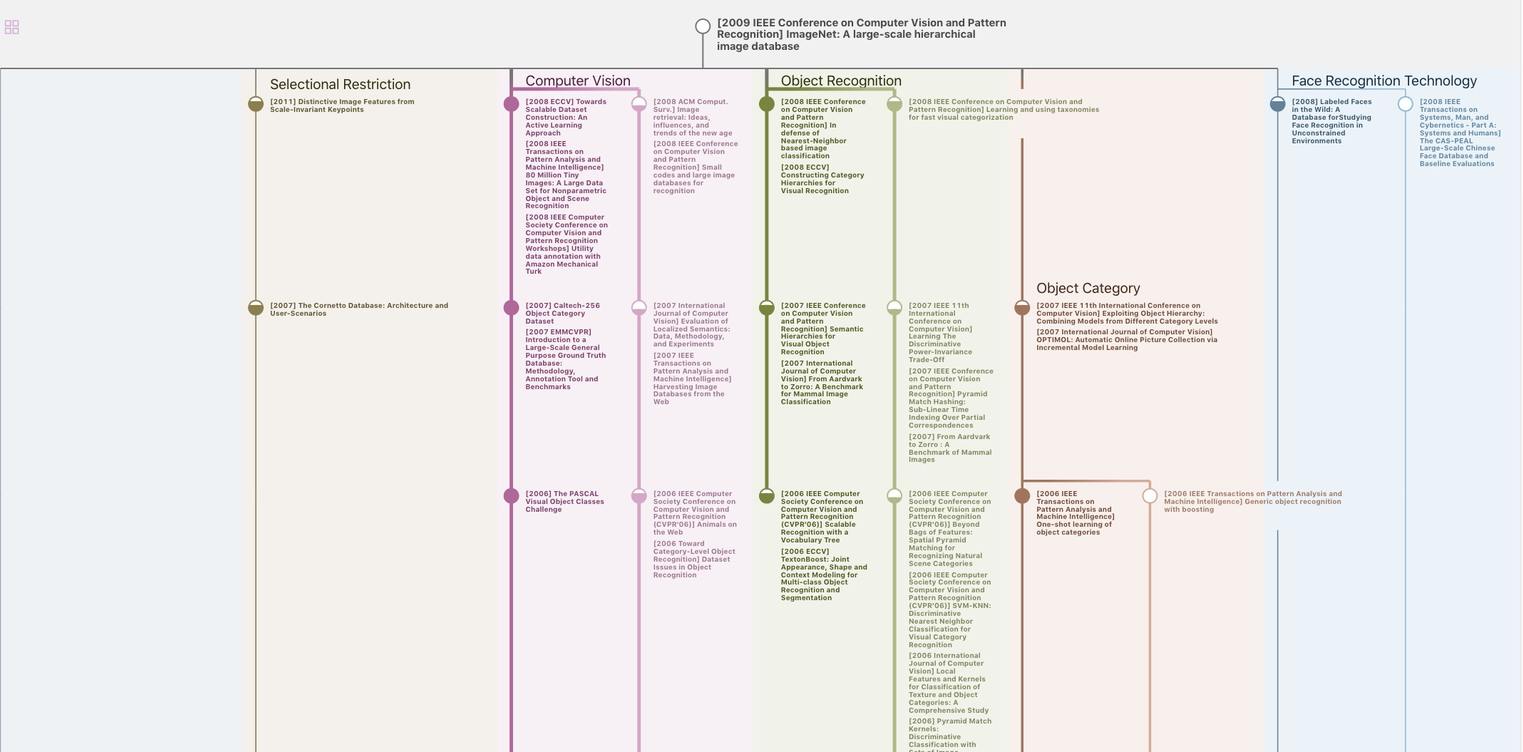
生成溯源树,研究论文发展脉络
Chat Paper
正在生成论文摘要